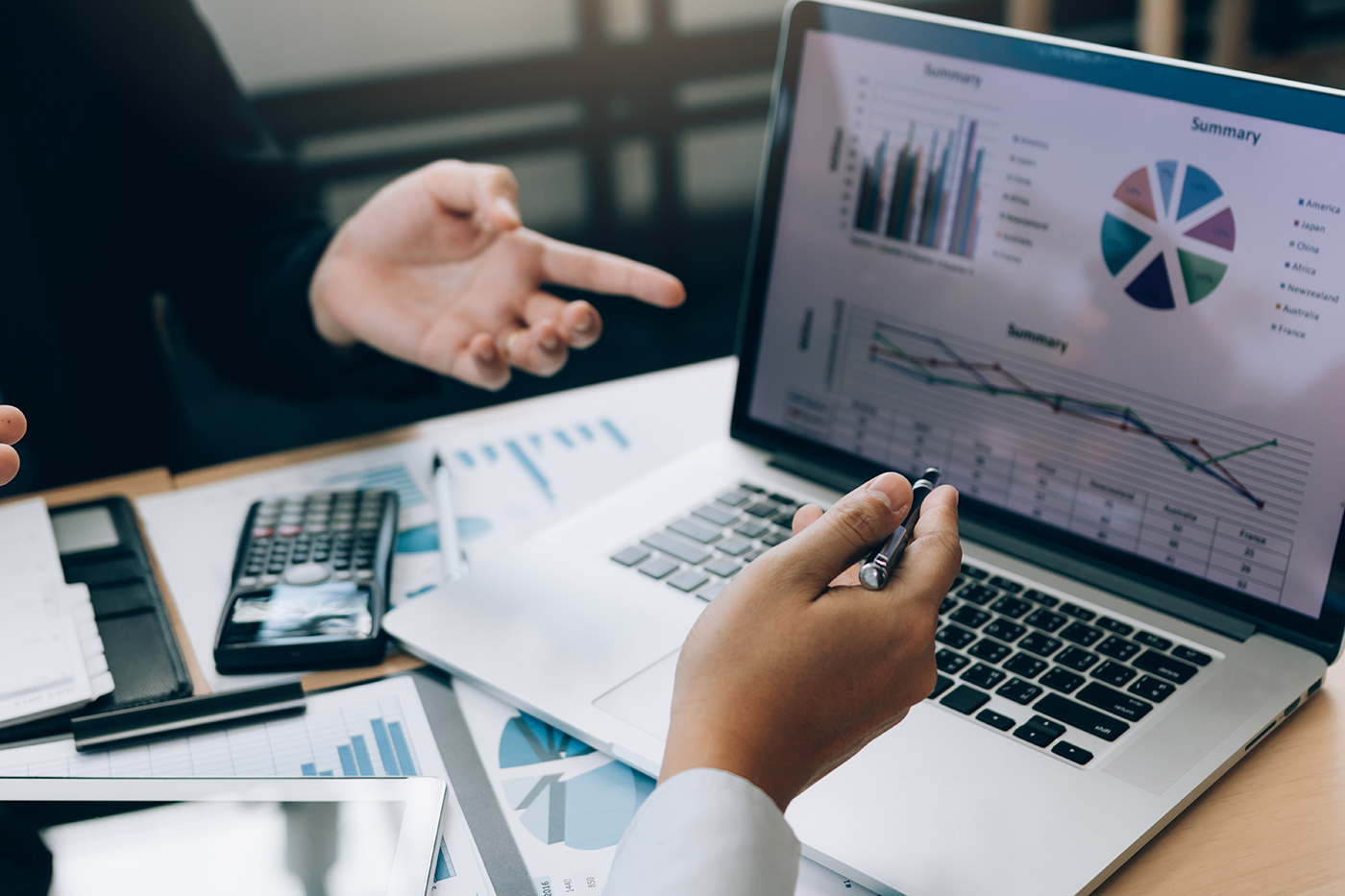
Forecasting is a key tool used in stress tests and in projections for expected credit losses. The length of a perfect forecast horizon, however, has always been a source of contention in business.
Moreover, with the growth of climate change research and regulation – which calls for institutions to stress test for the next 30 to 50 years in some jurisdictions – the debate has come to the forefront again.
Deniz Tudor
So, this forecasting time-horizon issue is worth revisiting, especially after the emergence of COVID-19, which ultimately required many financial institutions to rethink their pre-pandemic models and go back to the drawing board.
Before diving into the forecasting impact of COVID-19, we need to first understand how we arrived at where we are today. That begins with taking a look at the stress tests and financial accounting standards that were put in place in the aftermath of the Great Recession.
On the one hand, stress-testing regulations, like the Comprehensive Capital Analysis and Review (CCAR) and the complementary Dodd-Frank Act Stress Tests (DFAST), require institutions of a certain size to make nine-quarter forecasts for capital planning purposes. On the other hand, more recent accounting standards, such as the Financial Accounting Standard Board’s (FASB) Current Expected Credit Losses (CECL), expect institutions to forecast credit losses for the lifetime of loans, with the help of a concept called reasonable-and-supportable (R&S) periods.
For financial institutions with longer-term maturities (such as mortgages), the R&S approach made sense. A financial institution with a portfolio of mortgages with 30-year horizons shouldn’t be expected to predict that far in advance, and should be permitted to take, if you will, a shortcut (i.e., R&S) to make longer-term predictions.
Under FASB’s CECL rules, financial institutions need to use mean reversion – a well-known concept for asset-price volatility and historical returns – to leverage this shortcut. After much debate, FASB determined that financial institutions could mean revert (to meet CECL’s R&S requirement) using either an input or output approach; the former referred to reversion of input variables of loss-forecasting models, while the latter referred to reversion of the loss forecasts to some historical mean.
Although there were still some arguments about the merits of forecasting via a mean reversion to a historical mean (particularly for portfolios with a short history), it seemed as if all debates were settled by the time institutions had to comply with CECL in the beginning of 2020. That is, until March 2020, when COVID-19 hit, throwing the modeling world into self-preservation.
COVID-19: Impact and Lessons Learned
During the first month of the COVID-19 outbreak, macroeconomic forecasts were altered several times to meet the new realities of the world – a world with shutdowns, unemployment and tumbling GDP. In retrospect, almost no one saw this 1-in-a-100-year tail event coming; moreover, no one expected the pandemic to last long, and any forecasts released at the time had significant shortcomings.
By April or May of 2020, most businesses realized any short-term forecasts coming out of pre-pandemic models – particularly those that were designed to predict medium- or long-term horizons – were going to be difficult to use without significant overlays. Consequently, that was the approach everyone took, via complementing their pre-pandemic models with internal forecasts designed especially for the short term, often depending on roll rates that aligned more with business expectations.
As time passed and policy interventions kicked in, businesses also realized they had to do negative overlays for models that were designed to be sensitive to the economy (such as CCAR-DFAST) and positive overlays for models that were designed to predict the long term.
One of the lessons we’ve learned during this pandemic is that it does not matter whether models have good intentions. In times of crisis, risk modelers must predict the short term, and predict it accurately, so that they can survive in the long term.
In some circles, this approach of customizing models to improve short-term accuracy has been disparaged – especially by those who criticize corporations for chasing short-term profits instead of long-term profits. However, from a forecasting perspective, the long term is, in fact, conditional on the short term.
Where you start, in other words, matters. In business, this approach is similar to planning for liquidity versus capital. In nontraditional econometrics, it matters even more, in terms of priors and hyperparameters.
Financial institutions that adjusted their models, via focusing on short-term accuracy enhancements, were able to decrease the errors between forecasted losses and actual losses. But this strategy wasn’t well received by those that expect models to perform well under all conditions.
Parting Thoughts
Improving the short-term accuracy of models was, indeed, not a panacea, because it created other issues (such as governance of overlays) for business practitioners. Lamentably, although this approach helps businesses make better decisions, it does not necessarily align with the theoretical expectations of non-practitioners. More specifically, it is hard for those not running a business to understand why one would need to use overlays for the short-term, depending solely on business acumen, in crisis times.
As mentioned earlier, FASB expects businesses to predict perfectly well within an R&S period – using models, initially, followed by mean reversion. But forecasting length has been heavily debated in the industry.
Before the pandemic, some thought an R&S period couldn’t be longer than a business cycle. Some said it depended on the length of the history of the portfolio, while others claimed it should be the nine quarters intended for CCAR-DFAST. Meanwhile, others argued that it depended on the management’s outlook, with some institutions sticking to one-year or shorter horizons.
However, when one is searching for period in history resembling a pandemic period, a short R&S window is challenging. Since it requires a reversion to the historical mean, such an approach is also problematic for companies whose portfolio composition has changed significantly over the years.
Beyond the expectations of a perfect prediction within an R&S period, and a reversion afterward, FASB also expects the R&S period to be assessed every quarter and adjusted, if necessary. One would think, due to increased uncertainty, the R&S for CECL should be shortened during crisis periods. But the question then is, what use is shorter R&S for a model developer who has to mean-revert to a historical mean, if that requires him or her to use data from periods with no similar crisis?
In contrast to CECL, CCAR-DFAST assumes that nine quarters are perfectly R&S, no matter what’s happening in the markets. (To help institutions with these forecasts, the Federal Reserve annually releases 13-quarter projections of more than 20 U.S. and international macroeconomic variables under baseline and stress scenarios.)
But business practitioners understand that there is no infallible time horizon for forecasting. Whether we’re talking short term or long term, predictions must regularly be adjusted to account for current economic circumstances.
Sometimes, though, existing models can only be trusted after adjustments for the short-term. This rings especially true during times of crisis, when CCAR-DFAST and CECL models, by design, fail and require overlays.
Deniz Tudor works in the banking industry as a lead model developer and risk strategist. She specializes in econometrics, statistics, and enterprise risk management, and can be reached at denizktudor@gmail.com.