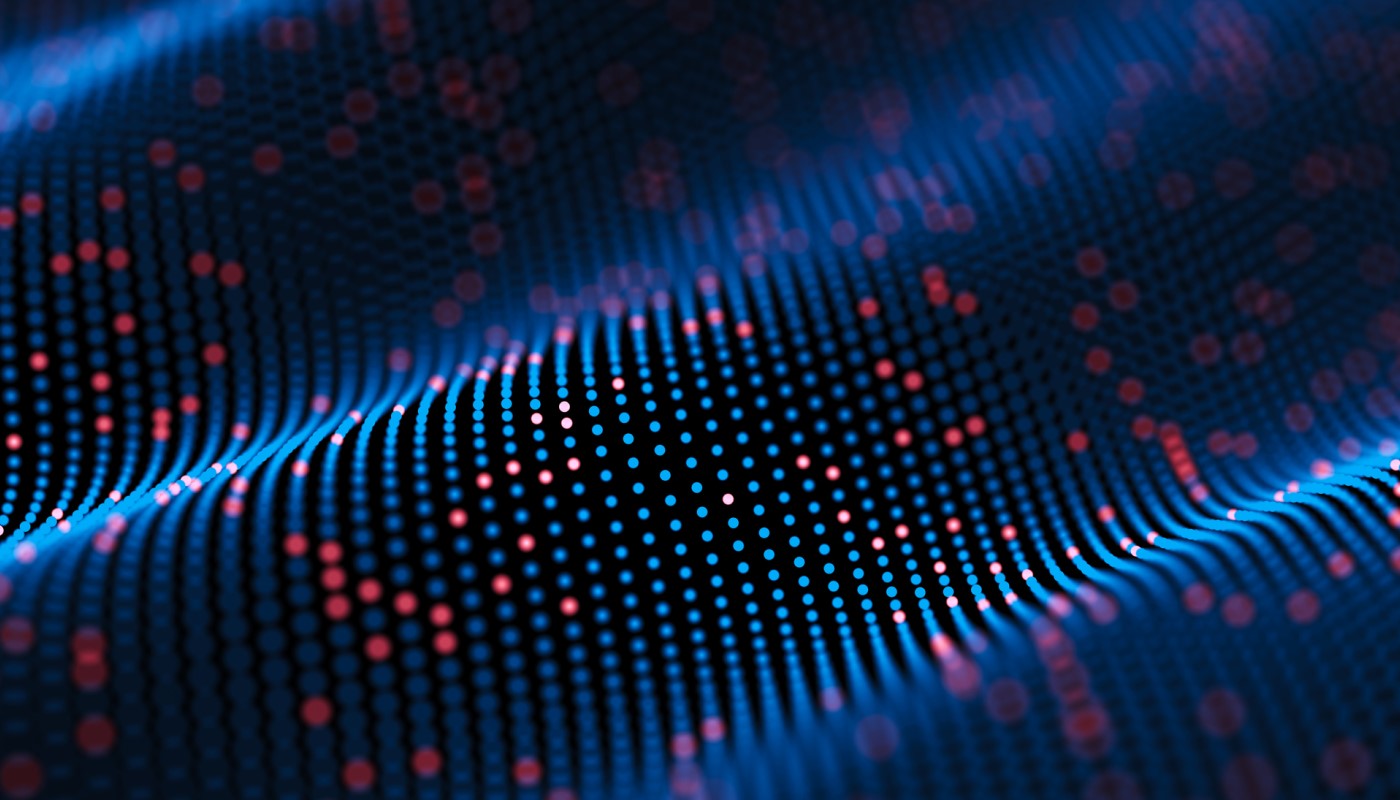
The financial services industry is becoming rapidly transformed by the shift toward online banking products and services, where models driven by artificial intelligence (AI) have risen to prominence. Financial technology companies (fintechs) are playing a pivotal role in the so-called banking digitization trend, but now face their own set of challenges based on their heavy use of largely autonomous algorithmic models.
These models generate decisions that have grown increasingly separate from direct and immediate human monitoring, supervision and oversight. But fintechs must be careful: algorithmic failures expose them to various hard-to-manage, headline-grabbing reputational and legal risks that often prove quite expensive.
Indeed, as highlighted by the growing list of AI-decision incidents, the main hazard facing fintechs is not strategic and business risk but, rather, algorithmic risk. To reduce algorithmic risk, fintechs should follow risk management guidelines and build their frameworks around transparency, explainability, safety and accountability.
Let's now take a closer look at each of these principles.
Algorithms Must be Transparent
The purpose of algorithmic transparency is two-fold. One is connected to regulation: there are already legislative mandates in the U.S. for businesses to explain the considerations behind adverse decisions that impact retail customers.
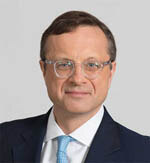
Since algorithms evaluate sensitive information, it's important for fintechs to make it clear that technology - rather than humans - is the driving force behind all decisions (including adverse choices) made by their algorithmic models.
Beyond enabling fintechs to meet regulatory requirements, transparency can not only increase trust in the inner workings of an AI system but also potentially enhance its accuracy, reliability and overall performance. Through offering information on the development, training, operation and deployment of an algorithmic model, fintech model developers can provide clarity and precision to management and regulators about data, inputs and decisions.
Management, regulators and clients need to understand how algorithmic decisions are made and how the various factors impacting the decisions influence both each other and the final outcome. Transparency can help highlight how an algorithm derives decisions based on data from similar or dissimilar cohorts. To gain trust and confidence from a risk management perspective, it is important to make the models as transparent as possible, while also keeping their sensitive inner workings privileged.
A final and important point is to distinguish transparency from explainability. Transparency depicts the working mechanics of the model, as well as the factors that influence its actions and decisions. It does not explain individual performance, but, rather, focuses on the overall functioning of the model.
Algorithms Must be Explainable
Algorithmic discrimination can cause significant reputational and economic damage. In the context of risk management, explainability addresses the adverse or discriminatory impact from algorithmic decision-making.
If a model is not sufficiently explainable, it can create hard-to-understand risks. To mitigate these risks, fintechs should not only adhere to process-documentation standards but also delineate and embrace principled ethical standards for organizational AI usage.
One of many approaches to manage risk from algorithmic decision-making is to document the development of algorithmic processes with clarity and consistency. This will build trust with senior management, providing them with sufficient knowledge to explain the considerations underlying algorithmic decisions to clients and, if necessary, regulators.
To understand the impact of any algorithmic decision, from a modeling perspective, one must start by quantifying the marginal impact each factor has on the decision. This can be achieved through a variety of metrics - like the standardized mean difference and the Shapley Value - that capture the interdependence of factors that may influence the outcome of an algorithmic decision.
These metrics and their interpretation can, moreover, help organizations identify biases in their algorithmic decision-making. Remediation to address biases should also be documented, using the same stringency and consistency applied to model development.
The reasoning for these actions is simple: eliminating or at least mitigating the biases introduced by either the data or the algorithms themselves will improve model performance. Ultimately, the more reliable and less biased the decisions become, the less likely a fintech will be to make adversely discriminatory decisions that are exposed to legal risks.
Algorithms Must be Kept Safe
The SolarWinds hack is undoubtedly one of the largest cyberattacks perpetuated on the U.S. government. We may never know exactly how disruptive it was to financial institutions, but it carries important risk management lessons for fintechs.
Apart from needing to adhere to the same security standards for their products as regulated financial services companies, fintechs face two concerns unique to algorithmic decision making.
First, the cost of developing any AI-driven product is significant: commercial espionage - such as stealing ideas, codes and algorithms on nascent AI projects - is believed to be rampant. Successful attacks can wipe out years of hard work and capital investments in new fintech products and services.
Second, the cost of having automated (algorithmic) decisions hijacked, or otherwise influenced, is significant. Successful AI projects may attract attackers who seek either to manipulate the decision-making process by changing the algorithms or by loading the system with data that may corrupt the decisions made by the system. This can be particularly problematic for overly complex black-box algorithms, where even small changes to the data or modeling assumptions can skew the results.
Fortunately, criminal activities like adversarial manipulation of AI-based decisions and corruption of sensitive data are not yet common. But the trajectory is clear: data security for AI products needs to go beyond the currently established standards.
Risk managers need to include cybersecurity and protection in their overall assessment. More specifically, they must consider risk mitigation tools such as robust ML, differential privacy and federated learning.
Comprehensive risk management is the ultimate goal, but cannot be achieved without adequate documentation and clearly outlined responsibilities for managing security breaches.
Algorithms Must be Held Accountable
Many fintech products and services incorporate algorithmic decisions that materially impact external users or clients. In developing and commercializing such products, developers should ensure that decisions have some level of human monitoring, oversight and authorization.
For fintech products and services that integrate predictive or analytical capabilities, such oversight should focus on the data that feeds into the model and the consistent performance of the model. Models that are fed inaccurate or untimely data will inevitably suffer from incorrect forecasts and poor decisions. Maintaining the integrity and quality of the data is a critical data engineering problem and its importance in reducing avoidable errors cannot be understated.
What's more, for a model to operate as originally intended, its performance needs to be evaluated over time and compared to acceptable performance benchmarks. This is particularly important for those self-learning models where decisions and outcomes are interdependent on the quality of the data and the baseline algorithms.
Imposing certain limits on the use of automated decision-making on specific business decisions is an approach many fintechs follow. A variation of the limited use of automated decisions is to subject some, if not all, algorithmic decisions for human approval before the decision is released to the public. This approach can be particularly advantageous when an explanation is required for an adverse algorithmic decision about an external party.
Looking Ahead
Governments around the world continue to explore the long-term policy implications of increased algorithmic decision-making. In the absence of common international and national guidelines, many organizations have developed their own industry-specific algorithmic standards.
Algorithmic accountability, moreover, is one of the many components in the politics of how governments should be regulating both fintech and Big Tech companies. In anticipation of yet-to-be developed standards for algorithmic accountability, fintechs should proactively align their product development efforts.
Over the long run, preemptively considering the impact of potential risks will create a competitive advantage for fintechs, which must protect the substantive investments they have made in their products.
Peter Went (PhD, CFA) lectures at Columbia University on disruptive technologies, such as artificial intelligence and machine learning and their impact on financial services and financial risk management.
Article Updated: May 23, 2024