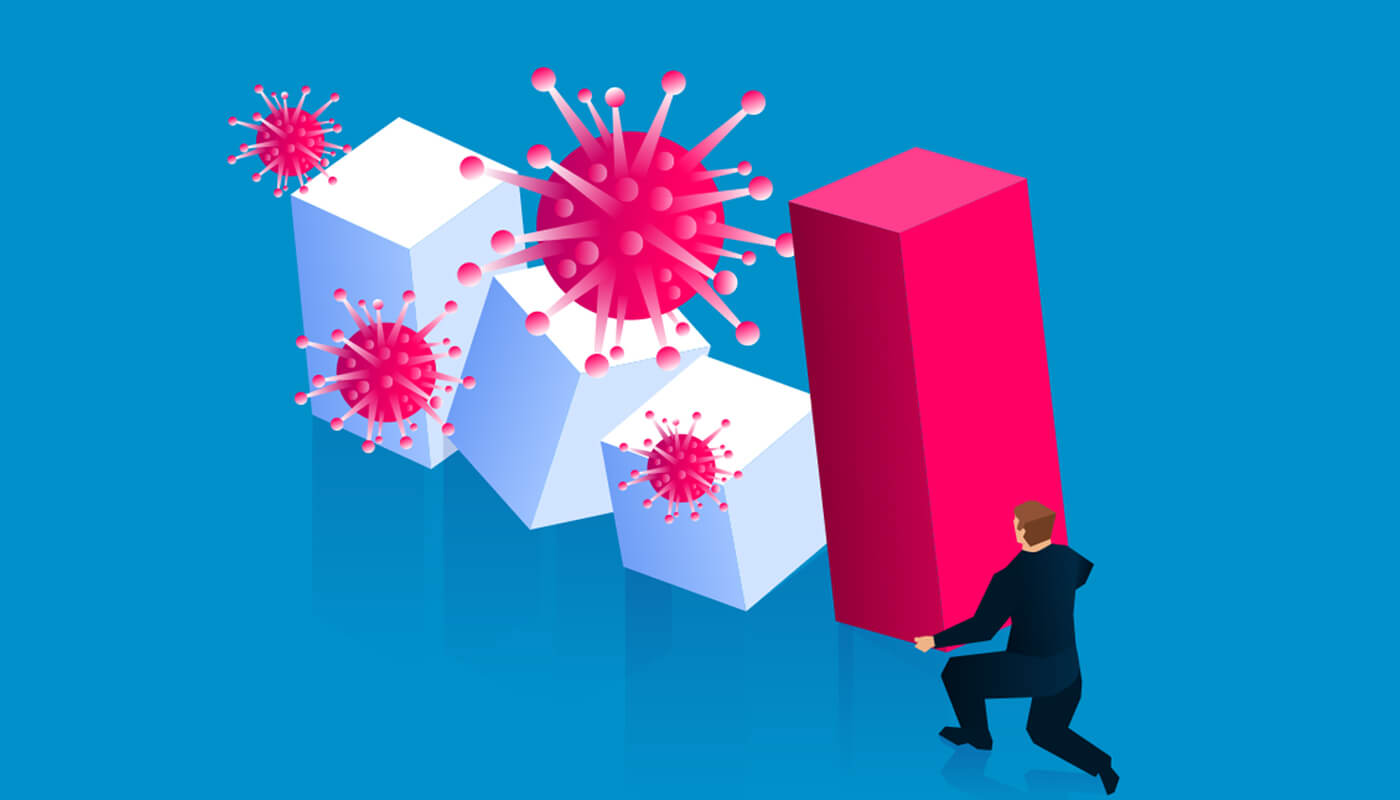
Over the next 12 months, as COVID slowly wanes as an economic disruptor, a lot of industry credit risk models will be redeveloped. Historically, these methods have provided superior predictions to those based on gut feelings - but most of them were sidelined during the pandemic, because they were unable to explain the odd behavior that was unfolding. At some point, though, model owners will need to grapple with the unusual data and rehabilitate their quantitative models.
As the dust settles, we can begin to survey the methods that are likely to be used to analyze pandemic data. As is always the case in risk modeling, the ability of senior business leaders to understand the models, to be able to use them correctly and to judge their strengths and weaknesses is paramount. This is a key concern, because statistical methods used to correctly capture the effects of COVID are likely to be more sophisticated than methods in common usage during the pre-pandemic era.
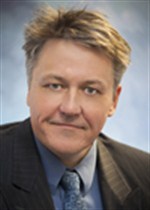
The simplest approach to the COVID conundrum will be to ignore it.
The idea is that the pre- and post-pandemic data will be pooled, and then modeled with no specific treatment applied to the impacted data. If COVID's real impact on borrower behavior (both during and after the pandemic) was basically inconsequential, this method will be most effective.
The downside of this approach is that if COVID was impactful on borrower behavior, the model's estimates will be biased. It will fit both the COVID era and the post-COVID era data poorly, and predictions will also tend to be rather inaccurate. The onus will then be on modelers to specify models to minimize these distortions, which may lead to some odd choices in variable selection.
Despite these abject weaknesses, I suspect that this pooled-data approach will turn out be the most used post-pandemic modeling method. Users will justify this choice by saying it is simple and consistent with past modeling efforts that have passed muster with regulators. Moreover, it also shelters business executives from having to stretch to understand slightly more complex statistical formulations.
Simply excluding the inconvenient, COVID-affected data from the modeling analysis in its entirety is a second dubious approach - but one that may nonetheless be used by some banks. This approach presumes that there is no useful signal that can be derived from COVID-era behavior that is relevant for successful prediction in a post-COVID world. Like the pooled-data method, it has the benefit of being simple, but, on the downside, it lacks any and all scientific merit.
If the new normal turns out to be a straightforward continuation of the old normal, this selective-data method will work fine - but will never be optimal. In fact, this, to me, is the ultimate manifestation of statistical “head-in-the-sand” strategy, and should be viewed very dimly by regulators around the world - if any banks choose to pursue it.
Tracking and Understanding Borrower Behavior: Alternate Methods
The next method involves the liberal use of dummy variables to capture the aberrant behavior observed during the pandemic. This approach is rather unadventurous. While it won't provide a lot of insight into the ways borrower behavior was affected by COVID, it is basically sound, easy to interpret and flexible enough to deal with some of the unusual dynamics observed over the last two years.
Specifying the exact structure of the dummies needed to control for COVID will take some effort. It will be very easy to pinpoint the beginning of the crisis, but capturing the impact of payment holidays and the gradual reopening of the economy will be difficult without excessive amounts of data mining. Largely because of this, the resulting predictions of this method may be quite sensitive to the exact specification of the dummies.
Once level shifts are introduced, an obvious generalization is to allow slope parameters to similarly vary over time. This can be achieved by interacting pandemic dummies with other independent variables.
What's more, to help explain the changed behavior observed during lockdown, the concept of using interactions can be further generalized to measure reciprocal actions between any combination of drivers - be they discrete or continuous.
If we could identify a variable or two that act as proxies for the progression of the pandemic, these could be interacted with variables traditionally used to model credit performance, allowing us to track behavior of borrowers through the pandemic. With these types of models, we not only start to develop an understanding of how borrower fortunes shifted but also get a sense of how performance may change as COVID becomes endemic.
When break points are determined by dummies, one common criticism of structural-break models is that the transitions between states are too abrupt. In the case of COVID, the beginning of the crisis might be well approximated by a such a sudden shift; however, on the back-end of the pandemic, when daily commerce gradually settled into new and pre-existing grooves, behavioral changes were likely to have been far more incremental.
In situations where behavioral change is more of an evolution, a commonly employed formulation involves the use of regime-switching models. These structures assume that data are drawn not from a single distribution but from a plurality.
In the case of COVID, we can imagine a scenario under which there was a pre-pandemic distribution, followed by one or more subsequent distributions shaped by a different set of characteristics. Under a regime-switching approach, a probabilistic switching function would govern which distribution is responsible for each observation. This mixing process would be able to capture behavior that shifted in response to a stimulus and then gradually drifted back toward its pre-stimulus state (or toward some new, previously unseen state). Such a model seems ideally suited to understanding our recent COVID adventures.
Parting Thoughts
When developing risk models for consumption by bank executives, there has always been a strong preference for simplicity. At the end of the day, models are used to aid decision making, which will be impossible if decision makers cannot understand how the model can help.
Sometimes, this thinking hamstrings modelers, most of whom would be comfortable calling up clever modern techniques to solve specific challenges. Indeed, most modelers believe that if we really want to understand the impact of COVID on borrower behavior, and to also optimize post-pandemic predictions, some of these more sophisticated techniques will need to be rolled out.
Back in the real world, a compromise outcome is far more likely. Some of the more dubious modeling choices will no doubt be discussed in banks, but should definitely be rejected. Models using dummies to capture pandemic-era data, though not ideal, seems like a reasonable compromise that business users should eventually be able to stomach.
Tony Hughes is an expert risk modeler for Grant Thornton in London, UK. His team specializes in model risk management, model build/validation and quantitative climate risk solutions. He has extensive experience as a senior risk professional in North America, Europe and Australia.