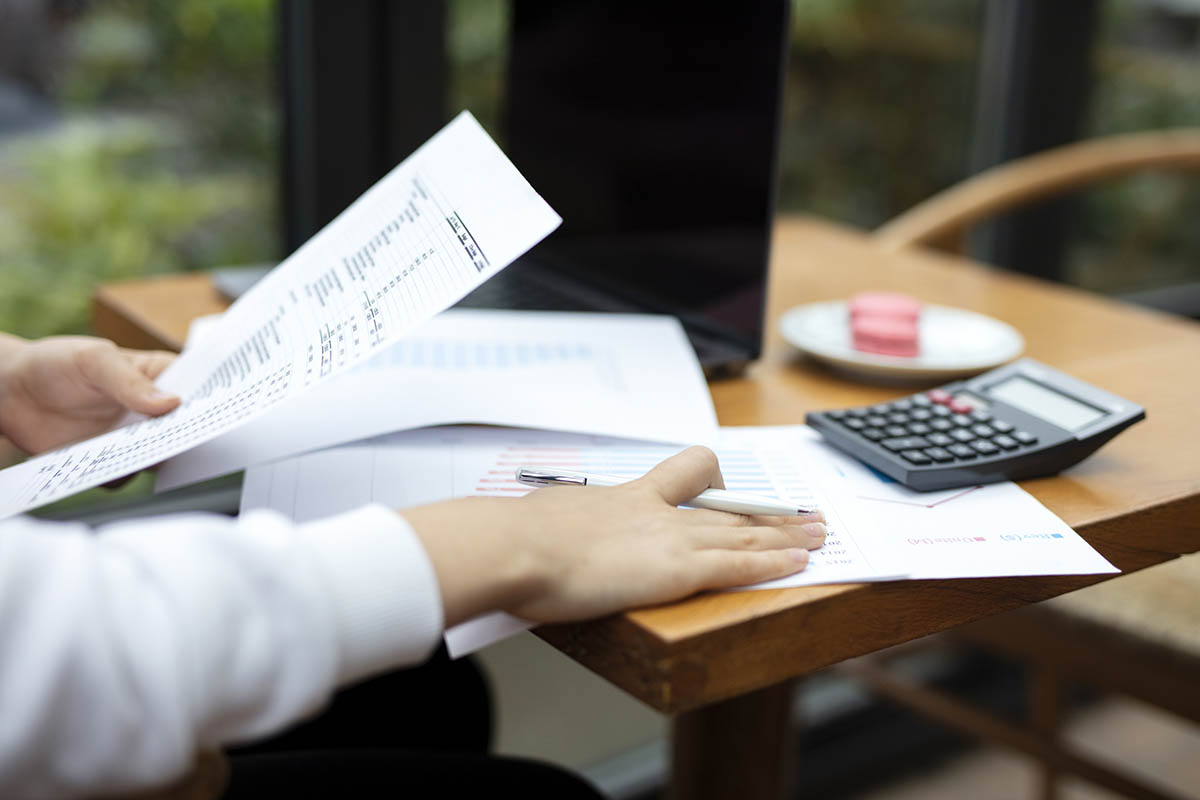
Financial institutions annually go through a budget allocation process, with business heads competing for capital and other resources. They give their projections on how much capital they need and how much revenue they expect to generate with it.
The problem, however, is that the projections are typically based on a combination of current market trends and the historical performance of a firm's business lines, which leaves plenty of room for error.
Alla Gil
Under this approach, when an economic environment substantially deviates from history, the financial revenue projections for a business line are not realized. Established goals are typically then revised – but this retrospective strategy also suffers from serious flaws.
Firstly, this reactive approach to budget planning lacks agility and forward vision, and doesn’t help with optimal allocation of capital. Secondly, it doesn’t enable full estimation of potential future risks and their hidden concentration pockets. Lastly, it doesn’t allow for establishing early warning indicators and respective contingency plans, which are critical for surviving various shocks.
What’s more, the flaws of reactive financial planning might negatively impact a firm’s earnings, as well as the reports of its investment analysts. It is therefore necessary for financial institutions to develop planning approaches that are proactive, reliable and robust. But how, exactly, can they meet this goal?
Optimal Capital Planning
Better planning can be achieved through enhancing governance structures and increasing CEO engagement. Everyone involved in the planning process should also be eager to use modern data analysis to support agile decision-making and optimal resource allocation, even amid unprecedented market conditions. Proactive planning, moreover, must be transparent and allow for the incorporation of expert judgement at every step, because strategic decisions can’t be driven strictly by black-box models.
For capital allocation forecasts, the focus is often on enhancing the accuracy and reliability of predictions. But this is an impractical and nearly an impossible task that sometimes leads to drastic changes – not only in the forecasts themselves but also with respect to a firm’s strategic direction.
Rather than pinning their hopes to unrealistic accuracy expectations, firms that want to improve planning should strive for a comprehensive understanding of potential outcomes for a full range of scenarios. This will allow them to (1) identify potential future risks and prepare proactive adjustments to the capital allocation plan; (2) incorporate the economic outlook and link performance to macro and market factors; and (3) develop early warnings that are dynamically tailored to the allocation of the budget.
Achieving these benefits requires time series analysis to identify behavioral patterns and scenario generation and analysis to discover hidden risk concentrations. Sophisticated artificial intelligence (AI) and machine-learning (ML) techniques can be used to perform these steps and to support decision making.
Traditionally, forecasting entails developing three to five scenarios (including the baseline, and a couple of best-case and worst-case ones) – but that does not account either for rapidly changing market conditions or for full estimation of potential risks. Using multiple forecasting methods or frequent updates doesn’t help, because those approaches still rely on historical data and the viewpoints of modelers, which tend to be biased by their experiences.
It’s also important to keep in mind that tail risk is the key contributor to failed forecasts and poor capital allocation decisions. That’s why producing multiple scenarios via Monte Carlo is also not a panacea, because such simulations use constant covariances and aren’t capable of realistic assessment of tail risk probabilities.
This is not, however, an unsolvable dilemma. By combining best practices for prognosticating with full-range scenario generation, firms can leverage the advantages of forecasting and avoid the flaws associated with this methodology.
Full-Range Scenario Analysis and Machine Learning
Forecasting through full-range scenario generation allows risk managers to assign probabilities to potential outcomes. This ensures that management doesn’t get distracted by tail risk with low probability. Instead, managers can receive early warnings whenever the probability of an adverse outcome increases – triggering contingency plans, whenever necessary.
This full-scenario approach is particularly effective when combined with ML techniques that learn from data proxies, such as behavioral responses to observed regulatory, market or other shocks. Indeed, ML models tend to do better with forecasting and with managing uncertainty than standard regression techniques.
ML techniques overcome human biases in selecting risk drivers by suggesting their own drivers, which can then be tested by modelers. When combined with sensitivity analysis overseen by modeling experts, ML can reduce dimensionality and simplify the financial and capital planning processes.
Exhaustive reverse scenario analysis can be performed through additional ML techniques, like cluster analysis of the generated synthetic data for, say, thousands of forecasts. Through this approach, firms can identify early warning indicators, and then adjust their budget allocations accordingly.
Management can also consider and overlay other potential actions (like changes in pricing, promotions, and product mix), and subsequently assess the impact of such actions on the risk/return profile (including cashflow projections) for each business.
A Practical Example
Let’s consider an illustrative example of a bank with six business lines (BLs). Figure 1 depicts the percent change in their respective historical monthly time series data.
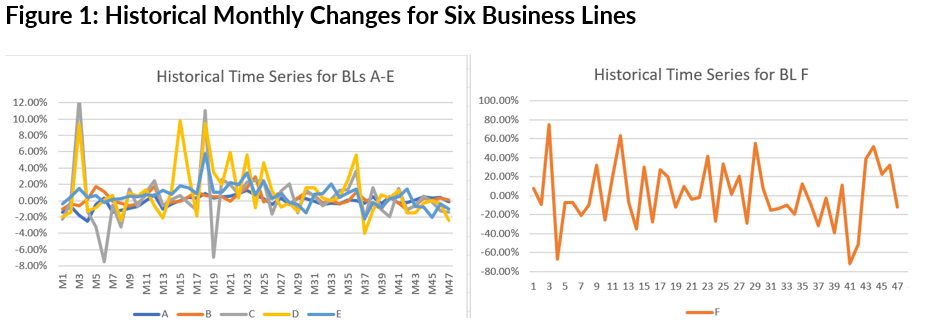
From the charts in Figure 1, we can see that business line F is order of magnitude more volatile than the other five business lines (BLs A-E). Even though it looks like investing more in this business line might generate more return, this is not the case (see Figure 2, below).
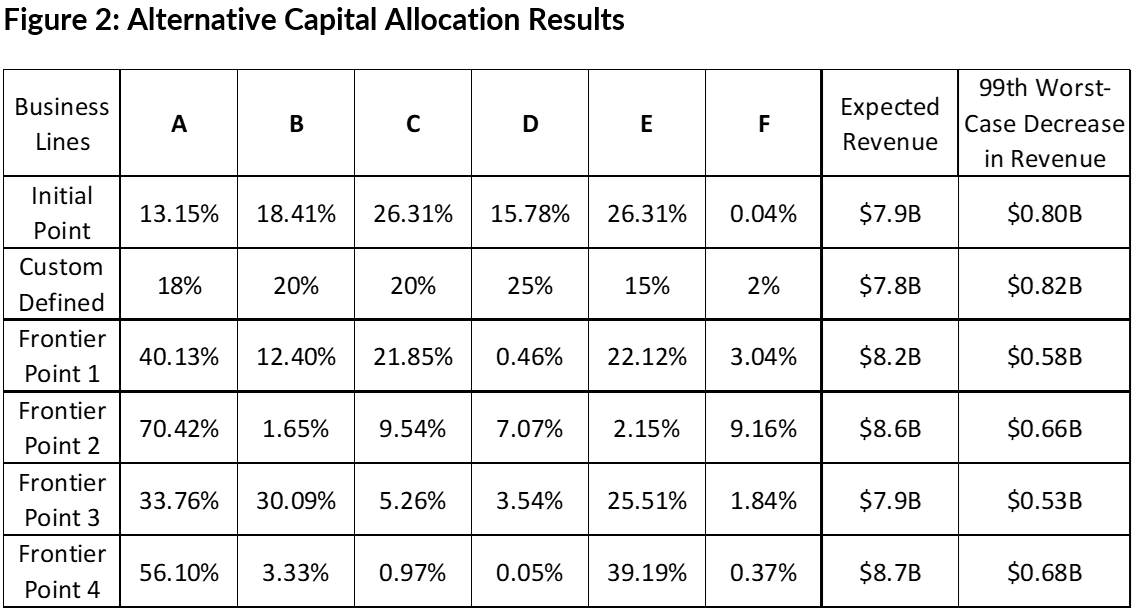
In the example above, the strategic objective is the total expected revenue over the three-year forecast horizon - from all the businesses, based on the given budget allocation. The expectation was calculated from more than 1,000 generated forecasts. The risk in this analysis was measured as the 99th-percentile worst-case decrease in revenue – i.e., the difference between the expected value and the 99th worst-case outcome.
The table in Figure 2, as well as the chart in Figure 3 (see below), show the results of running hundreds of various capital allocation strategies.
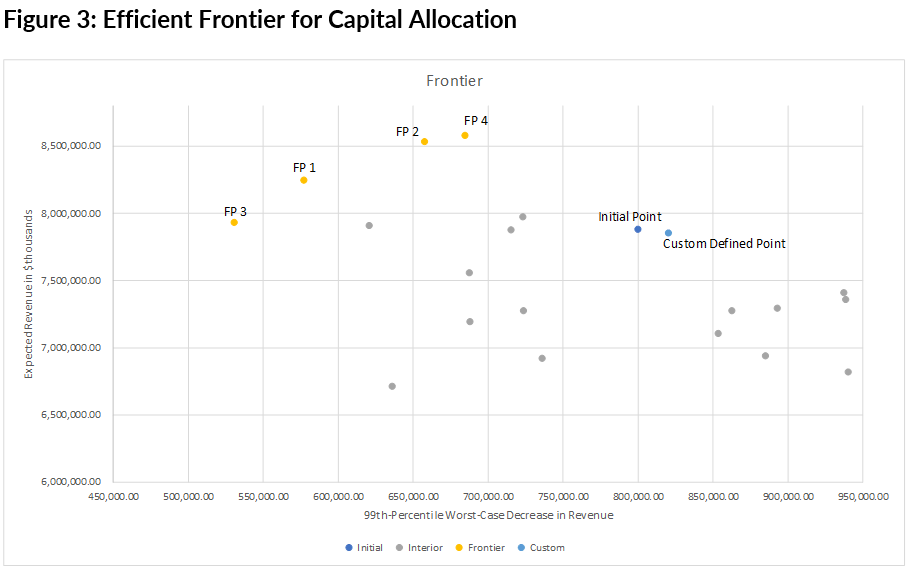
The four highlighted points in Figure 3 (FP1, FP2, FP3 and FP4) represent the efficient frontier. Their optimal capital allocations across business lines are given in Figure 2.
All four optimal points in Figure 3 recommend reducing the allocation to business lines C and D, while increasing investment in business line A. Most managers would find that the allocation in frontier point 2 (FP2) is too concentrated in business line A, and would therefore select either FP1 or FP3 – the frontier points with the lease deviation from the current budget allocation.
Planning departments can analyze and challenge capital allocation requests through this comprehensive scenario analysis approach, which allows for rapid adaptation to changing circumstances and continuous evaluation of strategic alternatives.
Parting Thoughts
For executives in charge of budget allocations, many benefits can be derived from full-range scenario analysis – including improved confidence in projections, enhanced agility and resilience, and better use of advanced technology. Firms that instead rely on bottom-up, manually-developed scenarios could miss the combination of risk drivers that are specifically relevant for a particular objective.
Only full-range scenario analysis enables senior management to make proactive, informed decisions with a complete understanding of the risks, opportunities and ripple effects of unprecedented shocks.
Exhaustive reverse scenario analysis, moreover, makes it possible to develop contingency plans based on early warning signals – and to effectively communicate with boards, regulators and investors why a particular plan is selected and under what circumstances modifications can be triggered.
Effective decision-making should be based on actionable insights, rather than complex analyses. Through data-driven, full-range, scenario-based forecasting, firms can evaluate the realistic probabilities of tail risks, substantially improving the robustness of their capital allocation and budget planning.
Alla Gil is co-founder and CEO of Straterix, which provides unique scenario tools for strategic planning and risk management. Prior to forming Straterix, Gil was the global head of Strategic Advisory at Goldman Sachs, Citigroup, and Nomura, where she advised financial institutions and corporations on stress testing, economic capital, ALM, long-term risk projections and optimal capital allocation.
Article Updated: May 20, 2024
Topics: Modeling