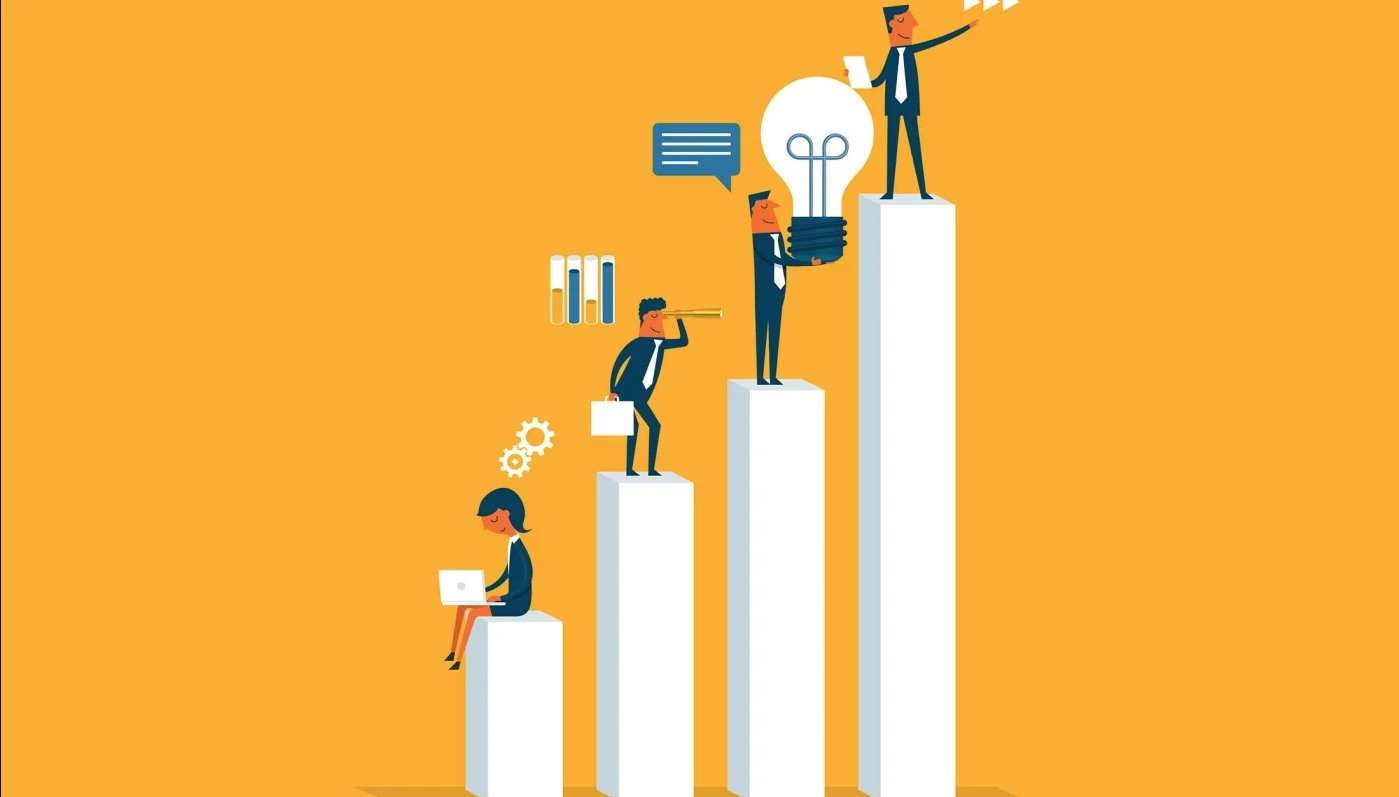
How well a financial services firm fares in a crisis isn’t down to luck. A large part of their success likely depends on the robustness of their model risk management (MRM) processes. By continually refining their approach, forward-thinking firms are setting the standard across the industry amid a shifting regulatory landscape.
Whether a firm is ahead of the curve or playing catch-up in MRM strategy and practices, there are five MRM best practices that can help them drive continuous improvement.
1. Take an interconnected approach.
A 2019 PwC report noted that firms’ “ultimate goal is a MRM framework fully embedded in business decision-making,” while in a global MRM survey report from McKinsey, published in 2021, half of respondents indicated that “automation [is] the most important approach for improving validation efficiency.”
Miles Elliott
Now let’s build on these themes and extend MRM beyond its specific boundary to explore interconnectedness – not the interconnectedness between models, as mentioned in our recent GARP contribution, but between the key aspects of the model lifecycle.
As the quotes above indicate, industry leaders recognize that greater automation will drive greater efficiency and that MRM must be integral to the firm’s decisions. The logical extension of this is to leverage an effective, automated MRM capability to:
- Shorten the time from initial identification of model risk to remediation of that risk.
- Mitigate the impact of the identified model risk during the time required to remediate that risk.
Why should firms strive to do this?
The winners and losers from the 2007-2008 Global Financial Crisis and the COVID-19 pandemic may offer the answer here. Those firms that were quickest to move from identification of the underlying risk to the remediation of it – while at the same time mitigating the impact of the underlying risk during the time taken to remediate it – were the firms that emerged from the crisis with the healthiest balance sheets, the strongest financial performance and the most effective customer processes.
How should firms tackle this?
With regard to adopting an interconnected approach across the model lifecycle, firms should consider the following:
- Early warning from model monitoring can help detect initial signs of model risk degradation. This requires a firm’s measures and tolerances policy to be connected to the materiality of a model’s movements and its impact on the firm.
- Model risk appetite defines when, how and to what extent firms are required to take action in response to changes in model risk. This requires firms to have a connected approach to model monitoring alerts and downstream action planning, be that for model re-calibration or risk mitigation.
- Effective data management allows firms to mine more deeply and broadly to enrich the determination of underlying risk. This requires firms to connect risk modeling teams to the right depth and breadth of data for analytics, validation and, of course, implementation.
- Risk modeling capability is the enabler that unlocks the determination (and validation) of underlying risk. This requires firms to have an agile and efficient operating model to connect what can be developed with what can be implemented at pace.
- Model deployment and execution capability is fundamental to acting quickly to remediate risk. This requires firms to connect agility, robustness and governance to ensure that pace is met with quality.
- As mentioned, it is crucial that firms shorten the time from initial identification of model risk to remediation of that risk. This also requires firms to take a connected approach to model monitoring alerts and downstream action planning.
- Firms must take actions in parallel during periods of model re-calibration/development to mitigate the impact of emerging model risk. This requires model risk processes to be connected to broader risk mitigation processes – for example, those associated with lending or collections – so that the right decisions can be made at the right time and in the right way.
2. Move to continuous assessment.
Only by continuously assessing, smartly adjusting, and iterating can firms use their model risk data to make more effective decisions. Manual reporting of model risk is time-consuming and makes it difficult to proactively identify potential problems.
David Asermely
Moving to continuous assessment – ensuring it is efficiently embedded into business-as-usual governance – allows firms to fully report model risk to internal audit committees, the board and regulators. It also helps everyone better understand the on-the-ground realities and adjust as needed.
A good starting point is for firms to ask whether they have the latest model risk data. Can they readily answer the questions that come up daily? Is their reporting automated, or does it require manual effort to produce comprehensive quarterly or even annual reports? To achieve business-as-usual continuous assessment, we suggest the following:
- Link model risk inventories directly to code and data repositories. Providing visibility of the model itself allows firms with numerous analytical tools to connect models with the central governance inventory used for organization-wide model risk reporting. This, along with the use of modern APIs, reduces the manual effort required to update the model risk system in line with the different analytical tools used.
- Automate performance, bias, and data drift monitoring. Most model monitoring is manual, and the results may not be fully accessible. However, with the right MRM system, firms should be able to assess the current performance of a given model and monitor other key metrics, such as explainability, bias reporting and how key factors are changing over time.
3. Use your MRM data in the right way – and understand the risk factors.
Firms may have the best model risk data in a robust platform that both orchestrates and enforces their MRM policies – but who exactly is using it? Do they review data in committees and audits? And how much do the regulators scrutinize it?
By organizing and delivering model risk data in an intuitive way, firms can more easily manage and report on it. Communication is critical. Everyone from users to auditors to executives must understand model status to properly assess the risk.
Teams should document model features, weaknesses, assumptions and limitations within a well-functioning model risk program, so the firm can assess models as conditions change. With so many variables impacting models – e.g., interest rates, oil prices, GDP and unemployment – firms must also understand which ones are most sensitive to change.
4. Use AI to govern AI.
When business leaders and risk professionals think about artificial intelligence, they don’t always consider the role it can play in governance. Yet universities are already using AI detectors to check whether students have used AI to complete their assignments – and with the rise of generative AI, it’s likely that detection software will become more prevalent.
So, how can firms apply the same method to MRM? A model risk system contains a large amount of information, including metadata, risk factors, performance and bias metrics, findings, model type, model reviews and documentation – and AI is a powerful tool for identifying relationships hiding in data.
Creating an AI-generated risk rating alert can ensure the MRM team is immediately notified when a model appears at risk. This can happen when similar models experience a problem or be based on macro factors to which the model is sensitive, such as inflation. A model validator can then assess whether the AI risk alert is appropriate and feed that information back to the model for supervised learning.
5. Connect model and data governance systems.
Model and data governance have grown separately in many financial services organizations – but it’s more important than ever to connect them.
With more effective data management, firms can mine more deeply and broadly to better determine risk. This means modeling teams need the right depth and breadth of data for analytics, validation and implementation. Firms must also understand:
- What data was used to train models.
- The extent of data drift between the training data set and live data.
- Whether the data suggests bias or quality concerns.
Finally, modeling teams must be vigilant to the fact that data could be manipulated by bad actors that influence models and decision-making.
Final Thoughts
In this article series, we’ve explored a number of themes relating to MRM – from how well-prepared firms are for regulation to what they can do to improve their MRM processes. The Prudential Regulation Authority’s holistic approach (set out in SS1/23) and the availability of advanced MRM technology should help firms beyond maintaining financial stability. With the right approaches and capabilities, firms can meet their obligations, better protect their customers from risks like fraud and grow their market share through innovation that boosts customer experience.
Miles Elliott, Risk Management Advisory Lead for EMEA at SAS, has over 25 years of financial services experience serving multiple banking groups, covering start-ups to global systemically important banks (G-SIBs) across both U.K. and international jurisdictions. He has held a variety of risk modeling roles, including risk modeler, Head of Risk Modeling and Chair of Model Risk Committee. He joined SAS in 2019 given his deep belief in SAS’ capability to power firms through their risk management transformations.
David Asermely, Global Lead for AI Governance and Model Risk Management at SAS, is responsible for product design, support, partner strategy and more. Passionate about translating data into actionable intelligence, he combines the best technologies and design principles to help financial services organizations improve modeling efficiency and quality. Asermely holds Master of Science and MEd degrees from the University of Massachusetts Amherst. Prior to joining SAS, he managed the Bank of New York Mellon’s Global Performance and Risk Analytics product set.
Topics: Model Risk