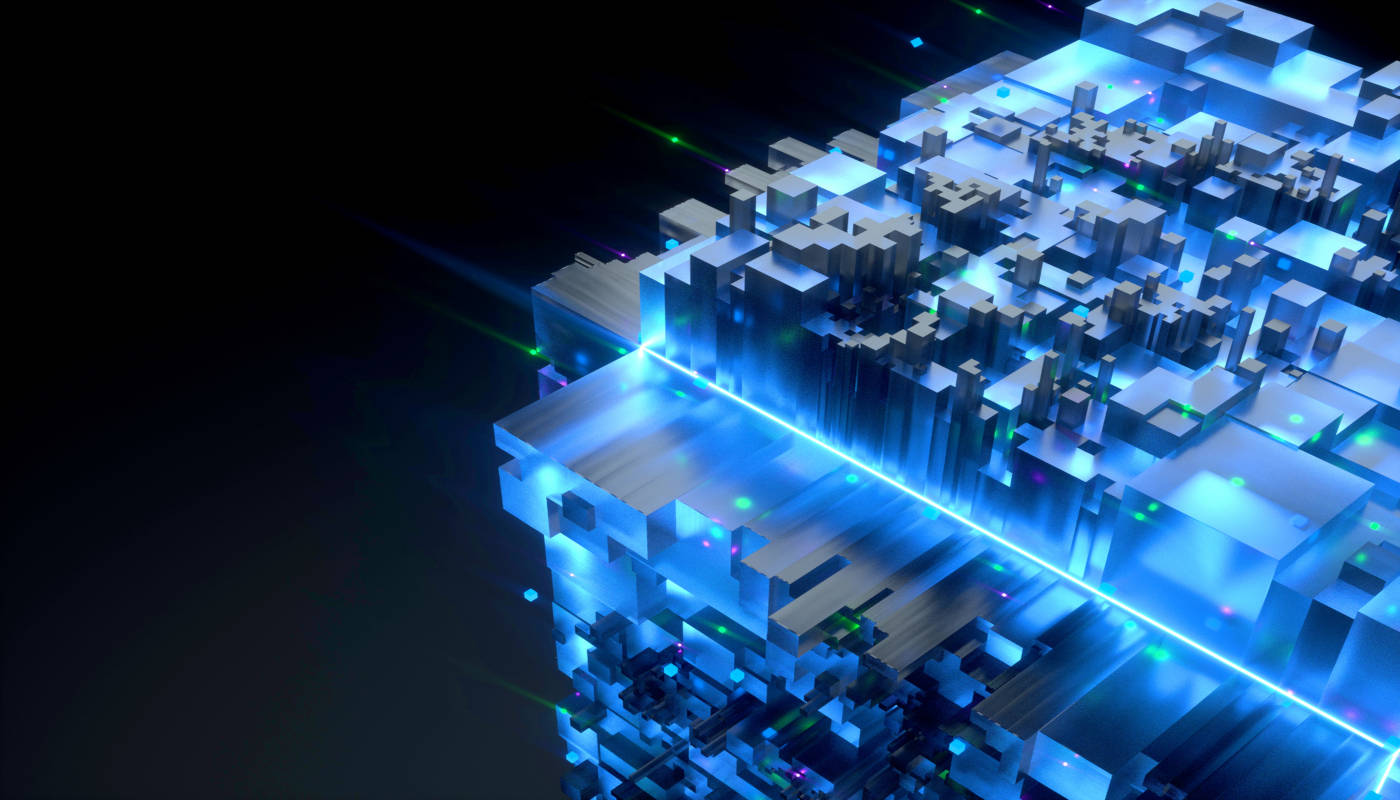
When working earlier this year in San Francisco, I found myself in a small breakfast restaurant in the Embarcadero area, where I was enjoying breakfast and the newspaper. Since the place was otherwise very quiet, I couldn't help listening to the conversation that took place in the booth next to mine. Two risk professionals were discussing the potential of AI in credit risk.
“AI is going to be big in credit risk and this is already happening,” the gentleman said. “There is much more data out there that can be connected to current client data. Think about personal data that can be gathered from social media. As long as this is data on public profiles at social media and the bank can connect their IDs to these profiles, data is enriched big time.”
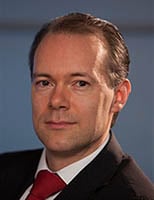
His female colleague, Elena, retorted: “I am not sure, John. What I see is that there are still lots of practical obstacles to overcome when joining these data, and then there's the question whether firms are allowed to store this information and work with it.”
John subsequently argued that AI is already enriching input data for probability of default modeling. He cited people sharing happy “almost payday” posts on Facebook, arguing that this is a good indicator of their credit status.
Elena then asked whether there were any academic studies that could back up this hypothesis.
Banks that want an edge, John replied, should commingle client data with data that is available through public platforms and sold by intermediaries. He conceded, though, that he's not certain whether there are strong academic studies that support his point of view on AI.
Doubts about the relevance of certain data were then expressed. “I mean, people sharing their holiday pictures on Instagram, what could one make of that?” Elena asked.
At this moment, I decided to approach John and Elena, noting that I'm in the credit risk profession, and asking them if I could join their conversation. After welcoming me, John said that we can distinguish between data that is useful and data that is not with the help of AI. This can be done, he elaborated, through trial-and-error.
“Yes, I see that, but you can also find pseudo-drivers. Causes that look good for a start, and then turn out to be fake,” Elena cautioned.
Whether AI can prevent overfitting and filter out such mistakes is up for debate.
Big Data is Not Smart Data
Questions about potential bias then arose, with Elena asking whether PD models should be altered if Facebook data showed that, say, all people with red hair present an increased credit risk. “If it is confirmed in a test set and a validation set, I don't see why not,” John replied.
Elena then argued that certain types of data have nothing to do with credit risk. “AI reaches higher levels of explanation than other more traditional techniques,” John countered. If the relevance of a group of data is confirmed in cross-validation of several samples, he elaborated, then that information should be trusted.
But what if credit risk professionals can't explain the drivers behind such data?
This, John explained, is where AI can be truly beneficial, using critical thinking. “It can open up a whole new way of looking at credit risk, outside of current convictions,” he reasoned. “It is light in the dark. There used to be very few acknowledged drivers - say, loan-to-value, loan-to-income and past behavior, like arrears in the past - that were being used. But there is a whole area of other possible drivers that have been neglected in the past that can now be used - thanks to big data and AI.”
Elena countered that big data is not necessarily smart data. “If I understand your argument, this never stops,” she said. “One should collect as much data as possible, without any direction, including pet pictures and happy socks, in the hope that these very sensitive algorithms find some kind of association.”
Savvy financial institutions, she elaborated, should aim to collect and analyze relevant smart data, rather than big data. “In your big data, a lot of data points are redundant, pointing in the same direction. Smart data means collecting additional data that is orthogonal. If you can do that, the predictive strength will appear in both traditional models and AI models. You don't need a random forest to pick it up,” Elena reasoned.
At this moment, 8:30 a.m. was approaching, and we all had to head to our respective offices. We exchanged phone numbers, and would save the rest of the conversation for a later day.
Baby Steps
A couple of days later, John called me early in the evening, expressing his excitement about the potential of AI to “change everything” - particularly in the area of credit risk modeling. He wanted to know whether I favored the arguments made by him or Elena, and I asked him to give me some time to perform additional research.
The day after, John called back, asking if I was sold on the effectiveness of AI in credit risk modeling.
“The strategic studies are certainly impressive,“ I replied. “However, from the academic research, I cannot distill a clear picture. In some cases, AI does not really increase the performance, as compared to a traditional model.”
John conceded that AI is still in its early stages at financial institutions, but re-emphasized his strong convictions about its predictive power, especially when combined with big data. “We're currently taking baby steps. But with big data, everything will be connected and, from the zillion datapoints, the algorithm will predict whether you will be paying the interest on your loan next time. They will know it better than yourself,” he concluded.
The societal picture John was painting was not attractive to me, and I also have concerns that an over-reliance on AI and big data for credit decisions could lead to an enormous data overload at single institutions. “I do agree with Elena's point that big data does not sound like smart data. Even apart from the privacy angle,” I told John.
Parting Thoughts
When reflecting afterwards on the discussion between John and Elena, I had to admit that there are no easy answers. High hopes have been attached to predictive modeling, but we have also seen reports of AI not delivering on its promises outside of finance - particularly in fields such as healthcare.
To determine whether AI is truly effective in credit risk modeling, case studies that dig deeper than the occasional “challenger model” need to be developed. The studies need to evaluate whether AI-based PD models are truly enriched with relevant data outside of traditional drivers - as well as whether new drivers are tainted by bias.
These drivers must be explainable, at least ex-post (i.e., after their importance has been confirmed in the classification model). What's more, the generalizability of AI-based models beyond the time frame of training and test sets must be discussed.
Supervisors have an important role to play here, since they can establish standards to which predictive modeling for PD should adhere, with clear boundaries to address the ethical concerns.
For the future development of AI within financial risk management, John and Elena need each other. It is only while defining reasonable expectations and respecting boundaries that John's AI ambitions can be fulfilled.
Dr. Marco Folpmers (FRM) is a partner for Financial Risk Management at Deloitte Netherlands.
Article Updated: March 26, 2024