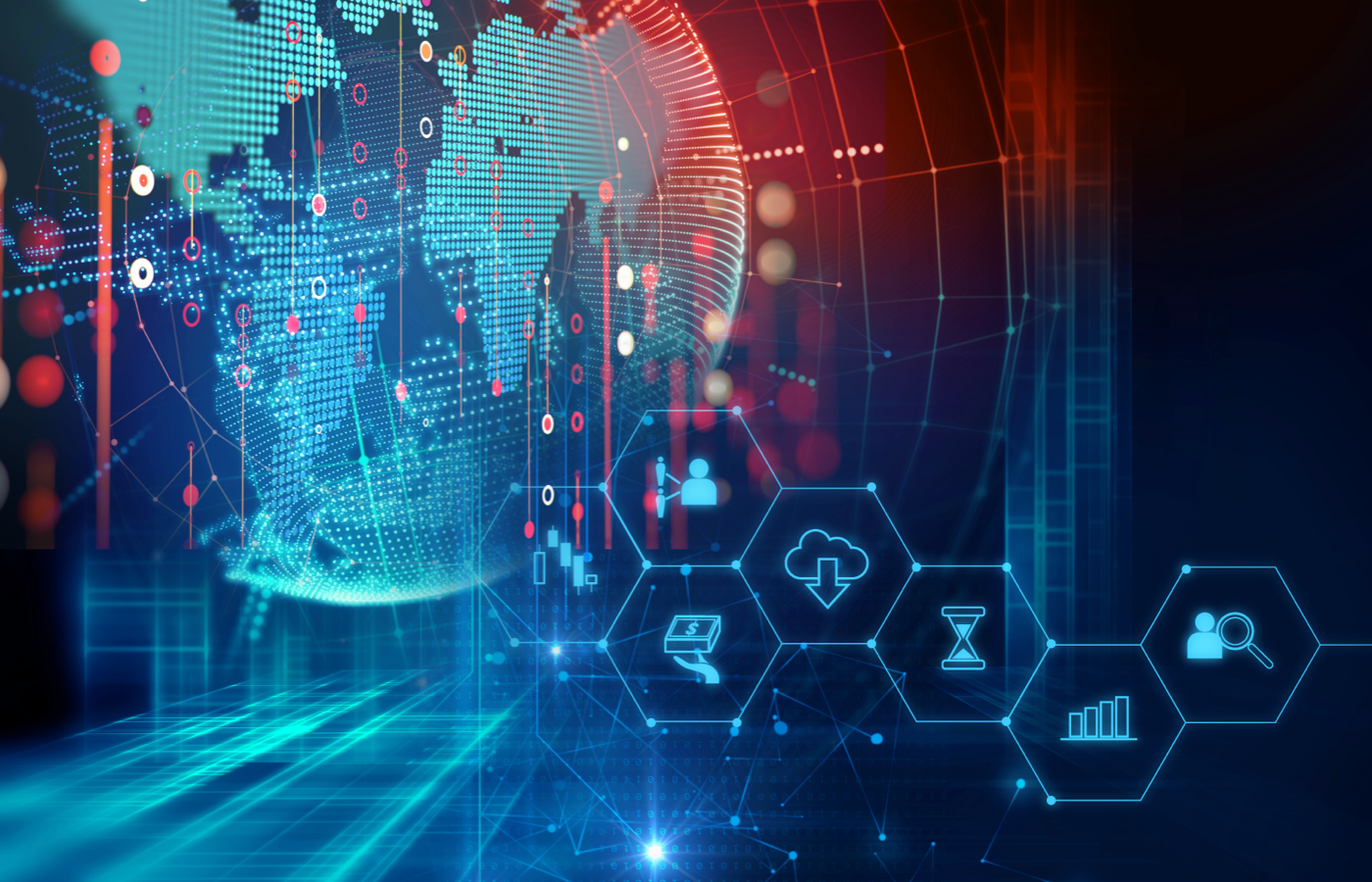
Market structural changes, machine-learning techniques and climate risk management initiatives will present some of the most interesting model risk management obstacles and opportunities for banks this year.
Clifford Rossi
While inflation today remains well below levels of the 1970s and early 1980s, we seem to be entering a distinctly different economic environment than we’ve faced over the last five years. Indeed, all the signals point to the beginning of an economic period that few risk managers today have experienced in their careers. For example, the Federal Reserve is set to embark on an accelerated plan to raise interest rates (while reducing its balance sheet), and the latest release of the U.S. consumer price index showed a 7 percent year-over-year increase in December – its highest rate since 1982.
Caution! Structural Changes Ahead
Looking at the chart below, we clearly see that we’ve been living in a generally declining interest rate environment for the last 40 years or so, with inflation averaging 2.64% since 1983. This implies that models used to assess interest rate risk, to forecast interest rates, and to price loans (as well as other fixed-income and derivative products) have been largely based on historical data from periods of relatively low inflation and interest rates.
Interest Rate and Inflation Trends: 1975 - 2020
But the modeling game is about to change. Upcoming market structural revisions will, in fact, have major implications for future models used to measure interest rate, market and credit risk.
Specifically, slowing economic growth, continued inflationary pressures and rising interest rates may take considerable air out of asset valuations in several markets this year. Moreover, the potential for new COVID-19 strains to emerge remains a looming risk to public health and markets.
One of many questions that will be discussed in asset-liability management (ALM) meetings in 2022 is how all of these factors will this affect interest rate risk hedging strategies, which are based on current interest rate risk models.
Modeling teams and risk managers must adapt their tools to unfamiliar economic conditions, and one ongoing challenge will be to strengthen linkages between well-understood economic factors and a model’s market and credit risk outcomes.
Opportunities to improve financial risk models in this new economic environment will arise from analytical insights drawn from other techniques (such as artificial intelligence) that may discern risk outcomes in data patterns from earlier periods that are reflective of higher inflation and interest rates.
Preserving the Art and Science of Risk Modeling
Increased scrutiny in 2022 will certainly be applied to machine learning (ML) and associated applications for risk measurement. Although ML techniques have been around for decades, advances in computational power have accelerated interest in these models.
ML models take into account certain issues that are not well-identified in standard parametric models, including inherent nonlinearities and interactions among risk factors. Indeed, considerable analysis has demonstrated the power of ML models over standard statistical models. However, while ML models have some advantages, we must not lose sight of the importance of business and economic intuition in the model-building process.
For the data scientist interested in mining large datasets for important analytical insights, ML models are a welcome tool. But the potential darker side of ML applications is that they can easily diminish one the most important skills a risk analyst possesses: namely, his or her instinct and intuition regarding fundamental relationships between economic and risk factors and associated risk outcomes.
Risk modeling teams must continue to explore ways of integrating ML techniques into mainstream risk management applications, while simultaneously preserving the essence of what makes a risk modeler valuable in the first place. It isn’t just their skill in accessing large databases, leveraging state-of-the-art software programs, or implementing the latest genetic algorithm for solving optimization problems. Rather, what truly makes a risk modeler a risk analyst is their ability to tell a credible story about risk, backed up empirically by the analytics.
Turning Up the Heat on Climate Risk Models
As banks continue to ramp up their climate risk assessment capabilities, one of the thorniest issues they face is how to leverage and assess the outputs of so-called climate and associated integrated assessment models (IAMs). Critical components of IAMs include economic damage and emissions abatement – cost functions that have been the subject of much debate over the years in terms of the accuracy of their results.
The general lack of experience with these models by bank modeling units – and the lack of transparency regarding model specification, key assumptions (e.g., model resolution and statistical downscaling techniques) and validation – put climate risk modeling and validation activities squarely in focus this year.
In his seminal article, the Use and Misuse of Models for Climate Policy, Robert S. Pindyk levies a damning indictment of IAMs, specifically citing the following drawbacks: arbitrary parameterization and assignment of model input functional forms; difficulty in understanding climate sensitivity impacts to the models; a paucity of data relating to damage functions; and poor characterization of tail risk associated with climate outcomes.
“In fact, I would argue that the problem goes beyond their ‘crucial flaws’: IAM-based analyses of climate policy create a perception of knowledge and precision that is illusory and can fool policymakers into thinking that the forecasts the models generate have some kind of scientific legitimacy,” Pindyk elaborates.
We should not just accept scenarios generated by climate and IAM models without performing model due diligence consistent with regulatory model risk management practices. Understanding the effects of climate and IAM model sensitivities on bank balance sheets and income statements is a critical step in this process.
Parting Thoughts
This year will present more challenges to risk modeling teams than they have experienced in a long time. Modeling teams will have to apply greater scrutiny to the validity of key data embedded in current financial risk models, thanks to evolving dynamics from the pandemic and potential structural changes in the way the economy works.
Coupled with increased interest in ML and climate risk models, risk modeling teams are sure to have their hands full. But the promise of adapting and leveraging these models to elevate risk management insights still burns bright.
Clifford Rossi (PhD) is a Professor-of-the-Practice and Executive-in-Residence at the Robert H. Smith School of Business, University of Maryland. Before joining academia, he spent 25-plus years in the financial sector, as both a C-level risk executive at several top financial institutions and a federal-banking regulator. He is the former managing director and CRO of Citigroup’s Consumer Lending Group.