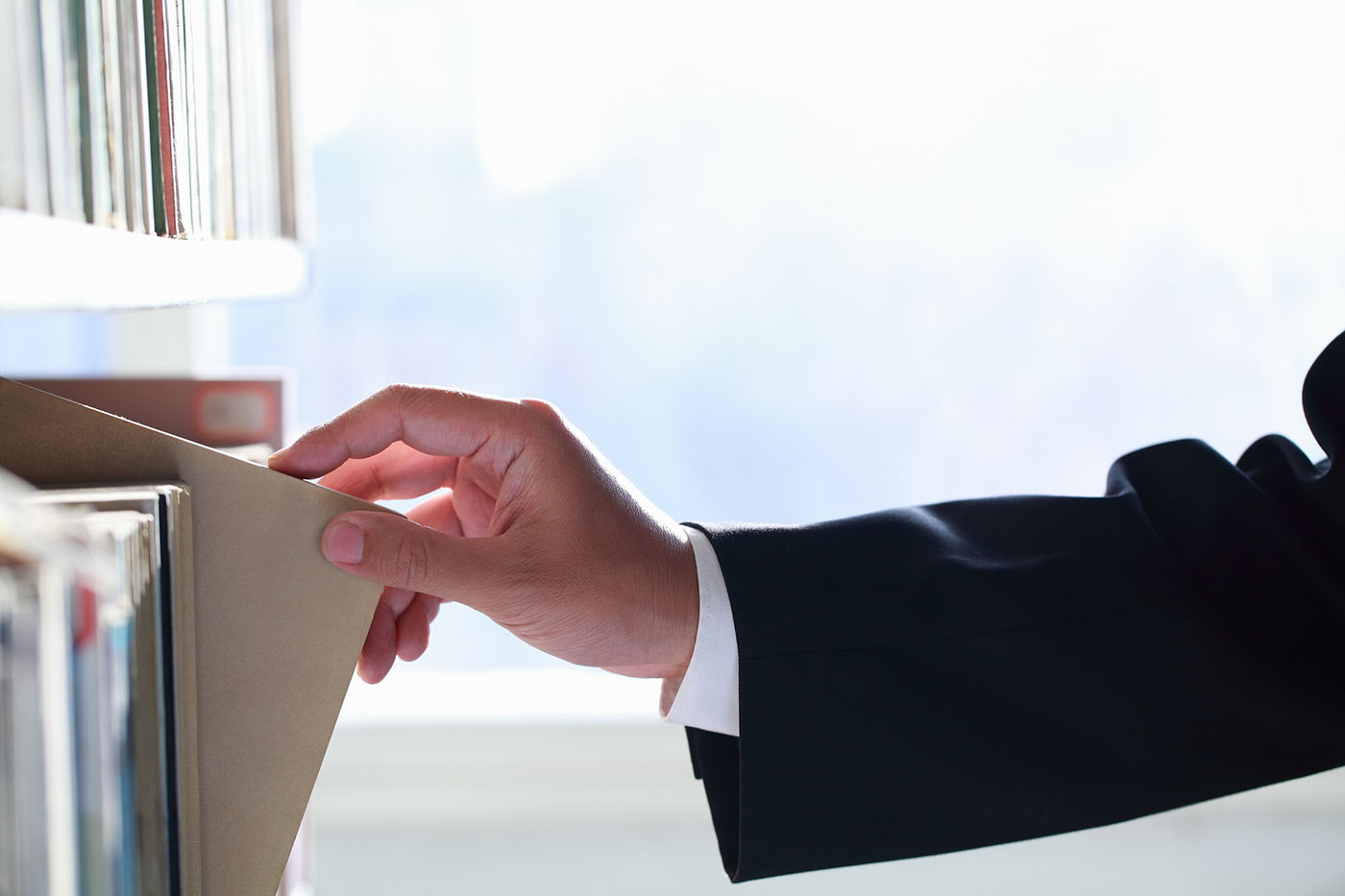
In the post-COVID era, in the midst of a mini-banking crisis, we’re at something of a crossroads in modeling and model risk management. Figuring out a way to improve financial risk modeling, which remains restricted by stringent regulations on model documentation and validation, is imperative. But how can risk modelers go about moving the needle?
Well, for starters, we may be able to learn some valuable lessons from academics’ rigorous approach to modeling.
When I moved from academia to the finance industry many moons ago, I was taken aside by a number of shocked professors and warned that my research skills would atrophy. The perception – whether deserved or otherwise – was that work in industry was sloppy, unscientific and too rarely interested in cutting-edge techniques. Put simply, the viewpoint of academics was that the work of the professors was rigorous and pure, while industry practitioners were just going through the motions.
In my subsequent travels, I’ve often reflected on what the industry misses by not taking a more academic approach. Generally speaking, modeling in the financial services industry really is less rigorous than it is at universities.
Tony Hughes
Some of the reasons for this are banal – for example, the range of topics covered by financial risk modelers is much narrower and the data sets being used are more uniform in their constitution. Moreover, in some cases (the modeling of loss given default for wholesale portfolios springs easily to mind), the sparseness of the data means the modeler will be scrambling for something that works, regardless of their academic credentials.
Indeed, for a lot of practical problems in finance, the classic three-variable regression really is the best available tool.
There are, however, pockets of financial risk modeling that would warm the heart of the average academic. In situations where regulations are light and research staff are asked for models geared for maximum profitability, risk analysts are often allowed to express their talent in the fullest possible sense. (This is particularly true at banks that have successfully implemented modern machine-learning techniques.)
Where the data constraint, moreover, is less binding (modeling mortgage default probabilities would be a good example), financial risk modelers can flex their intellectual muscles much more than they normally do. Of course, if you handed the available data for mortgage default probabilities to a talented academic, he or she would return pretty quickly with some cool models providing new insights into the behavior of mortgage borrowers. Whether these models would pass the standard validation process, though, is another matter altogether.
Regulatory Obstacles: Restrictive Rules
Why would cool, cutting-edge models not pass validation? I feel a digression coming on.
Like many others, I dove into online chess during the pandemic. There are innumerable “rules” for determining whether a particular opening is sound: e.g., don’t move the same piece twice, develop knights before bishops, and recapture pawns toward the center. The difference between a Grandmaster (GM) and a normal player is that the GM will routinely break the rules, but do so in a way that’s invariably advantageous to the mission of checkmating the opposition king.
With model validators and regulators, though, the rules are the rules and they can’t ever be broken. A standard set of diagnostics are typically applied to the finished model, and even the coolest model in the world cannot be used if it fails the most redundant of tests.
To cite an example close to my heart, I cannot begin to count the number of times my models have been dissed for excessive multicollinearity. This is despite the fact that multicollinearity is largely irrelevant for forecasting and demonstrably advantageous in a stress testing context.
Model builders, of course, are aware of the rules, so they tend to stick to the script and keep things nice and vanilla. Industry models will generally be built at the level of a good club player, but, for Grandmaster-level work, you need to look in academic journals.
There are various other incentives and logistical constraints that push the industry toward mediocrity. The inevitability of management overlay, for example, saps the lifeblood of the modeler: why pour your heart into a model if its findings are likely to be overruled by a committee? Similarly, scenario analysis – a staple of modern risk management – does little to promote modeling excellence, because the quality of the analyses cannot be objectively judged.
Model Envy
While an academic may consider hundreds of different formulations when developing a new paper, a bank will have one or two models in place for a specific purpose – as well as rigid rules governing the circumstances and timing of replacements.
An academic can produce an interesting new thought during the morning shower and have new models ready to explore by lunchtime. The professor does not need to produce a zillion-page document for every model they use. Indeed, a short academic paper may rely on the results of many models, all making a specific point.
Contrastingly, at a bank, the notion of having 20 different mortgage PD models, all exploring a different nuance, is completely ridiculous from a practical viewpoint.
I think this is the main reason for the lack of rigor in industry. The marginal cost of using an extra model is close to zero for the academic, but highly significant for the bank CRO. The ability to experiment with the modeling process and to judiciously break the rules – the tactics that lead to GM-level insight – are thus generally lacking in financial risk modeling.
The cost of this relative lack of rigor is that certain risks get missed.
I think a free-wheeling, academic-style research program, driven by scientific curiosity rather than regulatory requirements, would have a better chance of identifying idiosyncratic risks faced by the banks. However, it would also be easy for the banks to fake such a model development process, and it would be difficult or impossible to regulate.
So, then, what can be done to improve risk modeling at financial institutions?
Parting Thoughts
A loosening of some of the rules and conventions of the model-building process could have a positive marginal effect. For example, today’s model documentation process is unwieldy and could be greatly simplified, without losing anything critical.
The focus of the validation process, meanwhile, could shift to a broad assessment of the overall modeling process, as opposed to a singular focus on specific models. In modeling mortgage PDs, for example, a risk team could use this approach to demonstrate the journey they took to reach the preferred specification, informing management of lessons learned along the way.
It now seems as good a time as any to question the modeling culture that emerged in the aftermath of the global financial crisis. Small moves in the direction of rigor and excellence would be positive, and may help to rekindle financial risk modeling.
Tony Hughes is an expert risk modeler. He has more than 20 years of experience as a senior risk professional in North America, Europe and Australia, specializing in model risk management, model build/validation and quantitative climate risk solutions.
Topics: Modeling