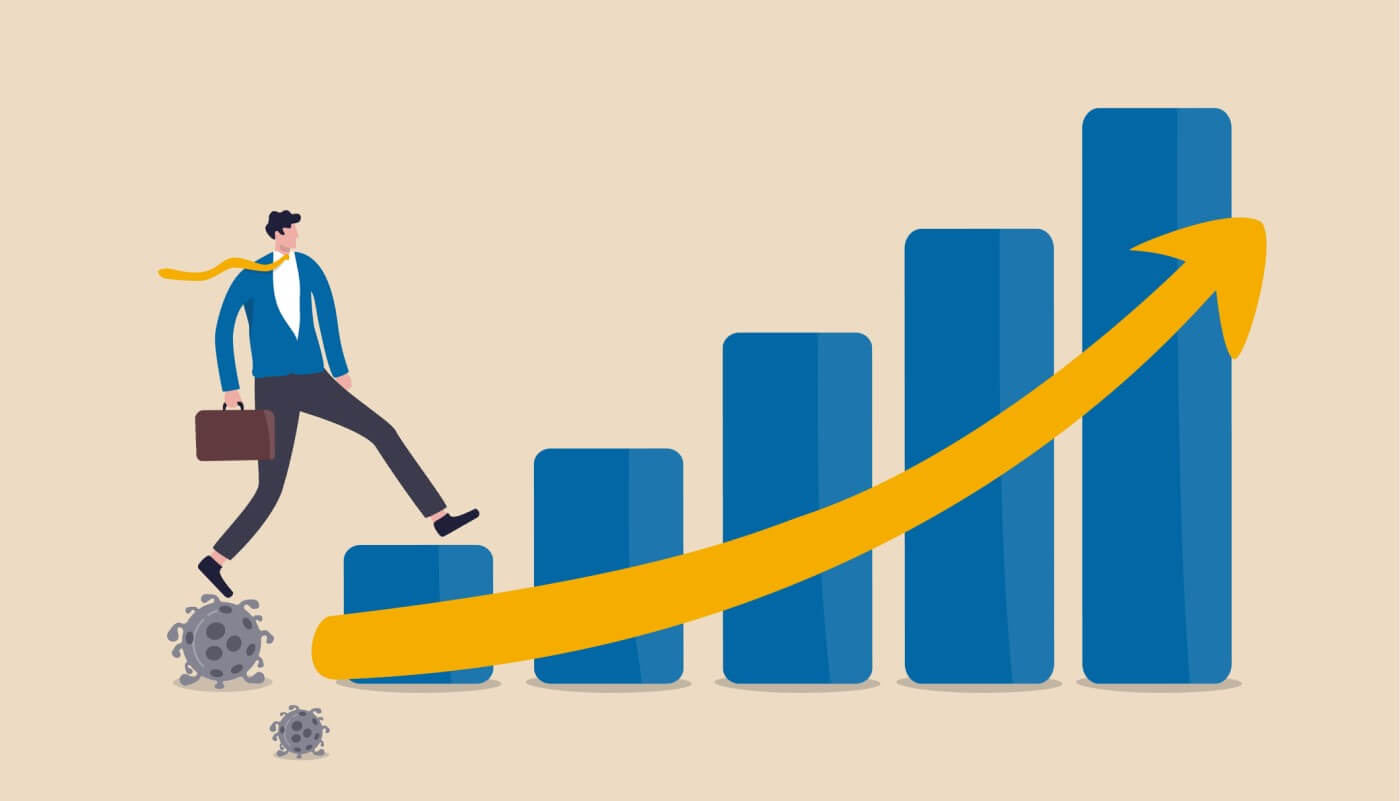
Now more than ever, with COVID-19 rendering historical data mostly irrelevant for loss forecasting, estimation of expected credit losses must be forward looking. This presents significant challenges for modelers, analysts, senior management and other key stakeholders within financial institutions.
Teams that develop credit loss models and analysts in risk and finance groups must come up with defensible, integrated models - all the while managing scarce data and developing a robust and repeatable process. Moreover, they need to be able to integrate new data and adjust their models in a timely fashion.
Senior management, meanwhile, has to develop proactive contingency plans and adjust credit limits dynamically, based on early warning signals provided by these models. And these actions must be transparent and explainable, so they can be approved by boards of directors, verified by auditors and understood by investors.
Considering the complexity of these challenges, combined with the credit risk uncertainty fueled by the pandemic, it should come as no great surprise that FASB's Current Expected Credit Losses (CECL) accounting standard has already been delayed three times.
The CECL deadline for smaller reporting companies (SRCs) was extended from 2021 to 2023 in October 2019. Last year, via the pandemic-driven CARES Act, the deadline for large firms (including bank holding companies) was pushed from January 2020 to Dec. 31, 2020, or the end of the pandemic - whichever came first. Finally, on December 27, 2020, the U.S. Consolidated Appropriations Act extended the aforementioned deadline for large banks to the earliest of Dec. 31, 2022, or the “end of the national emergency declaration related to the COVID-19 crisis.”
One key question now is whether banks should attempt to adopt CECL early or simply take advantage of the CECL leeway granted by regulators. I think the smartest banks - even those on the smaller side of the CECL compliance spectrum - will choose the former.
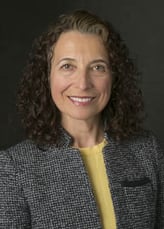
Forward-looking estimation of potential credit losses - through-the-cycle - represents a great opportunity for banks to overcome many of the critical issues they face with their financial planning, capital allocation and risk modeling.
Banks that strive to become early adopters, of course, will have to overcome some significant data and strategy challenges. But before we have an in-depth discussion on CECL challenges and potential solutions, it's important to consider how we got to this point.
Up until 2020, there were many distinctions between big and small banks in terms of implementing CECL. Different CECL modeling approaches were suggested for banks of varying sizes, depending largely on the amount of data an institution had at its disposal. But the advantages held by larger banks that were more data-driven were obliterated by COVID-19, almost as quickly as the virus has spread.
Before the pandemic, to meet CECL's reporting guidelines, banks relied on a mix of historical data, current data and forward-looking projections. But the uniqueness of the COVID-19, with respect to everything from surging unemployment to falling GDP to government-support programs that muddied default projections, meant that banks in 2020 had almost no useful historical data with which to work.
Indeed, since they can no longer provide robust loss projections, CECL methods that rely heavily on historical performance have become irrelevant - at least for a while.
Now, with forward-looking projections so crucial to CECL compliance, banks need to figure out how to hurdle data obstacles while improving the accuracy of their forward-looking scenarios.
Overcoming CECL Challenges
The first big CECL challenge is, of course, the data. Banks may not have enough historical data on retail and commercial loan portfolios to assess future losses on the projected scenarios.
Pooling the data into uniform risk and behavioral segments - including by geography, product type and market segment - has always been one way to deal with the problem. Today, however, even perfect historical data may be irrelevant, so what's the best path forward?
I suggest a two-part solution: the first involves better segmentation of historical data (supplemented by machine learning), and the second calls for changes in the list of variables we project in our scenarios.
Retail loans are typically broken down by market segments and product types, but can be further clustered according to their delinquency status. Reliable aggregated data of this nature - which may fill in the gaps in an institution's own historical data - can be obtained from the Federal Financial Institutions Examination Council (FFIEC).
However, as was demonstrated during COVID-19, even historical time series as reliable as these can't be directly regressed on macroeconomic and market variables to forecast behavioral patterns. Instead, we can use explainable machine-learning techniques to learn from the outliers in the available history, and to project delinquency variables in a much more reliable fashion.
Our second suggested change - regarding the careful selection of scenario variables - is relevant for commercial real estate (CRE) and commercial and industrial (C&I) loans. Expected credit losses for such loans are traditionally measured as the product of probability of default (PD), loss-given-default (LGD) and exposure-at-default (EAD). But one cannot adequately capture all of these metrics through direct projections. It is therefore also necessary for banks to consider local variables (relevant to their geographic HQ) in their CECL scenarios.
Complications in Default Estimations, and the Need for Better Scenarios
Keep in mind that the dynamics of PD are driven by the entire rating transition matrix. So, PD could be impacted, for example, by increased credit spreads that lead to more potential downgrades.
LGDs, moreover, are intricately connected with the collaterals of their respective loans. Such collaterals - or their representative benchmarks - must be modeled on the scenarios.
EADs, meanwhile, are mainly relevant for revolving loans and credit lines, and are dependent on how much of unfunded commitments can be drawn on projected scenarios.
Complicating matters further, in the stress situation that surrounds us right now, it is possible that PDs, LGDs and EADs might all increase at the same time - even under the baseline scenario appropriate for the current environment.
So, circling back to our proposed solution, we must consider the following questions: what variables should be included in our scenarios, and how they should be quantified? Obviously, all variables that are relevant to projecting delinquencies for retail portfolios, as well as PDs, LGDs and EADs for C&I portfolios, should be included in the scenarios. Typically, these include some major macroeconomic variables, like the ones that make up the Federal Reserve's CCAR scenarios.
But it's important to also include local variables. If your business is based in either New York, New Jersey or Connecticut, for example, you can weave house price indexes, the unemployment rate and income growth for the tri-state area into your scenarios. The historical time series of these variables are available via both the FFIEEC and the Federal Reserve Economic Database (FRED).
To assign such values to the variables on the scenarios, banks generally combine quantitative models and expert judgement. While the preferred ratio of the former to the latter is supposed to be around 80/20, the events of 2020 - which disqualified many credit loss models - have skewed this ratio toward managerial overlays.
This over-reliance on managerial overlays makes it difficult for large banks, and practically impossible for smaller ones, to establish and maintain robust and repeatable CECL processes with the proper governance and validation that passes muster with regulators and auditors.
Banks can get around this problem by considering a multitude of scenarios. While CECL requires a baseline scenario that should reflect reasonable and supportable forecast horizons, it's also good practice to incorporate sensitivity analysis by considering a few more scenarios - most commonly around the 25th and 75th percentiles of loss distribution.
The most reliable way to design such scenarios is to extract them from the full distribution of credit losses, with empirical estimation of percentiles and reverse selection of scenarios leading to the respective outcomes.
Advantages of Early Adoption of CECL
The methodology described above turns the dilemma of early adoption into a no-brainer. Indeed, financial institutions that do not have to comply with CECL until 2023 would be wise to weigh the advantages of starting now against the difficulty of data preparation and scenario design.
All of the data they'd need to get started is available through FRED and FFIEC sources, and the new scenarios we've suggested can be automatically extracted from the full loss distribution. This means the only additional step early CECL adopters will need to take is to adjust their loss-forecasting results based on aggregated state data. (For banks that do not have reliable internal data, this is particularly important.) Banks that prioritize internal data gathering and perform sensitivity analyses today will be perfectly set up to file early CECL reports.
Besides accounting benefits, this multi-pronged approach to CECL will help develop common underlying processes (systems, tools and data), ensuring consistency across the entire balance sheet with respect to financial planning, stress testing and the calculation of capital and liquidity ratios.
Parting Thoughts
CECL provides a strong incentive for financial institutions to step up in the process of holistically managing their organizations. The achievable goal should be fully integrated governance and transparent communication between businesses, risk management, board of directors, investors and regulators.
In the current environment of extreme uncertainty, the only way to analyze risk and properly plan for the future is through forward-looking analysis informed by probabilistic outcomes on dynamically-projected scenarios.
Alla Gil is co-founder and CEO of Straterix, which provides unique scenario tools for strategic planning and risk management. Prior to forming Straterix, Gil was the global head of Strategic Advisory at Goldman Sachs, Citigroup and Nomura, where she advised financial institutions and corporations on stress testing, economic capital, ALM, long-term risk projections and optimal capital allocation.