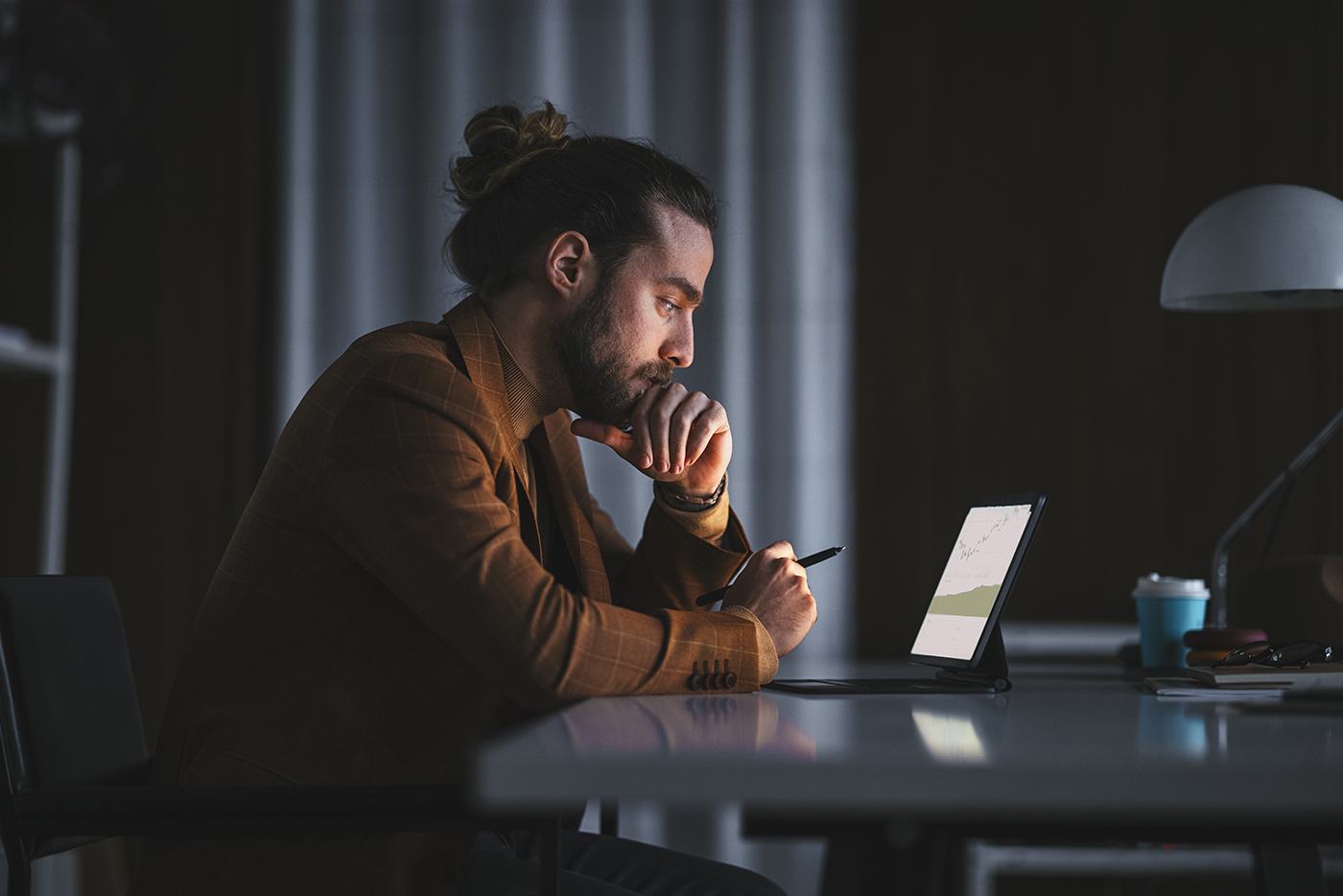
Having seen my share of risk models in the financial services industry, there are two conventional practices that I find especially infuriating. One is the overuse and misuse of statistical hypothesis tests; the other is that model uncertainty is rarely, if ever, explored.
I beg you'll excuse my self-indulgence here. It's the dog days of summer in the northern hemisphere, which seems like as good a time as any to wade into the mores of model- building methodology.
When we sit down to build risk models, we are faced with imperfect data and a blank slate. As soon as the starting pistol fires, we must use the data and our common sense to make a long series of potentially important decisions about the structure of the model.
Inevitably, we will commit a sequence of mistakes along this journey, simply because statistics is a probabilistic science. Estimators and test statistics sometimes have poor properties, and risk modelers tend to be fallible human mammals.
If the ideal model for a particular data set was written down and handed to us by a higher power, our predictions and inferences would still be imperfect; what we typically describe as "model risk," therefore, can never be fully eradicated.
Our aim when building a model is to achieve our objectives – whatever they may be – as efficiently and effectively as possible. To these ends, we want our selected model to be as close to the divinely-inspired version as we can possibly muster.
Wanted: Marginally-Better Forecasts
While the final application of the model may be to test theories, the problem of model selection, at its core, is not a testing problem. We don't need the selected model, from a statistical perspective, to be significantly distinct from the model perceived as second best; rather, we just need it to be marginally superior in apparent performance.
To illustrate, suppose we are forecasting and have a simple choice between an AR(1) and an AR(2) specification. Assume for simplicity that no other models may be considered.
We could solve this problem by testing the significance of the AR(2) coefficient at the 5% level. If the null hypothesis was rejected, we would use the AR(2); otherwise, we'd choose the AR(1). The problem is that the traditional formulation of a hypothesis test "protects" the null hypothesis: we’d only reject the null model, the AR(1), if a substantial weight of evidence supported the alternative. In building our forecast, though, we just want the model that produces marginally-better forecasts.
Ideally, we would choose the model based on that which performs best across a holdout sample. In a simple nested case like AR(1) vs AR(2), using a criterion like this is equivalent to running a test, but the implied significance level is typically much higher than 5%. Running the test at 5% is therefore in no way optimal when forecasting is the primary aim.
The interpretation of tests becomes much more complicated when the choice set is expanded beyond two, meaning that multiple tests (involving multiple models) will now be required to find the preferred model. Under this approach, the properties of every new test are conditional on all the mistakes that have been committed earlier in the process, making it virtually impossible to understand the properties of the tests being used at the end of a long sequence. It's therefore much safer to stick with the holdout sample prediction criterion when forecasting is the end goal.
Tony Hughes
When using tests – diagnostic tests, significance tests, whatever – the tendency across the industry is to run every imaginable test and to then believe that every test result is accurate. But just think about this in comparison to the field of medicine. Your doctor would never send you out for every medical test, because it is too likely that you will erroneously test positive for some rare condition for which you have no symptoms.
Running every test pays no heed to (1) the relevance of each test to the stated aim; (2) the underlying properties of the test (its power and size); or (3) the order in which the tests are applied.
Best practice would instead see tests used sparingly and selectively. They would only be used, in other words, if test properties were well understood. The results of all tests would also be viewed skeptically, recognizing that they are often prone to error.
Where appropriate, moreover, robust estimators and statistics would be used in place of diagnostic tests, removing the need to open the testing can of worms in the first place.
Model Uncertainty and Modeler Bias
The lack of consideration of model uncertainty is my second bugbear for contemporary modeling.
When we select a bunch of variables to help explain, say, default behavior under stress, we normally have a huge array of candidate variables to choose from. We can also try several different functional forms or introduce the variables with a range of lag lengths. There may thus be more variable choices than there are stars in the observable universe.
Focusing on variable selection, I could choose my favorite subset of variables and then set off to find a formulation of the model where all are seemingly significant at the 5% level, with intuitively correct signs. I could then present this to you as the one true model.
The problem is that the model I presented as "true" is actually one of many locally-optimal representations of the data. Someone else with a different view of the same problem could follow the same steps and derive an equally plausible, completely different model using the same data.
How can this form of model uncertainty be addressed?
One method – which is very easy to implement – is called extreme bounds analysis (EBA). This technique calls on the modeler to imagine all the models that may be favored by all the world's modelers. In the age of fast computers, this usually amounts to estimating every possible combination of variables from the established choice set.
When implementing EBA, several million different specifications will normally be estimated. If a particular result is observed in the vast majority of models, it can be viewed as "robust." Results that only crop up in the odd model are described as "fragile," meaning they are very sensitive to the assumptions made by the model builder.
Famous empirical results, widely considered to be "true," have been debunked using techniques like EBA. Can you really say that a result is solid if only, say, 20% of hypothetical researchers would actually find it?
Parting Thoughts
In stress-testing applications where models are plucked from the thickets, the variables the models contain are treated as beacons of truth that managers must pledge their devotion to as they set about managing their portfolios. Subsequent to model selection, you see committee meetings where the survival of the bank appears to hinge on the vagaries of a seemingly random, strangely-specific macroeconomic variable.
The world, however, doesn't really work that way.
A wise professor of mine likes to say that there is no free lunch in econometrics. Tests are imperfect and often misleading. Consequently, they must be carefully interpreted.
The seemingly-sound model you built may be fragile to the order in which the variables were considered. An equally-adept modeler may thus produce something very different, but just as defensible.
The thread that connects my two bugbears is the need for humility in risk modelling. You must accept that your analysis could be wrong or that your colleagues' modeling choices may be just as valid as your own.
Tony Hughes is an expert risk modeler. He has more than 20 years of experience as a senior risk professional in North America, Europe and Australia, specializing in model risk management, model build/validation and quantitative climate risk solutions.
Article Updated: May 20, 2024