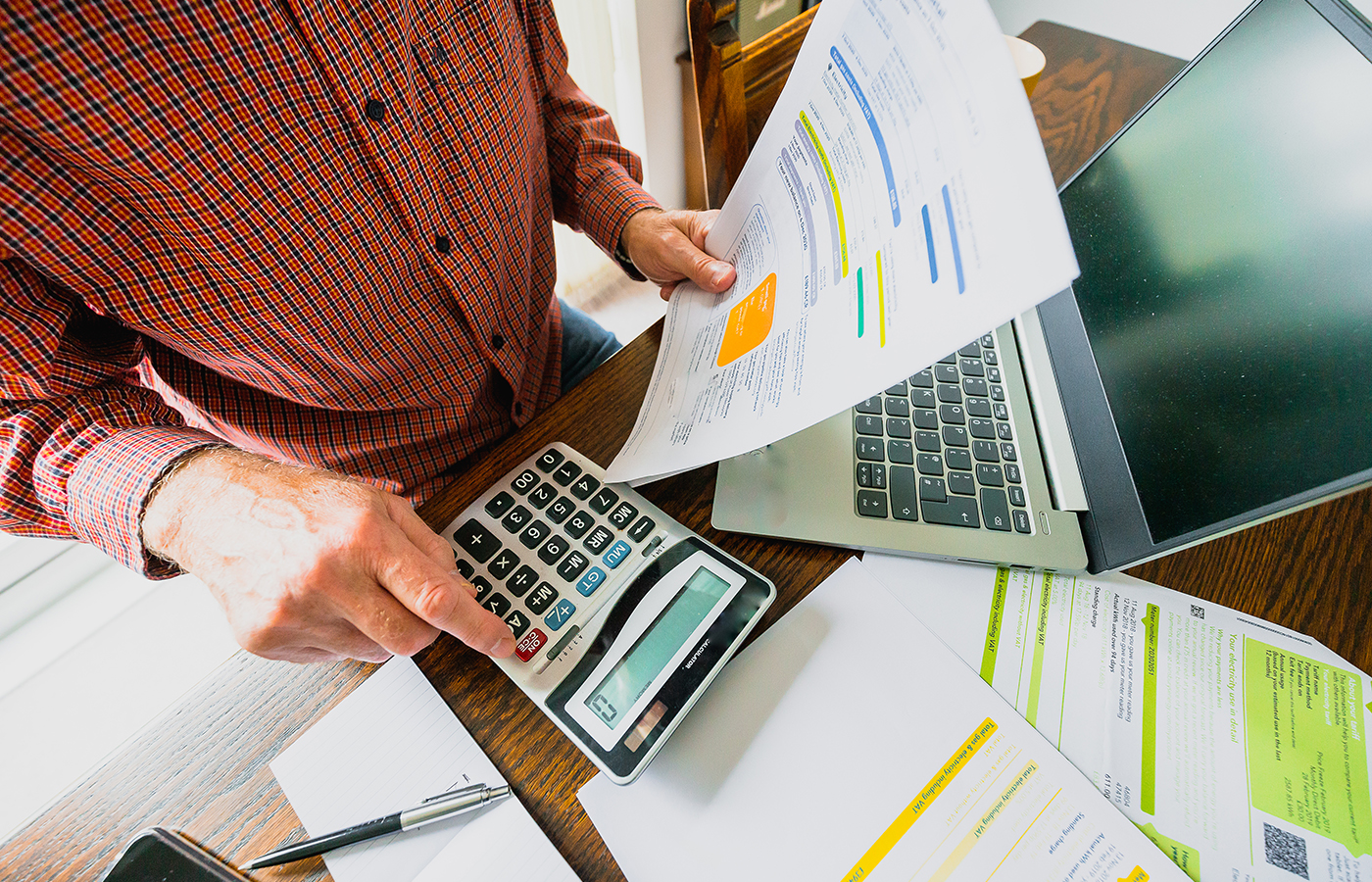
Next year, with a potential recession on the horizon, bank stress testing will be as vital as ever. Today, though, these tests remain too reliant on scenario projections of uncertain quality.
Why is this a source of consternation for stress-testing modelers, and what can we do to fix this? We’ll get to that in a minute, but first we need to consider the inefficiencies of the current environment.
Let’s start by pondering the following scenario: due to a bizarre funding irregularity, your stress testing budget is doubled for the next 12 months. Since you have always been skeptical of the exercise, you decide to commission two completely independent teams to produce identical reports based on the same underlying scenarios and assumptions. There is a baseline and two alternatives.
Both reports are professionally produced and easily pass independent model validation.
In terms of the results, both teams are basically aligned in their baseline view, predicting a 3% loss rate for the portfolio during 2023. Under the more benign downturn scenario, team A predicts a 5% loss compared to team B’s more pessimistic 8%. Under the severely adverse scenario, team A predicts a 25% loss compared to team B’s 12%.
So, the A team thinks the portfolio is more robust to mild shocks, but that once the dam breaks, losses will rise alarmingly. Team B’s view, in contrast, is rather more linear.
We have two equally plausible views of the same portfolio based on the same underlying assumptions. How should we decide which to believe?
We’re not used to thinking of scenarios as a form of statistical inference, but there’s no question that this applies. If the precise preconditions defined by the scenario narrative were to actually occur, the loss rate experienced by the portfolio would follow some unknown distribution of outcomes. The point of building statistical models for scenario analysis is to try to infer the parameters that define the form and the moments of this mysterious distribution.
The Statistical Uncertainty Dilemma
Needless to say, our estimates of the parameters are subject to error. In our thought experiment, the fact that the two teams produced different estimates is a consequence of a considerable amount of underlying statistical uncertainty.
When scenario results are presented to end users, however, this uncertainty is rarely declared. I’ve seen hundreds of reports over the years – and the projections are almost always presented as point estimates with no indication of the extent of possible error.
If portfolio stress testing was an experimental science, we could easily determine which of our two teams have the superior model. It would simply be a matter of establishing the conditions of the scenario in the laboratory and then running the experiment many times to capture the shape of the conditional loss distribution. It would then be a simple matter to determine whether team A’s or team B’s forecast was closest to the truth.
Back in the real world, we only observe one reality. What's more, in our timeline, the hypothetical scenario has never happened and will never happen. Something approximating the scenario may be possible: we could, for example, produce a distance estimator that measures the similarity between the reality we observe and that described by the hypothetical scenario. Moreover, if we then had 20 or 30 years of output from the two teams, we could eventually produce a measure of which team produces the best forecasts under which conditions.
Tony Hughes
Absent this kind of analysis, there can be no scientific way to determine which of our teams produces the best scenarios, either in prospect or in hindsight. As such, you could make your scenarios any way you like and no-one would be able to categorically refute your choice. The end user may simply have a feeling that one is better: do they prefer, say, Mark Rothko or Jackson Pollock?
A Plausible Fix
The discussion so far is very bleak for pure scenario analysis. Since we can’t evaluate the scenarios-driven models, we can’t identify techniques that are worth emulating; consequently, the methodology employed will inevitably stagnate.
In short, our thought experiment would have left our decision-makers more confused than enlightened, because they would now have access to two contrasting opinions, with no reasonable way to decide between them.
When stress-testing models are evaluated scientifically, it’s usually based on a criterion that is unrelated to the accuracy of the scenario forecasts. Most commonly, the models will be judged on their historical baseline forecasting performance, but, of course, team A and team B in our experiment were inseparable on this score.
Keep in mind, also, that a model that typically forecasts very well could be terrible at representing more extreme situations.
Some models may be invalidated on the basis of significance or diagnostic tests. Again, however, there is no reason to believe that the application of a particular test would be indicative of strong or weak performance in a scenario exercise. You could imagine gathering 100 models that all pass a particular diagnostic and yet still produce 100 very different projections under a stress scenario.
There is no basis on which to conclude that any of the proposed strategies discussed here for evaluating scenarios are superior to any other. With all that said, I think it’s important that scenario analysis not be the sole star of any stress testing show.
Stress-testing models should be used for a variety of purposes, including baseline forecasting and structural analysis. All stress-test reports should express a live prediction that could then be compared to actuals at a later date. What’s more, all reports should aim to identify a vulnerability or feature of the portfolio that has not been previously studied.
The point is that these more diverse and comprehensive stress-testing analyses, in contrast to those that are driven strictly by scenarios, can be scientifically refuted. Moreover, their quality can be rigorously determined.
When the results of a pure scenario analysis are presented, end users may mistakenly view it as rigorous or scientific. The producers of that analysis should strive to disabuse end users of this notion, and reports should make it clear that scenario forecasts are subject to statistical uncertainty.
Finally, research should be carried out to identify ways to measure the quality of scenario projections. This will not be easy. However, without these metrics, the discipline of scenario-based stress testing can never develop.
Tony Hughes is an expert risk modeler. He has more than 20 years of experience as a senior risk professional in North America, Europe and Australia, specializing in model risk management, model build/validation and quantitative climate risk solutions.
Topics: Resilience