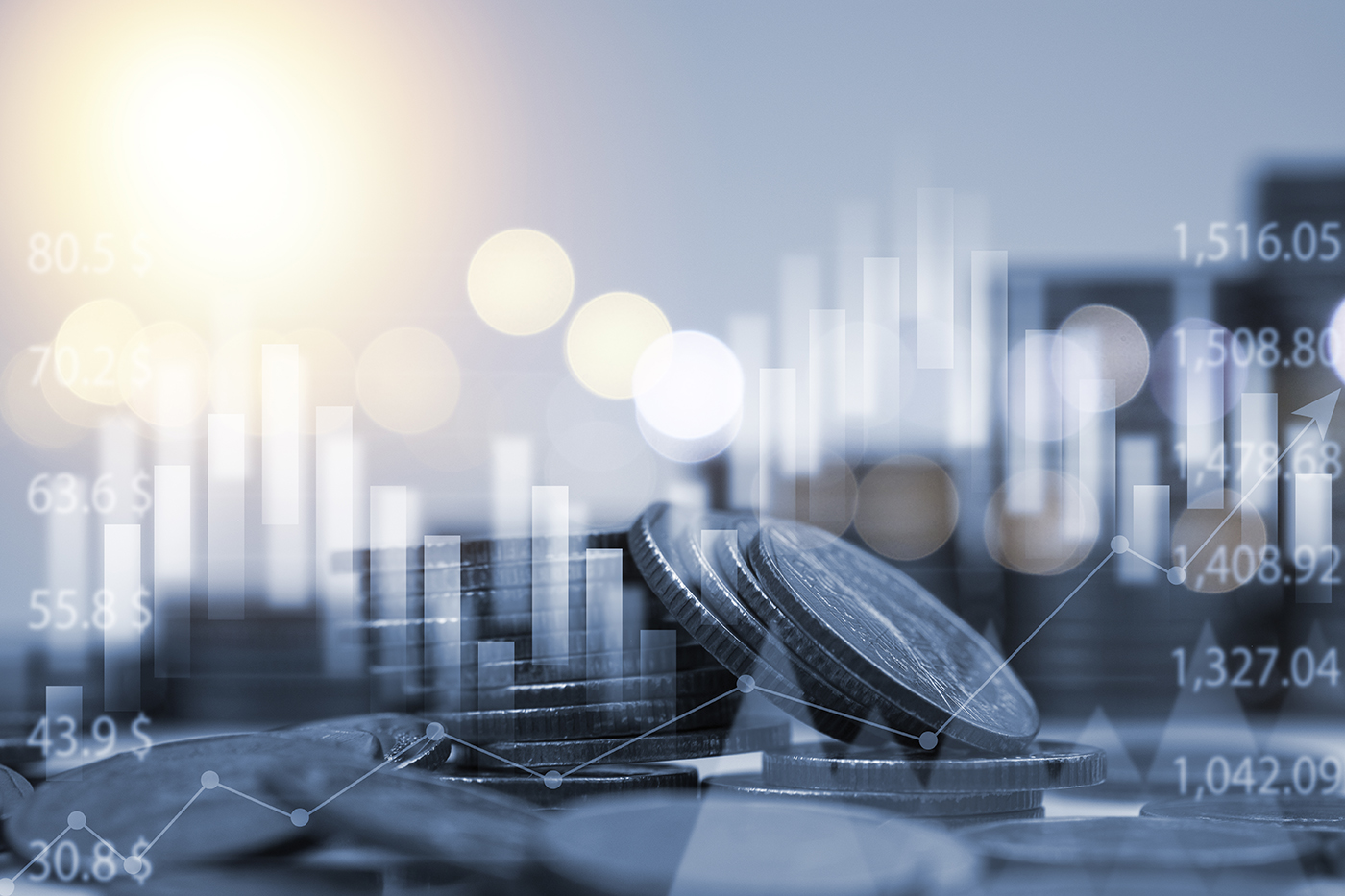
Risk managers grappling with multiple extreme events over the past couple of years must feel a bit like Dorothy and her Wizard of Oz associates, chanting a chorus of “Lions and Tigers and Bears, Oh My!” In the financial services community, these frightful beasts have taken the form of a series of nonfinancial events of such rarity and magnitude that they’ve humbled the best risk assessment and modeling teams.
Whether we’re talking about the pandemic or geopolitical unrest or the supply-chain crisis or extreme weather events (like the Great Western and European droughts), these extraordinarily challenging events have lately become the norm. All of these incidents, moreover, pose significant destabilizing risk to global markets, as well as to the health and general welfare of civilization.
Risk managers and their teams have struggled to modify their models to keep pace with the speed and uncertainty at which these events have moved markets and the global economy. Consequently, across the globe, risk executives are asking themselves, “What can we do to arm ourselves with tractable and reasonable approaches to incorporating these events into financial risk models?”
Tackling the modeling needed to understand these extreme risks must be a priority of all risk managers.
Challenges and Solutions
Extreme events tend to confound risk modelers, partly because of their rarity and lack of reliable data – but also due to their potential for high losses and their nonfinancial nature.
Given these challenges, how can we assess extreme events’ risk to credit portfolios? First, we need to separate the impacts of these events into direct and indirect effects.
Direct effects reflect the impact an extreme event has on an individual obligor and/or the underlying loan collateral, such as a commercial property. Teasing out these effects remains relatively elusive in the risk modeling community at the moment, and much more work needs to be done in this area.
Indirect impacts are those attributed to the effect an extreme event has more broadly on markets and the economy. Typically, these effects can create global shocks that translate into significant credit risk exposure.
Signal processing theory is one approach that can be employed to better understand risk from extreme events. The first aspect of this approach is amplitude, or the magnitude of credit loss and rate of change over time; the second is the wave period or duration of an extreme event’s impact on loss; the third is variability – i.e., the uncertainty of an extreme event’s impact on loss.
Estimating Damage with EERF
The vast majority of credit models in banking are statistical, based on developing relationships of various financial risk and macroeconomic factors – as well as credit risk derived from historical data. Most credit models feature macroeconomic data, alongside obligor-specific information. This provides a critical linkage between the risks an extreme event poses on the economy, society and financial loss.
One way to further integrate the effects from an extreme event and credit loss is by developing an extreme event risk function (EERF) that connects a risk driver of an event to some macroeconomic outcome (such as economic output or GDP) of interest. There are various ways EERF can be specified and there is ample precedent from insurance and climate modeling – via damage function estimations – to forge a path for other extreme event types.
DICE – an integrated assessment model that establishes the globally averaged temperature change as the risk driver of economic damages (the outcome variable of interest) – is one renowned damage function tool.
Clifford Rossi
Despite the fact that economists have, for years, identified and debated several limitations with the assumptions and estimates associated with these damage functions, they remain a mainstay of climate modeling – and are expected to improve over time. Making the damage function stochastic, for example, would allow modeling teams to explore a multitude of damage outcomes, rather than rely on a single expected value.
Once a functional form of the EERF and its risk driver F(t) have been identified for each extreme event at time t, a distribution of risk multipliers can be generated on a variable (such as GDP), which, in turn, can be introduced into a credit loss model to adjust current or baseline GDP. An example of a risk driver for a pandemic would be the hospitalization or death rate from a virus.
Figure 1 illustrates how such an EERF could be used in a credit model where GDP is the macroeconomic variable of interest.
Figure 1: Linkage Between Extreme Event Risk Function and Credit Loss
In this EERF example, the modeling team has identified the risk driver for the event at time t (e.g., the number of military combatants for a geopolitical conflict) as f(t) and generates a distribution of risk multipliers (<1) to be used to scale GDP in the credit loss model. If, for example, the baseline estimate for GDP in time t is 2% and a GDP risk multiplier from the EERF is .5, then GDP is set at 1% in the credit loss model.
The stochastic EERF allows the modeler to generate hundreds if not thousands of different risk multipliers, which, in turn, can be used to generate an entire credit loss distribution.
Parting Thoughts
In recent years, nonfinancial extreme events – including a pandemic, a supply-chain crisis, geopolitical conflicts and extreme weather incidents – have sent economic shockwaves around the world. Financial risk modeling is in dire need of techniques and data to properly link such events to financial loss.
To better understand how these rare events fit into our normal depiction of markets, and the economy, it is now imperative for us to redouble our efforts to build proper frameworks and capabilities.
Clifford Rossi (PhD) is a Professor-of-the-Practice and Executive-in-Residence at the Robert H. Smith School of Business, University of Maryland. Before joining academia, he spent 25-plus years in the financial sector, as both a C-level risk executive at several top financial institutions and a federal banking regulator. He is the former managing director and CRO of Citigroup’s Consumer Lending Group.
Topics: Metrics