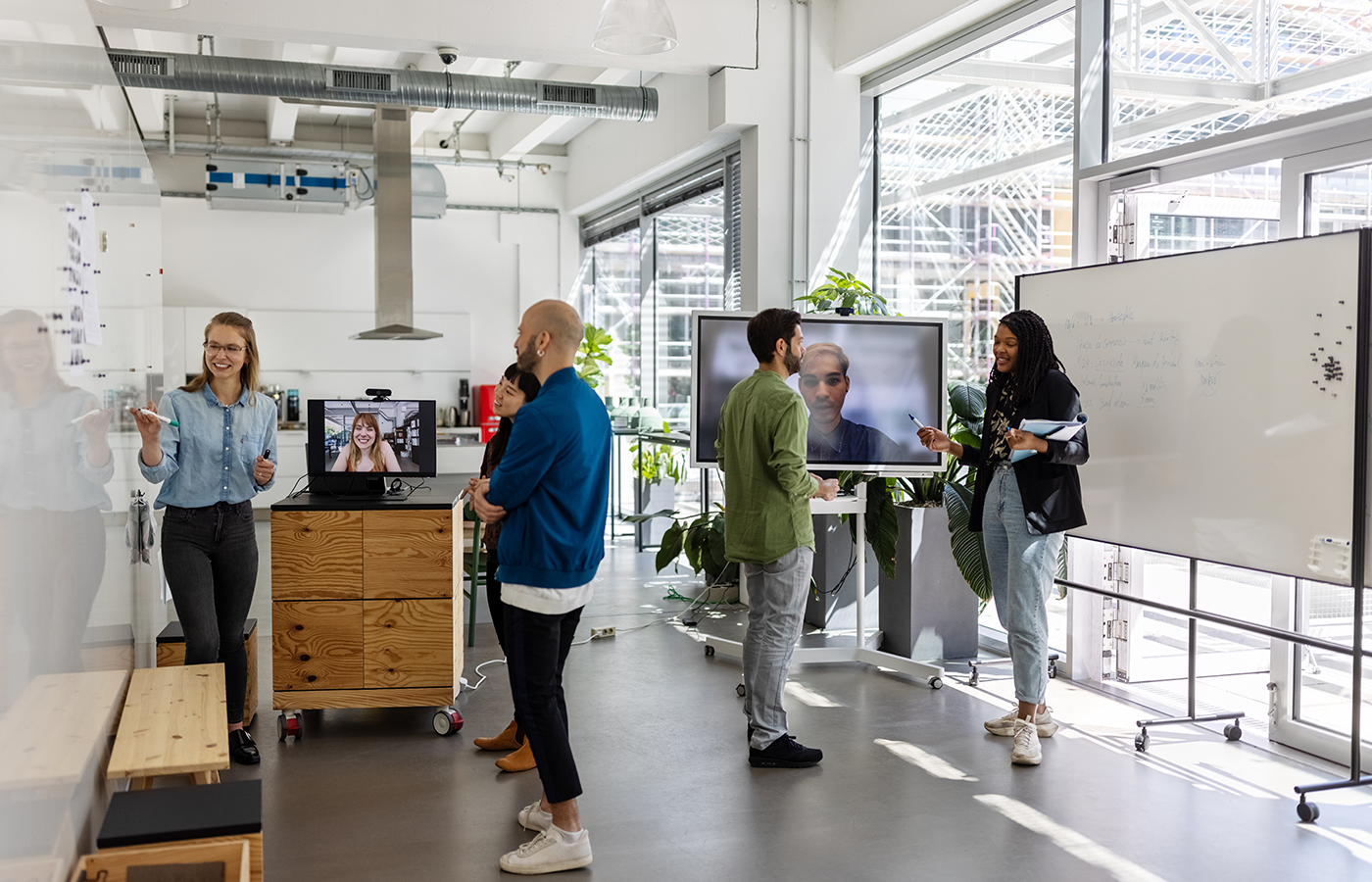
Credit risk models that are adaptable, flexible and fast, one could argue, are what’s needed in the current financial environment of uncertainty and volatility.
Banks need to be able to respond to market events quickly – and to reprioritize, when necessary. But what’s the best way to achieve this? Should banks stick with the traditional “waterfall” approach to model development or transition to so-called agile approaches?
Before we explore the pros and cons of the latter, let’s consider the components of the conventional waterfall methodology. The waterfall approach is a rigorous process consisting of several phases, including collection of model requirements; data gathering and preparation; univariate analysis; multivariate analysis; model estimation and testing; calculation of the performance statistics; and documentation.
The first waterfall phase is perhaps the most important. Modelers should know what the requirements are for the model from at least three perspectives: (1) the model owner; (2) the users of the model and; (3) the regulator. The careful planning and sequential approach of the waterfall methodology are meant to ensure that the end-product fully complies with both user requirements and any applicable regulation.
Agile approaches, in contrast, are more adaptable, flexible and responsive to changing market conditions. Consequently, while they are not yet widespread, they are gaining in popularity.
It is logical to deploy agile approaches in customer service units first, so that they can benefit from appropriate responses to evolving customer requirements. However, if a bank has the ambition to become an agile organization, the rollout should be expanded to other units, like IT services and even model development. But what does this entail?
Breaking Down the Agile Approach for Model Development
Let’s now examine what an agile approach for model development would look like. The crucial element of such an approach is that modelers start to experiment with model estimations early in the development process. Essentially, this means that it is not necessary to complete an entire data collection phase before fitting a default indicator to risk drivers for, say, a PD modeling project.
One starts with the first data set and, after a quick and preferably automated data cleansing process, the first model fit is then made. This process completes a first sprint, also known as a two-week iteration.
Agile model development, as a whole, is inspired by an overarching narrative that different user groups deserve customized solutions. According to the agile philosophy, to address each group’s needs explicitly, the user stories should be written from the point of view of a specific user.
Let’s consider a model development example where, say, a second-line stakeholder needs a PD model that has a proper discriminatory power – i.e., an area-under-the-curve (AUC) of at least 75%. This user story would be linked to tasks via a “backlog,” from which the development team would identify a workload for the upcoming sprint. For model development projects, a sprint duration of two weeks seems to be a good choice.
Marco Folpmers
At the beginning of each sprint, the backlog is reviewed. Priorities can change from sprint to sprint, since the agile approach is adaptable to new information that needs to be picked up by the development team. If, for example, the bank has just received a new letter from a supervisor with additional requirements for the PD model, this can be seamlessly integrated into the backlog.
At the end of the sprint, it is important to identify the tasks, or groups of tasks, that have been completed, so that these can be removed from the backlog and the work on new tasks can be planned. To avoid discussion on whether a component of the newly developed PD model qualifies as completed, it is necessary to have a proper definition of “finished” in place upfront.
Common Pitfalls
Every credit risk model must not only meet user requirements but also comply with regulation. However, this dual objective is not easy to achieve. This is one of the reasons why the agile approach to model development has not yet been widely adopted, even though many modeling teams understand its advantages.
What are the pitfalls of this alternative methodology? Well, since there is typically no set delivery data cited at the start of agile modeling projects, they may not live up to expectations. The backlog of tasks is adaptable and priorities are constantly in flux. What’s more, it is not even certain whether a finalized model can be submitted to the validation unit after the project is finished.
To avoid failures, it is necessary to anticipate common agile pitfalls for model development. The most important pitfalls can be avoided if one adheres to the following guidelines:
An agile project needs an agile organization. The model development project needs to be embedded in an overall culture that is supportive of experimentation and prototyping, while allowing for adaptable short-term goals, quick sprints and on-the-go reprioritization.
The risk of misestimation is acceptable. When models are first estimated using an agile approach, important risk driver data is lacking, and each project therefore starts with a misestimation. In such cases, the coefficients and related statistics are also unreliable. The first estimation may only be useful to get acquainted with the data and to gain a general idea about how well the initial risk drivers work. As more data comes in, the risk of misestimation will gradually decrease.
Training is needed. One cannot expect the modeling team to switch from waterfall to agile overnight. Training in agile project methodology is required.
There must be a clear definition of “done.” The big risk when executing agile projects is that activities on the backlog are not effectively completed, so that they are transferred to the next sprint. This risk is mitigated by having a good understanding of the conditions when a single task or a group of tasks can be marked as done. When this is clearly defined, one can make a better estimate of the number of hours it will take to complete the tasks and – after the result is submitted – to assess whether they are really completed.
Oversight is critical. Unlike the Prince2 project approach in waterfall planning, neither a project initiation document nor a steering committee needs to be set up using the agile approach. But project oversight is still crucial for success, and must be managed by the product owner. He or she should be mandated by the bank to make decisions regarding the project.
Is Agile Riskier?
Projects managed through waterfall-driven models are generally less risky than agile projects. After all, waterfall projects have a lengthy project plan, tollgates and a steering committee to manage exceptions.
But if agile is properly applied, can it actually be the safer option? Possibly.
Let’s consider an example where we have two teams working on a PD model development project. One team applies agile, the other a waterfall approach. As time progresses, the agile team receives the first dataset and develops its initial model, automatically cleansing the data at the start.
During follow-up sprints, let’s also say that the input data is gradually improved and a proper perimeter is established, so that the definition of the model development scope complies with the model application scope. By renewing the data and running new estimation procedures on the enhanced data set, the AUC is gradually increasing (via bi-weekly sprints) toward 80%.
Figure 1: An Example of AUC Development for the Waterfall and Agile Teams
How different this looks in comparison to a model developed by the waterfall team! Keep in mind, before moving on to any other steps, the waterfall team must first write an elaborate project initiation document. Moreover, it needs more time to receive a complete modeling dataset, and a full univariate analysis must be run – identifying and removing outliers and assessing proper risk drivers – before the model is estimated.
During this set-up period, there is no AUC. It’s only after the proper modeling phase is entered – following passage through the required tollgates – that the first AUC appears using the waterfall approach. (Eventually, as we see in Figure 1, that will be optimized during a very limited number of model generations.)
The main message is that, although a waterfall process with tollgates looks more robust, it is placing a heavy bet on “being right the first time,” rather than improving as model development evolves. An agile approach, in contrast, quickly works toward an initial model estimation that is iteratively improved.
Parting Thoughts
While the agile approach offers an attractive alternative to the waterfall approach, it is, of course, no panacea.
Problems that exist with the waterfall approach do not magically disappear when using the agile methodology. Indeed, using either approach, model development can be derailed by bad data quality or insufficient project management skills.
However, when the proper steps are followed, the agile approach to model development is an effective alternative for financial institutions that need credit risk models that are more adaptable and responsive to changing market conditions.
Dr. Marco Folpmers (FRM) is a partner for Financial Risk Management at Deloitte the Netherlands.
Topics: Modeling