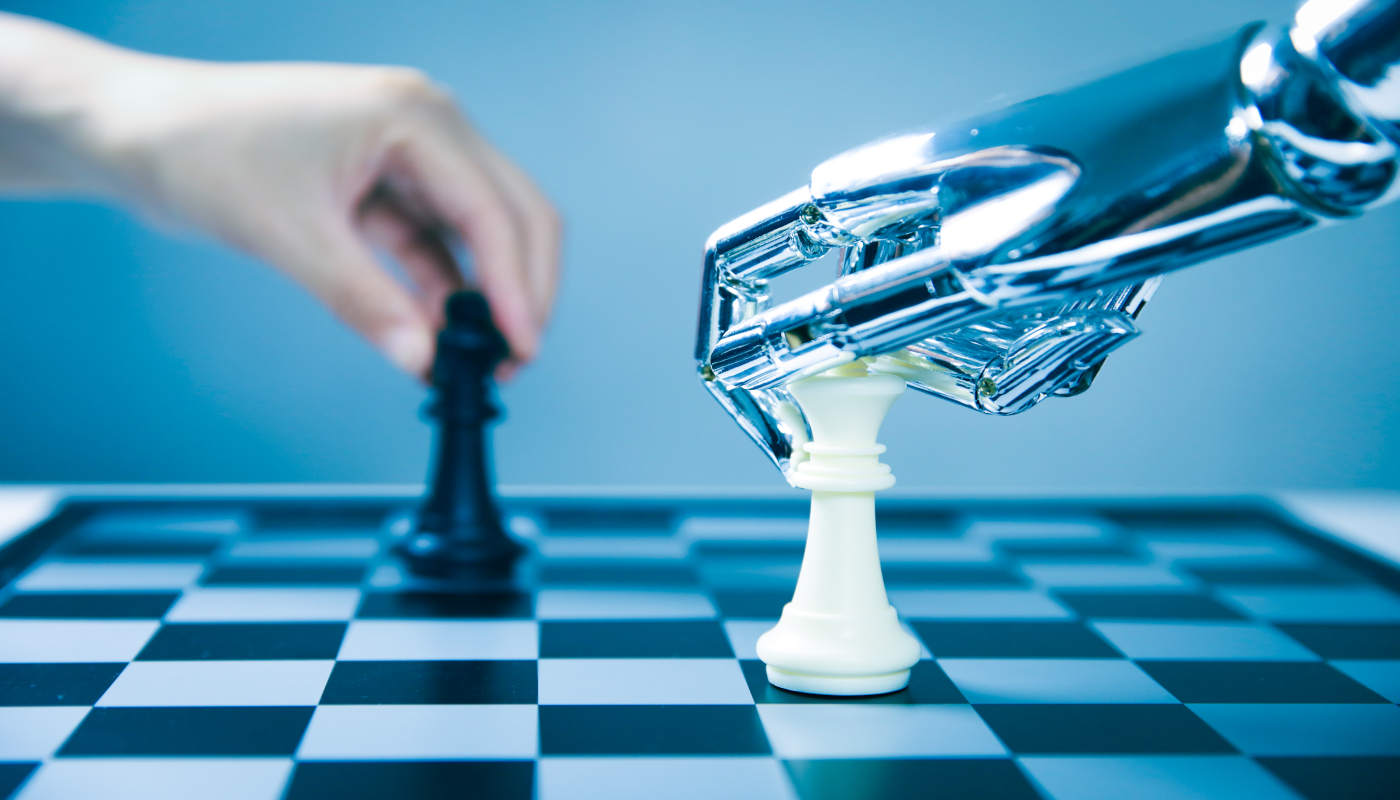
The most endearing sketches of Little Britain, a BBC comedy show, features a character named Carol Beer. Carol always responds to a customer's enquiry by typing into her computer and responding, even to the most reasonable of requests, with a monotone, "Computer says no." She exemplifies the downside of over-reliance on computer-based decision making.
As the financial industry rapidly adapted to the COVID-19 pandemic, it executed a substantive digital transformation plan in a matter of weeks, gearing up remote operations platforms, apps and devices. In this strategic shift to increased automation and a greater reliance on algorithmic decision making - specifically, artificial intelligence (AI) and machine learning (ML) - too many Carol Beers can be a problem.
To reap the benefits of algorithmic decision-making, a firm must first establish trust in its model across stakeholders: e.g., the front office, risk management, regulators and users. The biases and problems stemming from the black box nature of AI- and ML-driven approaches undermine that trust.
Bias Problems
ML algorithms incorporate, in most cases, inadvertent and subconscious human biases, further amplifying inaccuracies in data collection, processing and engineering. To mitigate the impact of these errors, developers can explore a range of specifications (including the use of different data sets), or benchmark the performance and predicative accuracy of their ML models against existing traditional (non-ML) models.
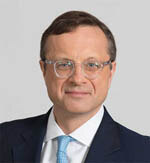
Statistical approaches, including re-sampling, binning, and other techniques, can address biases in the data itself. Moreover, independent validation by subject matter experts can identify possible biases in the data beyond the usual “garbage in, garbage out” problem.
As policy makers, businesses and developers further attempt to improve the fairness of ML models, additional consideration must be given to their complexity (the feedback loop). Small, incremental changes to the data or the parameters of a model can ultimately become problematic. How, where and what data are split, or even integrated at the various stages of model deployment, will impact the final results.
Consequently, simple regulatory, rules-based standards - or broad-based principles - may not effectively address the bias of ML-based models. Developers can, however, help mitigate the potential for unfairness and improve the quality/reliability of decision making by clearly defining and documenting the goal and the purpose of critical algorithms - including how the algorithms were trained.
Algorithms and models, after all, reflect the goals and perspectives of their developers, as well as the data that “trains” them. What works for ML should also work for AI.
The Need for Explainable AI
Another concern is the explainability of models: ML approaches train series of algorithms on large data sets by iteratively optimizing the outcome of newly-identified patterns and correlations. The iterative optimization make these algorithms elaborate, with complex underlying mathematics.
Even some of the simpler models are convoluted: for example, a simple naive neural network used for credit assessment can generate millions of feature combinations. The functioning of a multi-layer Generative Adversarial Network (GAN) takes time to understand, even for experts. There is consequently a need for explainable AI (xAI).
Complicating matters further, the most powerful uses of ML in the financial industry operate in an unstructured - almost autonomous - manner. Sophisticated customer service chatbots, for instance, are "black boxes”: we can observe their inputs (the questions) and outputs (the responses), but the underlying language models (like BERT for natural-language processing NLP) may not explain completely the connecting mechanism. Indeed, beyond very simplistic tasks, the performance of the chatbots is not particularly good, which in turn raises risk management concerns.
Typically, explaining AI- or ML-based models requires additional steps, including algorithms (such as SHAP) to clarify the results. Simpler models are easier to interpret (qualitatively), partly because their predictions do not rely on highly convoluted and interlinked computations. Firms can also reverse engineer models to improve explainability - but this approach is not nearly as useful in more complex models.
Explainable AI: A Risk Management Advantage
Putting explainability and transparency at the core of algorithmic decision making is a key risk management imperative. Making business decisions based on information that is difficult to explain - or on conditions that are not clearly stated - will likely cause concerns. Firms that choose to ignore AI explainability therefore subject themselves to immense strategic risks.
Generally, credit decisions in the U.S. have to be explained in compliance with the Equal Credit Opportunity Act: lenders must provide specific reasons why a negative loan decision was made. The developer of an ML algorithm that more efficiently predicts mortgage loan default may be asked to explain why it priced a loan one way, or why a certain borrower was rejected.
Since there are regulations mandating that a consumer credit risk model is transparent and explainable, it should be easier to develop an AI- or ML-driven model.
Fixed income and FX trading models that intend to minimize costs and speedily execute transactions in a liquid market are considerably more complicated. Everyone who plays a role in an electronic trade (traders, portfolio managers, risk managers, executive management, clients and regulators) needs to understand - at least to some degree - the drivers of these models.
From a regulatory perspective, trading based on sophisticated algorithms is usually treated as a model risk management problem (with limited guidance in SR 11-7), focusing more on the model development process and less on explainability. But focusing on xAI as a model risk management issue misses the real problem.
Parting Thoughts
ML and AI offer operational efficiencies: a superior ability to recognize patterns, extract signals and compute correlations across vast volumes of data - at a fraction of the cost of an army of humans. But for AI and ML models to be widely integrated in any (semi‐) autonomous decision-making capacity, they must be explainable, accommodating some degree of human interaction and feedback.
Regardless of whether we're talking about Carol Beer or an actual credit risk analyst, the humans making the decisions must have as much input as the humans who created and validated the algorithms.
Peter Went is a lecturer at Columbia University, where he teaches about disruptive technologies like artificial intelligence and machine learning, and their impact on risk management.