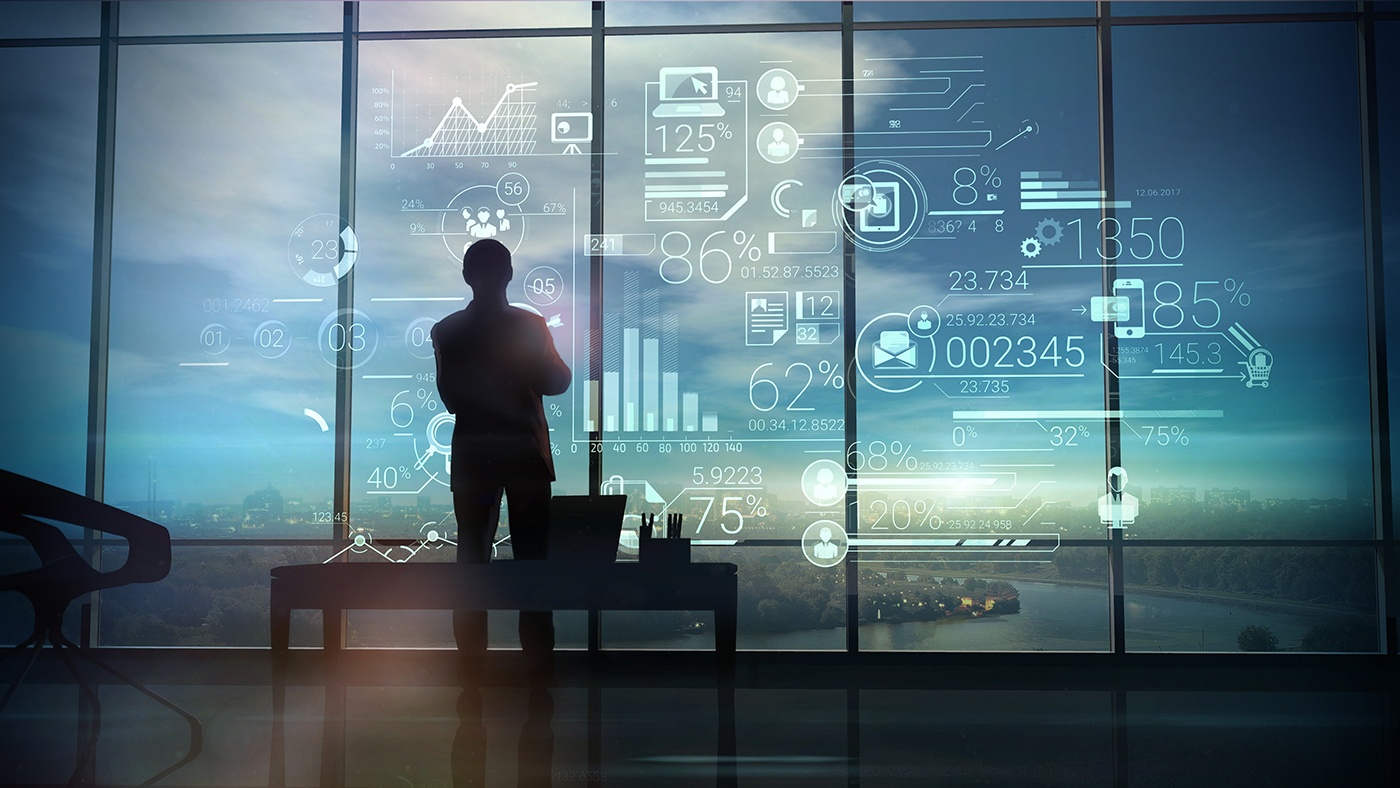
All across the industry, it seems, people have a penchant for “forward-looking” models. They are sick of crude “backward-looking” tools, and are instead keen to use “modeled” rather than “historical” data to overcome uncertainties associated with “future risk.”
Naively, I thought that future risk was tautological: i.e., that all risk is realized in the future!
But speculative, forward-looking models appear to be crucial these days. “Correlations in this cycle are totally different- if you don’t have a forward-looking way of looking at risk, you’re not looking at your risk properly,” the CEO of a tech-savvy British challenger bank once stated, in reference to the pandemic.
Tony Hughes
Accounting standard setters FASB and IFRS have also adopted the forward-looking mantra. The CECL method for expected loss provisioning, the standard in the U.S., “allows preparers to consider forward-looking information rather than limiting consideration to current and past events.” A similar feature is also present in the international IFRS 9 standard – a discussion of which explicitly ties “forward-looking information” to the use of scenarios in determining the appropriate level of returns.
I must say that this forward-looking information seems to be wonderfully important in managing risk. However, I wonder how this data can be identified and how we can gain comfort that it is reliable relative to the “crude” historical information to which we’re accustomed.
Moreover, if I’m keen to make sure that any model I develop isn’t backward looking, do I simply need to run a scenario through it to make it look forward? Surely, it can’t be this straightforward.
Historical Data is Not Passé
The first thing to realize as we explore these questions is that all data are historical. Every statistical model ever built, be it formal or informal, linear or nonlinear, Bayesian or frequentist, AI or non-AI, can be represented as a mathematical transformation of the available historical data.
Bayesian models may be based on a prior distribution that relies on externally sourced information, but even this should be developed from lived experience – and not simply plucked from thin air. Theoretical models should be supported by evidence, or at least be falsifiable in the here and now.
If a “model” is purely speculative - constituting an unsupported prior and nothing else - it does not meet the proper model criterion. It’s okay to speculate - your ponderings may even turn out to be correct - but it’s wrong and misleading to dress such activities in mathematical accouterments that imply an evidentiary basis.
If we build models to forecast input variables - hunting for the elusive forward-looking information - the specifications used to forecast the inputs must be consistent with the definition given earlier. It is often possible to construct forecasts of input variables that improve the core predictions of the model, but this is quite a fraught process.
Modeled data is always subject to prediction error, which will grow quickly as we extend the forecast horizon. At the end of the day, modeled data is just a function of historical data; the question is whether the transformation applied increases the quality of information passed to the core model.
There’s nothing particularly “forward looking” about anything we’ve discussed, so far.
The Irrelevance of Fanciful Scenarios
To my mind, a model becomes forward looking if it is specified to minimize some kind of ex ante prediction error. The issue is that even very simple loss forecasting and credit scoring models can often meet this criterion.
When such models are being built, so long as the modeler’s focus is on performance during an out-of-time holdout sample, they can justifiably claim “forward-looking” status. The models can then be evaluated by examining how well last period’s predictions stood up during the subsequent era.
But this is not as straightforward as it sounds. If the models are, for example, used for scenarios, working out the degree to which they are forward looking will be especially difficult.
A truly forward-looking specification will presumably capture the scenario more accurately than a backward-looking version. If the events actually occur, the forward-looking method will thus provide more accurate predictions of behavior.
Of course, in practice, we will never be able to make this comparison, because the precise scenario can never happen. If something roughly akin to the scenario pans out, we may catch a glimpse of superior model performance – but this is likely to be fleeting.
Scenarios must, at some point, be grounded in reality; they will lack any relevance if they are purely fanciful. We have observed portfolio behavior in the past; the scenario should then ask how such a portfolio might be expected to perform under a set of unusual conditions. If we start to imagine the portfolio performing much better or worse than it has historically, it’s hard to believe that such research has any analytical value whatsoever.
So, if we’re faced with two or more scenario projections, how do we decide which is more adept at looking forward – and, thus, superior? Alas, there is no way to reliably make this determination.
The fact that we can’t fully measure the effectiveness of scenario analysis is its primary – and ultimately, perhaps, fatal - weakness. Lamentably, risk managers often make enormously consequential decisions solely on the basis of scenario predictions that cannot be properly challenged.
In the context of scenarios, the lack of true validation means that anyone can claim that their methodology is looking toward the future and disparage anyone else’s for not enjoying that property. The term “forward looking” is therefore effectively meaningless.
Parting Thoughts
There is one final form of "forward-looking information" that demands attention: asserting something about the future that the forecaster just knows will be true, some day. Indeed, this is a common practice.
A modeler may, for example, declare that a particular relationship is non-linear, without providing anything (e.g., testing/rejecting the linear relationship) to substantiate the claim. Alas, faith in a particular concept does not count as evidence.
As part of the human condition, we face two unchanging realities: that everything we know about the world is gleaned from experience and that the future is inherently uncertain. Inferential statistics attempts to bridge this divide.
In trying to better understand the world, the best we can do is learn what we can from what we see and try to produce analytics that are conducive to sound decision making.
This process involves looking backwards much more than looking forwards.
Tony Hughes is an expert risk modeler for Grant Thornton in London, UK. His team specializes in model risk management, model build/validation and quantitative climate risk solutions. He has extensive experience as a senior risk professional in North America, Europe and Australia.
Topics: