A statement by regulators regarding technology implementation in the anti-money-laundering environment will have a significant impact on how financial institutions will select and implement new financial crime-fighting solutions.
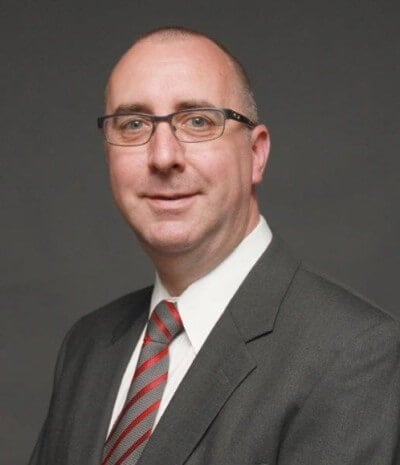
The December 3, 2018 joint statement - by the Office of the Comptroller of the Currency, Board of Governors of the Federal Reserve System, Federal Deposit Insurance Corp., Financial Crimes Enforcement Network (FinCEN), and National Credit Union Administration - encourages banks to take innovative approaches to meet their Bank Secrecy Act/anti-money laundering (BSA/AML) compliance obligations and further strengthen the financial system against illicit financial activity.
What makes this statement so significant?
Let's first focus on the environment. Fighting financial crime tends to be reactive in nature. Criminals have developed elaborate schemes to circumvent laws and go unnoticed. Financial institutions spend significant amounts of time and resources trying to identify illegal activity, with limited success.
It is estimated that less than 2% of the money being laundered is identified/seized. Traditional monitoring strategies are heavily “rules-based.” As new schemes are introduced, new rules need to be put in place.
Gradual Acceptance
In an effort to improve detection capabilities and control the operational costs of supporting AML programs, financial institutions have been looking for innovative alternatives. For the past year or two, virtually every AML conference, trade show, and tech sprint has offered multiple sessions on the benefits of artificial intelligence (AI), in particular machine learning (ML), and its application to anti-money-laundering operations.
Machine learning has been around for quite some time. It was first tagged “machine learning” as far back as 1959, though it gained most of its momentum in the 1990s. It was not until the last couple years that it found its way into AML.
Acceptance of the technology has been slow, especially with regulators and auditors. There was a concern that ML might produce lesser results than does hands-on scrutiny by human analysts - leading to missed signs of abuse or fraud.
One of the contributing factors was the lack of understanding of how to apply machine learning to AML programs, especially since many considered it to be a “black box.” AML models are expected to be clearly understood and explainable, with the inputs and outputs able to be validated for expected results. Machine learning steps away from the traditional rules-based models and is reliant on historical data and patterns to make predictions. The intelligence of those predictions develops over time and continues to become smarter as more data is available.
Transaction Monitoring
Initially, the focus was on the benefits to transaction monitoring. The application was in three different areas:
- Optimization of a financial institution's current monitoring model.
- “Predictive” alerts focusing on the likelihood of an alert resulting in a suspicious activity report (SAR) filing.
- Advanced anomaly detection to assist in identifying activities not being captured by the existing model.
Machine learning also has a role in the client due diligence space, assisting financial institutions in identifying those clients that pose a higher risk to engage in money laundering activities, as compared to their peers.
Regulators have been keeping a cautious eye; however, machine learning has mainly been used as guidance, with actual decisions and alert dispositions typically being performed by a natural person. This allowed organizations to take advantage of the benefits of machine learning without jeopardizing the health of their program.
New Attitude
The significance of the December statement is a major shift of regulatory views, from cautiously observing to promoting. The acceptance has grown so much that many regulators are currently looking or either pilot proposals or initiating their own pilots.
Financial institutions will need to maintain adequate solutions during any pilots; however, if any previously undiscovered money-laundering activity is uncovered by the new approach, regulators are offering a “safe harbor.” No penalties will be assessed due to their existing solution not being able to identify the activity.
The statement clearly outlined that those institutions with an adequate program in place, and which choose not to look for alternative solutions, will not be penalized. However, that stance will more than likely change as the applications prove themselves. As new, innovative solutions start to do a more effective job of identifying illegal activity, they will become the “new norm.” It will then be a matter of time until these approaches become a regulatory expectation.
Ted Sausen is a subject matter expert within NICE Actimize's AML line of business. His role focuses on ensuring that technology solutions align with regulator expectations and customer needs. A Certified Anti-Money-Laundering Specialist (CAMS), he previously led the global compliance analytics and technology group of a large financial institution.