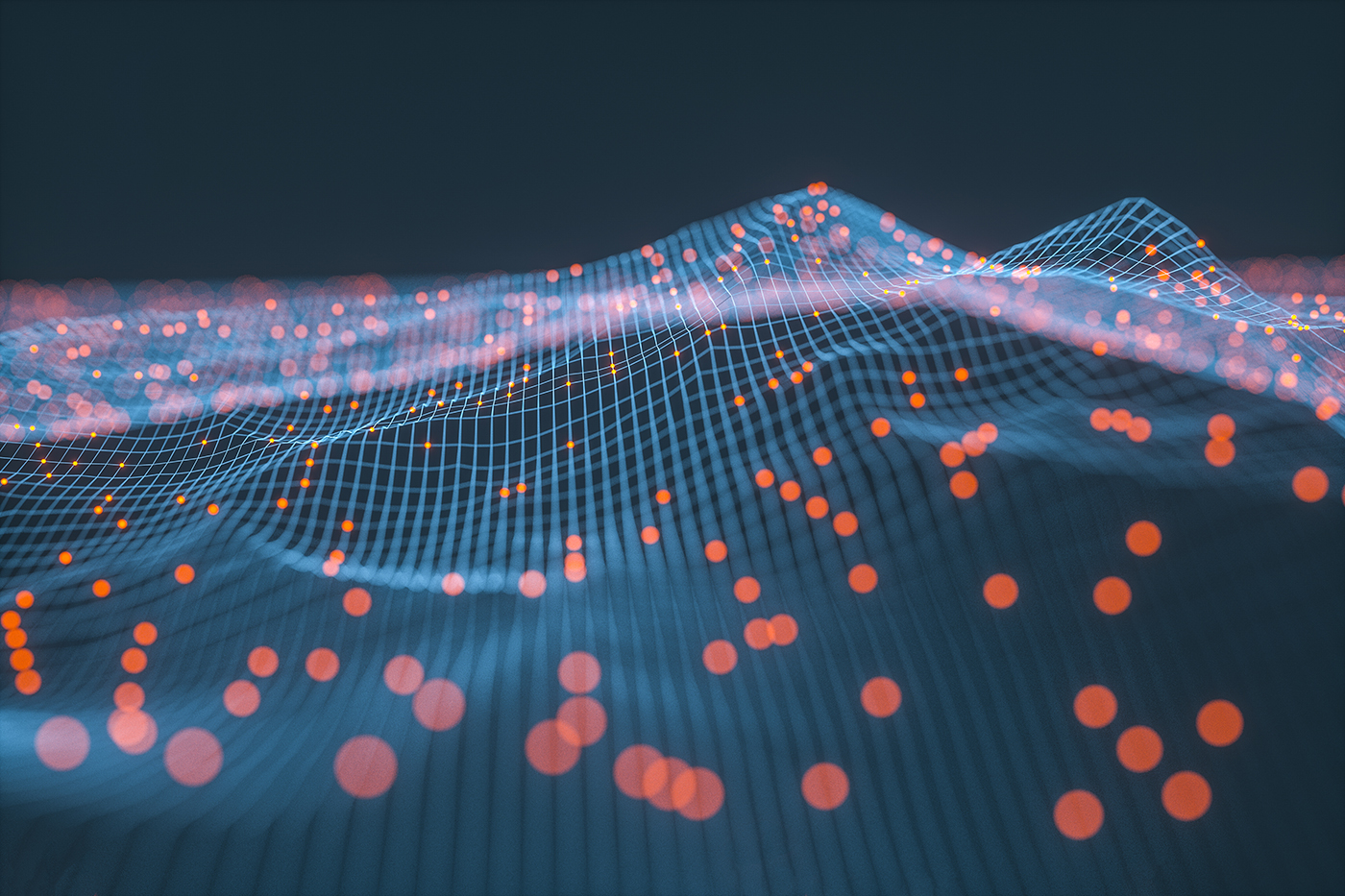
Whenever I question the statistical properties of scenario-based projections, people invariably remind me that the journey is more important than the destination.
But how should we assess the quality of the journey?
The notion of a valuable journey is that by completing the required steps of the annual stress test, for example, we gather so much useful insight into the nature of our portfolios that the obvious statistical weaknesses of the exercise simply don't matter.
The primary problem with scenario forecasts is that we don't know, and can never know, how accurately we are producing them. Because the scenarios never actually happen, we can't judge, with hindsight, the precision of past attempts. We thus cannot refine our methodology or even gauge the progress we are making in developing our art.
Tony Hughes
Consider these questions: Are we, as an industry, getting better at scenario analysis over time? What was the last major advance in scenario analysis technique? What is the incentive to innovate new scenario-based analytics? Does a particular new methodology or new data point significantly enhance the quality of our scenario projections? And does the champion model we're using really produce better output than the humble challenger?
There's no way to determine the answers to any of these questions from a stress-testing perspective. Meanwhile, for most other common exercises risk modelers undertake – like building credit scores – we can answer every single one of these questions with a high level of precision.
Analyzing the Journey
The aforementioned observations relate to the destination – the projections that result from the stress-testing process. Here, though, I want to focus on the journey.
Does our current approach produce good-quality travel? Would our experience be enhanced by using different models? Would we see more cool stuff on the way if we were aiming at a different destination?
In summary, how do we assess model risk when our sole focus is the quality of the journey?
In one sense, the answer to this question is easily within reach. All we need to do is ask the ultimate consumers of our research whether they find it useful.
When asked this question, many would say they do, but some – like JPMorgan Chase CEO Jamie Dimon – view the current stress-testing exercise as “arbitrary and capricious.” Moreover, even those who like scenarios might find it hard to enunciate precisely why they find them so compelling.
What is also clear is that scenario-based stress tests have little staying power in the public imagination. Regulatory stress tests are hugely consequential for investors, but results day rarely generates a substantial amount of commentary around the variety of risks faced by the banking system.
Limitations of Macro Scenarios
As a statistician on a perpetual search for rigor, I'd prefer to define what we mean by "a good journey," and then set out to try to measure progress along that path. Perhaps I'm losing the romanticism of bank stress testing, failing to capture its mystique, but these words usually don't apply in the prosaic world of financial regulation.
I would think that a good journey would provide a number of profound, evidence-based inferences that change perceptions of the portfolio. Such an exercise would identify factors to which the portfolio is especially sensitive. It would, moreover, uncover looming risks that might not have been identified through other means, like by reading the Financial Times or Wall Street Journal on a regular basis.
I would describe these aims, broadly, as structural analysis (i.e., setting out to test relevant hypotheses related to the portfolio) and data exploration (i.e., unsupervised machine learning).
An aficionado of scenarios might point out that these aims are covered by the existing process. The problem is that the models currently used are designed to reach the specified destination – in other words, to provide portfolio forecasts under stress scenarios.
If we only cared about the journey, we wouldn't try to construct such forecasts, which, as I stated earlier, cannot be properly validated. We would simply set out to analyze portfolio data using the best available models and methods.
Part of the reason that the scenario analysis journey is held in such high esteem is that only one route has ever been taken. It seems to provide a fruitful journey, but we know little of the benefits of the alternatives.
In the 13 years since the debut of the Supervisory Capital Assessment Program (SCAP) in the U.S., we have had some tumult – but also long periods of relative calm. Yet through this era, we have only run a small range of macro scenarios, always applying the same, severely-limited modeling methodology.
The Case for an Alternative Approach
Frankly, it wouldn’t kill us to try something radical, like building models that are based on more traditional forms of statistical data analysis. Such methods are amenable to rigorous evaluation and, for this reason, are clearly superior to scenario-based conditional forecasting.
Admittedly, it would be difficult for regulators to require banks to engage in academic-style research – which would be the closest proxy to what I have in mind.
One benefit of the existing system is that supervisors can easily identify banks that deviate from convention. If the stress test was more free-wheeling, with banks taking different approaches and employing different methods, it would become harder to set the appropriate minimum standard. It would also be more difficult to compare results across groups of peer banks.
On the other hand, we’d learn a lot more about the variety of risks facing the banking system. We’d be able to gauge our progress (e.g., by measuring improvements in model quality over time), and, by encouraging banks to explore other ways of assessing portfolio stress, we’d have a better chance of avoiding arbitrary portfolio assessments.
In other words, we can enhance the journey by leaving the scenario-analysis freeway to explore some of the back roads.
We’ll still reach the destination. While uncertainty about whether our scenario projections are any good will remain, we’ll at least have had a memorable journey.
Tony Hughes is an expert risk modeler for Grant Thornton in London, UK. His team specializes in model risk management, model build/validation and quantitative climate risk solutions. He has extensive experience as a senior risk professional in North America, Europe and Australia.
Article Updated: April 8, 2024
Topics: Model Risk