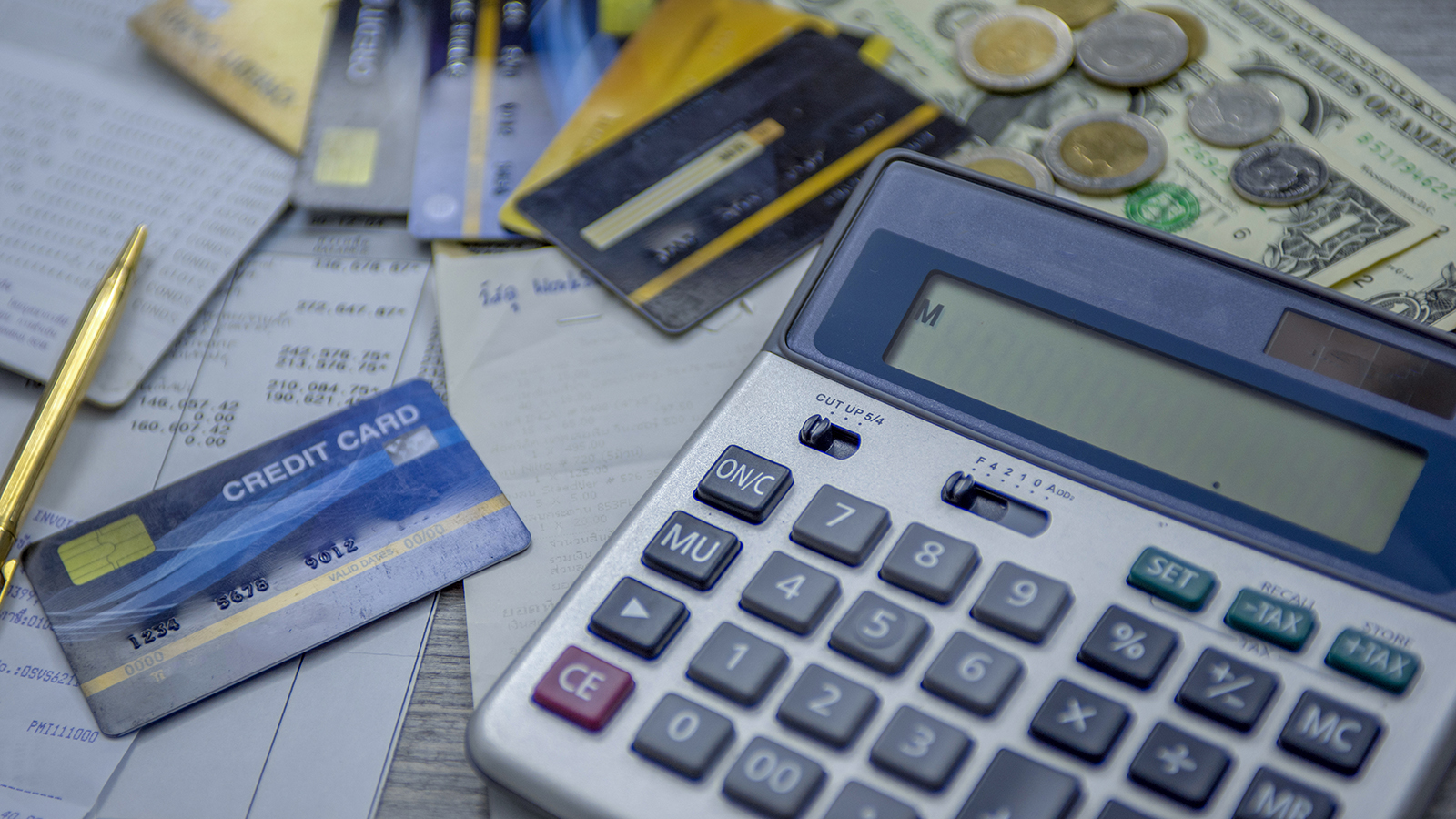
To what extent have rules developed in the aftermath of the Great Recession enhanced the reliability of credit loss forecasts?
This is a complex question. On the one hand, banks now have access to a vast amount of data about their borrowers, including their credit history, income, and debt levels. What’s more, they have sophisticated statistical models and access to powerful computing resources that can be used to analyze this data and predict the likelihood of default.
On the other hand, many factors can affect the accuracy of credit loss estimates, including internal data quality and the skill of risk modelers; the level of economic uncertainty; changes in regulation; and the development of new types of loans. The first factor is self-explanatory, while the others require a bit of context.
Cristian deRitis
The current economic environment can have a significant impact on credit losses. For example, a recession can lead to higher unemployment and lower incomes, which can make it more difficult for borrowers to repay their loans. The ability to predict credit losses may therefore depend heavily on the ability to predict the future economy.
Changes in regulations could limit underwriting to riskier segments, thereby reducing the volatility of losses. They could, moreover, create perverse lending incentives that credit models, trained on historical data, may fail to detect.
New loan products, meanwhile, could present obstacles, because banks may not have enough data to accurately estimate the default risk of these loans until they go through at least one business cycle.
Despite these challenges, over the past 15 years, lenders have made significant progress in improving their ability to forecast credit losses – thanks in part to the development of more innovative models and to enhancements in the collection of loan level performance data. Improvements in forecasting processes, such as the use of multiple economic scenarios, have also helped address economic uncertainty.
Learned Behavior
While banks cannot eliminate the uncertainty associated with credit losses, especially during periods of economic turmoil, they can now estimate losses more reliably than in the past. This is a key advancement, as it helps banks to manage their risks and ensure they have sufficient capital to absorb losses.
Looking back at the performance of credit loss forecasts over the past two decades, we can make three observations about loss estimates:
- Estimates are typically more accurate for portfolios of loans that are similar to each other, such as residential mortgages or credit card loans. For portfolios of loans that are more heterogeneous, such as commercial and industrial loans, forecasts are more difficult due to idiosyncratic factors.
- Estimates are more accurate during periods of economic stability. During periods of economic uncertainty, including the run up to recessions, it is more difficult for lenders to predict the likelihood of default or to judge what is a “reasonable and supportable” economic scenario.
- Estimates remain overly reliant on judgment, which makes them more susceptible to bias. Despite great improvements in the collection and modeling of quantitative data, banks still must use their judgment to assess the competitive environment, the creditworthiness of individual borrowers, and consumer psychology. This judgment can be influenced by several factors, such as a bank's culture, the experience of its loan officers, and external factors – such as media reports that may influence herd behavior.
Know Your Limitations
Overall, banks' credit loss estimates are a valuable tool for managing risk. However, it is important to be aware of the limitations of these estimates.
A 2023 study by the Bank for International Settlements (BIS) found that banks' credit loss forecasts were generally accurate in rank-ordering losses across institutions. However, it also determined that banks' forecasts were not especially accurate in predicting the evolution of credit losses over time.
Several factors – including bank profitability and macroeconomic conditions – could help to explain forecast errors. The study found, for example, that more profitable banks tended to be more optimistic in their loss forecasts.
Overall, the evidence suggests that banks can reliably estimate credit losses to a reasonable degree. But credit loss estimates are imperfect, and can be particularly inaccurate during periods of economic turmoil.
The Many Benefits, and Challenges, of Improved Loss Estimation
A pair of accounting standards for loss allowances – current expected credit losses (CECL) in the U.S. and IFRS 9 internationally – have stirred up significant debate for years. Implementation delays and final rule changes have added fuel to the fire. However, despite fears to the contrary, the historical record suggests that these rules have successfully met their objectives of improving the loss allowance process in several ways.
First, CECL and IFRS 9 have enhanced the timeliness of credit loss estimates. Under the previous incurred-loss approach, credit losses were only recognized when they occurred, which led to delayed recognition and understated losses. The new rules require financial institutions to instead estimate credit losses over the life of each loan, providing a more accurate picture of each institution's financial condition in real time.
Second, CECL and IFRS 9 have made financial institutions more resilient to economic shocks. By requiring the buildup of reserves for future losses, the rules help financial institutions absorb losses during downturns without having to scramble to raise capital or sell assets in a panic, making the financial system more stable.
Third, CECL and IFRS 9 have reduced the stigma associated with loan losses. In the past, financial institutions were often reluctant to recognize loan losses, because it could make them appear less profitable than their peers. By requiring potential losses to be recognized over the life of each loan, the stigma associated with loan losses is reduced, providing greater insight into firms’ financial conditions.
Fourth, CECL and IFRS 9 have led to improved risk management practices at financial institutions, including the development of more sophisticated credit loss models, improved data collection and analytical capabilities.
Fifth, CECL and IFRS 9 have improved transparency and comparability across the financial services industry. They make it easier for investors and regulators to compare the financial condition of institutions across time, leading to more informed investment decisions and more effective regulation.
Of course, even taking these benefits into account, the adoptions of CECL and IFRS 9 have not been without their share of challenges. The rules are, for example, more complex and costly to implement than the previous accounting model. In addition, they can lead to increased volatility in earnings, as credit loss estimates are subject to change based on economic conditions and other factors. (This has required investors and regulators to reset their expectations to avoid overreacting to quarterly shifts in the allowance.)
However, despite these challenges, the CECL allowance model is a significant improvement over the previous incurred-loss model. It has improved the accuracy of credit loss estimates, made financial institutions more resilient to economic shocks, and improved transparency and comparability.
Parting Thoughts
In the aftermath of the Great Recession, regulators across the globe introduced changes to capital stress tests and loss accounting rules designed to improve credit loss forecasting. Over the past 15 years, we have endured an extreme business cycle (including a pandemic, an oil price shock and several bank failures), and it’s safe to say we’ve seen significant advancements in credit loss estimation. But this remains an imperfect process, and there’s still lots of room for growth.
Moving forward, banks must invest in refining their credit loss estimation processes. This includes improving internal data quality and leveraging external industry benchmarks.
Firms need to develop more sophisticated risk models and enhance the skills of their risk modelers to keep up with shifts in credit markets. Moreover, they should continually monitor and adjust their credit risk models to account for changes in economic conditions and regulations.
Institutions should carefully consider the economic environment when making credit loss estimates. Given the volatility surrounding forecasts, lenders need to utilize multiple upside and downside economic scenarios to better control and prepare for macroeconomic uncertainty. If they don’t do this on their own, regulators and auditors will require them to do so.
Lastly, while credit loss estimates are a vital tool for managing risk, they are not a panacea. Risk modelers need to be humble and recognize that it will continue to be a huge challenge to forecast credit losses accurately more than a few quarters in advance.
Banks must therefore maintain robust capital buffers to absorb unexpected losses, rather than relying solely on their credit loss estimates for risk management. To ensure financial stability and resilience in the face of economic uncertainty, the goal should be to strike a balance between sophisticated credit loss estimation and prudent capital management.
Cristian deRitis is the Deputy Chief Economist at Moody's Analytics. As the head of model research and development, he specializes in the analysis of current and future economic conditions, consumer credit markets and housing. Before joining Moody's Analytics, he worked for Fannie Mae. In addition to his published research, Cristian is named on two U.S. patents for credit modeling techniques. Cristian is also a cohost on the popular Inside Economics Podcast. He can be reached at cristian.deritis@moodys.com.
Topics: Modeling