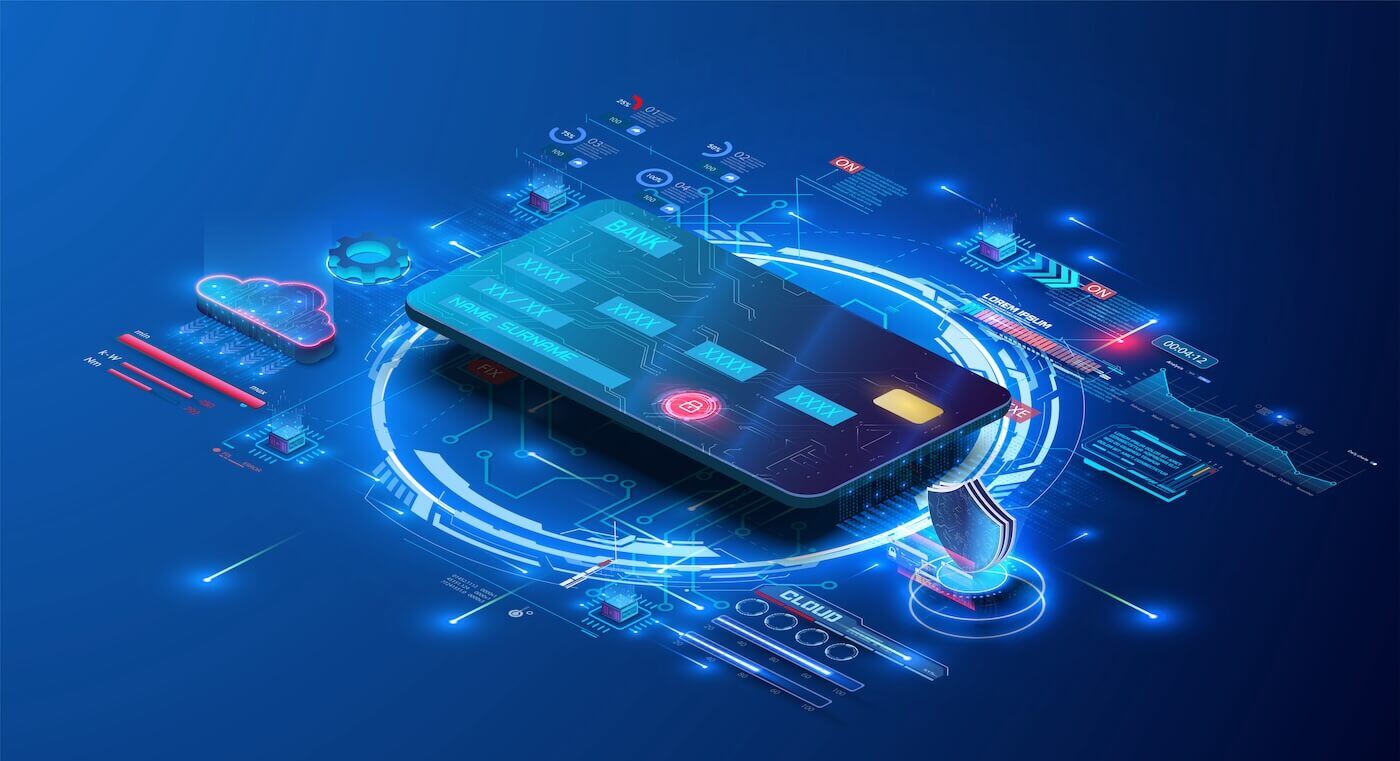
Complicated by cyber hazards and constantly evolving artificial intelligence, managing operational risk has never been more difficult for banks. Facing more regulatory scrutiny, operational risk practitioners must now consider what tools and techniques they need to implement to in response to the emerging threats.
Are banks prepared for the next CrowdStrike event? What about a direct attack on their own systems or those of their third-party vendors? To be ready, the banking industry must fast-track existing and emerging technologies while also leveraging novel approaches to managing operational risk from other industries.
Clifford Rossi
Before we consider the strategies and tools banks can employ to mitigate operational risk, let’s consider the current threat and vulnerability landscape.
Alarming Regulatory Assessments
AI has become a double-edged sword for banks. As we’ll discuss in a minute, they can use this innovative technology to combat operational risk attacks. But AI is also transforming the ways and means for operational risk events to manifest at banks.
Indeed, a recent U.S. Treasury Department study documented how generative AI capabilities are amplifying cyber and fraud risk at financial institutions. AI is being used, for example, to generate new malware code, or “data poisoning,” that is intended to compromise decisioning software at banks.
What’s more, AI-driven deepfake technology is being deployed to steal banks’ customer data in an effort to sell or access their accounts. These are no longer theoretical “what-if” scenarios, but, rather, very real threats that banks must confront as part of their operational risk readiness.
Unfortunately, the industry’s operational risk capabilities appear to be insufficient for this coming onslaught in cyber and AI-fueled threats, at least in the eyes of one U.S. regulator.
A recent Fortune article highlighted alarming “confidential assessments” from the Office of the Comptroller of the Currency (OCC). In these assessments, the regulator allegedly described 11 of the 22 large U.S. banks it supervises as having “weak” or “insufficient” operational risk management capabilities. Roughly one-third of those banks were also rated three or worse on the OCC’s supervisory ratings for risk – a direct sign of vulnerabilities in managing operational risk at our most complex and largest institutions. (The OCC also expressed concerns about cybersecurity at banks in its Semiannual Risk Perspective, published earlier this year.)
The Treasury report, moreover, illuminated the inability of many small banks to make proper use of more sophisticated technologies like AI. This technology capability gap between small banks and mid-sized and large banks indicates that the banking industry, as a whole, has considerable work to do – not just in catching up with today’s wide variety of complex operational risks but also with respect to addressing the latest AI threats.
What’s Next in Operational Risk Management?
Banks have for years quietly been building their risk management frameworks for operational risk and integrating them into their governance, risk and business processes. However, regular operational risk management assessments have become rote.
Standard monitoring and reporting processes create complacency among management and employees that operational risk is being managed effectively, if only because the tools to do so are physically in place. Financial institutions may therefore have a false sense of security that current practices are sufficient to control operational threats.
The good news is that there are steps that banks can take now to improve their operational risk capabilities considerably. These include learning and leveraging operational risk practices from other industries; understanding the interconnectedness of operational risks with other financial and nonfinancial risks; sharing data obtained through AI-based operational risk analytics; and prioritizing and expanding the use of the latest AI tools for managing operational risk.
Industries that have extensive experience in managing operational risk include power and water utilities, aerospace, and pharmaceutical and medical products. They operate the critical infrastructure necessary to ensure continuity of essential public services, national defense and health and safety, and their operational risk frameworks could be adapted to strengthen bank capabilities. It therefore makes sense for banks to study how these organizations increase awareness and sensitivity to operational risk among management teams and staff.
One example from the highly regulated pharmaceutical industry provides some insights. Pharmaceutical manufacturing operates under a set of strict regulations that require companies to implement current good manufacturing practices (CGMP). This includes quality risk management (QRM) and statistically-based process analytical technology (PAT) in manufacturing of pharma products.
These regulations are meant to ensure the management and mitigation of various risks throughout the product development lifecycle. Pharmaceutical companies and their regulators have very little tolerance for operational risk and therefore would be excellent sources for knowledge transfer to banking.
Aside from learning from other industries, banks can also employ agent-based or network models to perform microsimulations of their systems and processes. This will provide insights into how a bank’s customers, counterparties and internal and external business partners (including third-party vendors) interrelate.
These types of models have been used extensively in the past to comprehend the interconnectedness of financial systems. However, they could also be leveraged to understand not only how operational risks might ripple through a bank but also how emerging threats could fuel credit or reputational risks.
Op Risk Mitigation: Arguments for Sharing Data and Expanding AI
Banks have for a while now been using AI to manage fraud. But AI models can also be trained to identify other types of operational risk, and this data needs to be shared among banks.
A good data-sharing precedent can be found in the initiatives the American Bankers Association and the Bank Policy Institute have implemented to improve banks’ access to data about fraud risk. This type of pooling of resources could pay huge operational risk management dividends to all banks – but especially smaller institutions that lack the resources and size to acquire that information.
Tapping into innovative AI-based solutions is also necessary for banks to transform and elevate their operational risk capabilities. Generative AI has, for example, made enormous strides in recent years. When controls for generative AI applications are enhanced, this technology has great potential for managing the types of complex cyber and fraud risk that to date have remained aspirational for the majority of banks.
Existing cyber and fraud management tools have already been augmented through the improvement of anomaly detection and behavioral analysis methodologies. But these techniques must be supplemented by well-designed AI guardrails.
Parting Thoughts
We are at the cusp of a new era for operational risk management, which has for years been weighed down by overly bureaucratic and qualitative assessment methods. Many banks have built a consistent control environment for operational risk that accounts for business and risk processes, but this has often come at the expense of dulling operational risk awareness and sensitivity.
Today, with bad actors using AI technologies to wreak havoc at the institution and system level, banks need to fight fire with fire and begin ramping up their own AI-enabled operational risk capabilities. They can also gain insight and ideas from industries experienced in managing operational risk in critical infrastructure, and use lessons learned from past failures for network simulation modeling of interconnected risks.
Clifford Rossi (PhD) is the Director of the Smith Enterprise Risk Consortium at the University of Maryland (UMD) and a Professor-of-the-Practice and Executive-in-Residence at UMD’s Robert H. Smith School of Business. Before joining academia, he spent 25-plus years in the financial sector, as both a C-level risk executive at several top financial institutions and a federal banking regulator. He is the former managing director and CRO of Citigroup’s Consumer Lending Group.
Topics: Resilience