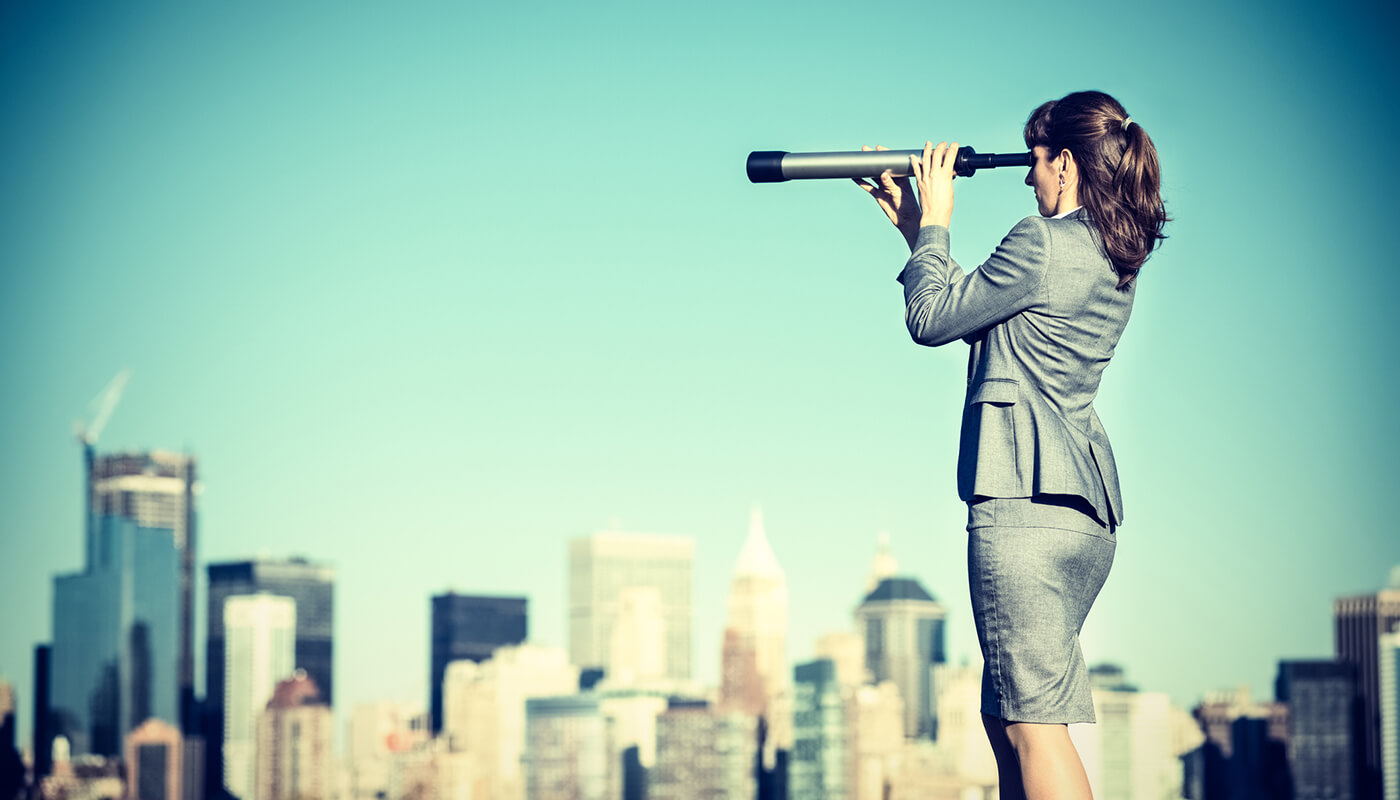
Lockdown restrictions during the COVID-19 pandemic have given many of us time to reflect on what matters in our careers and what we might hope to achieve in life once the crisis subsides. For some, this has meant leaving their current employment altogether. Since August, the U.S. labor market has experienced a “Great Resignation,” during which a record-breaking wave of 4 million workers have quit their jobs to find positions better aligned with their skills and interests.
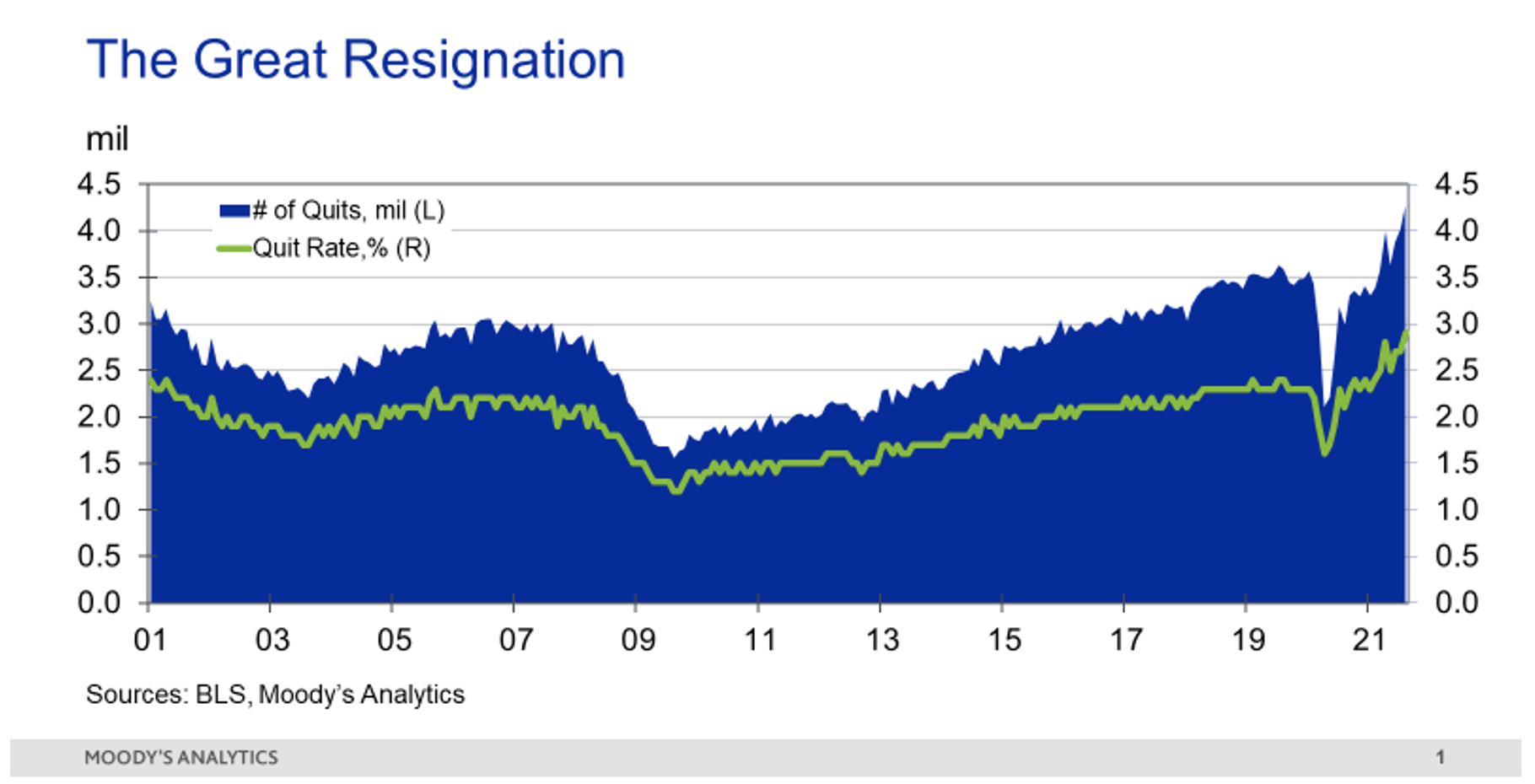
Time is, indeed, the fundamental driver behind most of our lives’ major decisions, yet its role in risk modeling is often overlooked. Analysts can become so concerned with accurately measuring asset values or collecting borrower financial information that they take time for granted. But this is a critical mistake.
Modeling: The Criticality of Time
The impacts of varying our time scales or modeling frequencies can be profound. Simply by truncating the forecast horizon, our views on the risk of an investment or a loan could shift by 180 degrees. Similarly, a project that might look lucrative when evaluated only at its starting and ending points may become unfavorable once we consider all the intermediate steps.
For example, suppose we are trying to assess the credit risk of a portfolio of auto loans. If we are interested in forecasting potential losses over the next month or quarter, we might choose a forecasting model, such as a transition matrix, that places significant weight on recent payment performance. After all, most of the defaults over this short time horizon are “baked in the cake,” coming from borrowers who are already struggling to pay their bills.
While the simplicity of this approach is attractive, it would be inappropriate for a forecast horizon going out several years or the entire life of the loans. Losses a year or two from now are likely to be caused by external forces or future adverse economic shocks that aren’t captured in current delinquency rates. A survival or hazard model that can account for time-varying covariates would therefore be a more effective approach for assessing the impact of specific economic scenarios - or for assessing the impact of simulations, if we are interested in measuring the upside and downside uncertainty around a baseline view.
Depending on whether we focus on a short or long-term view, our answer to the question of whether a specific portfolio is a safe, low-loss or high-risk opportunity may vary considerably.
The Future is Now
Though seemingly straightforward, the choice of a forecast horizon may be complicated by forward-looking markets. Take the case of the nine-quarter stress testing exercise mandated by the Federal Reserve and other central banks. While the required forecasting horizon for reporting results is obvious, events forecasted to occur after this event horizon could still have a substantial influence on prices and asset values within our nine-quarter outlook.
To illustrate this point, suppose a borrower is scheduled to repay a loan 10 quarters from the start of our forecast. If we know that the borrower is already under financial stress and unlikely to be able to make this payment, the value of the loan would fall immediately - and a prudent lender would begin to reserve for losses well in advance of the realized event. This behavior is even more apparent in corporate debt markets where investors discount traded securities immediately, at even the slightest suggestion that a company’s earnings may be weaker in the future.
The impact of forward-looking markets is increasingly relevant as we consider the assessment of climate risk on financial assets. With sea levels and storms projected to increase exponentially 50 years from now, commercial property lenders underwriting 10-year loans may believe they are able to safely ignore these threats. Even a 30-year residential mortgage, moreover, will be paid off well in advance of the worst projections. Mortgage debt investors can therefore sleep easy at night, no?
There are two key flaws with this logic. The first is the probabilistic nature of climate risks. Flood risks are increasing across geographies and don’t respect the boundaries drawn on flood maps. The likelihood of widespread flooding might reach a critical threshold 50 years from now (unless mitigating measures are adopted) - but even modest increases need to be incorporated in our risk assessments. Chances are, in fact, that more floods will occur in areas previously deemed as safe.
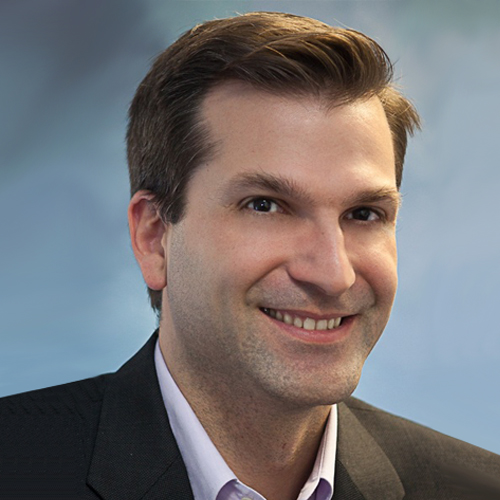
The second flaw is our time-sensitivity logic is that, in a well-functioning market, future climate risks will be reflected in asset values instantaneously. Higher property insurance premiums in areas with rising flood risk will increase borrowers’ user costs. This will lower the present value of properties immediately, leading to an increase in estimated mortgage default risk.
The pull-forward effect of future risks on current property values means that even when risk managers have short forecast horizons, they cannot simply ignore long-term events such as climate change.
What’s the Frequency, Kenneth?
In addition to understanding their “true” forecast horizon, risk modelers need to carefully consider the frequency of time used in their analysis. Should they forecast expected losses with a daily, monthly, quarterly or annual frequency? Or should they instead estimate a single cumulative probability of an event over the lifetime of a loan or other asset?
Higher-frequency forecasts provide greater flexibility, but also significantly increase complexity. Analysts need to balance the potential for additional forecast precision with the possibility of introducing noise into their estimates and increasing the operational risk of maintaining a more complex system.
Ultimately, there may be measurement issues that constrain modelers’ choices. For example, loan payments may be reported monthly, making a daily frequency impractical. Similarly, economic driver variables may only be reported on a quarterly or annual basis. Interpolation of intervening data points is an option, but carries its own set of assumptions and may introduce a false sense of precision.
At the other extreme, a model of cumulative behavior may be too coarse, failing to account for specific intertemporal dynamics that could significantly alter the trajectory of losses or profits. For example, a sharp decline and increase in interest rates could influence prepayment behavior in a consumer loan portfolio, sharply reducing the number of loans expected to default relative to a flat interest rate environment.
Parting Thoughts
When it comes to dealing with time, risk analysts should heed the warning on a car’s rear-view mirrors: “Objects in mirror may be closer than they appear.”
How carefully risk managers define time horizons and frequencies can have a significant effect on their assessments. In some instances, their conclusions of what is an acceptable or unacceptable risk may be reversed completely, simply by curtailing or extending the forecast horizon.
Beyond the direct impact that decisions related to time can have on quantitative models, we may also want to consider how our own lifespans could be distorting our views or introducing biases, especially when it comes to explaining seemingly irrational behavior. But let’s take it one step at a time.
Cristian deRitis is the Deputy Chief Economist at Moody's Analytics. As the head of model research and development, he specializes in the analysis of current and future economic conditions, consumer credit markets and housing. Before joining Moody's Analytics, he worked for Fannie Mae. In addition to his published research, Cristian is named on two US patents for credit modeling techniques. He can be reached at cristian.deritis@moodys.com.