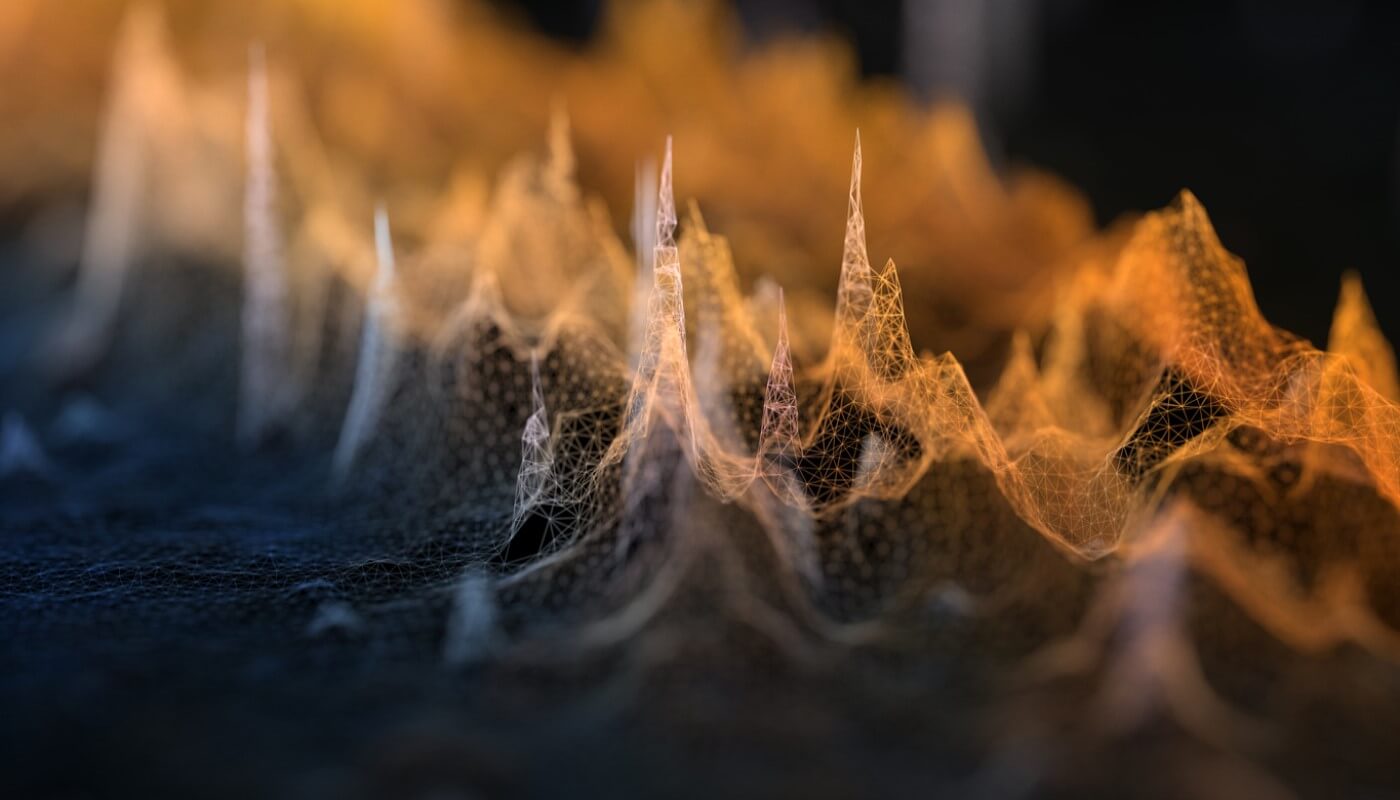
In an ideal world, we would develop CRE credit risk models using a complete data set representing loans from commercial banks, insurance companies, asset managers and CMBS. However, CRE loan-level default data from banks and insurance companies are generally not publicly available, and there is no third-party vendor that provides such information in Europe.
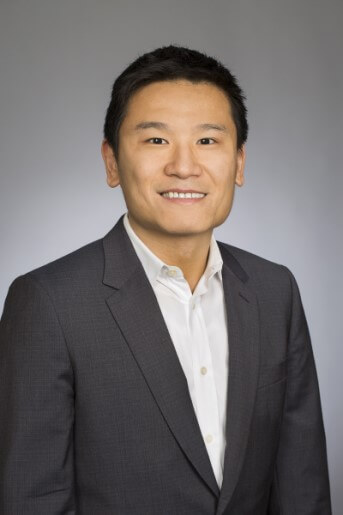
To date, CMBS remains the primary loan-level data source, having been well-tracked and maintained since its inception. While the European CMBS market has not been active since the financial crisis, its observable history is long enough to cover originations, defaults and distinct credit cycles, all of which are essential for developing a reliable credit risk model.
However, CMBS loans tend to differ in composition from portfolio loans, and the explosion of CRE lending among non-bank lenders (who often have unique portfolios) adds further complication to the calibration of models.
Specific guidance on these issues is sparse, but the development of our own internal CRE Europe modeling framework - built upon extensive research on public data sets and supplemented by our own internal loan-level data - has yielded several lessons (and one surprising finding) that should be instructive to risk managers in Europe as they consider their own CRE models.
No Two Borrowers Are Alike
Why would a commercial real estate loan borrower default on their debt obligations? Inadequate cash flow from the property to cover the scheduled mortgage payment is a primary and straightforward cause. Another is devaluation, where the underlying commercial properties that serve as the secured collateral are worth less than the mortgages.
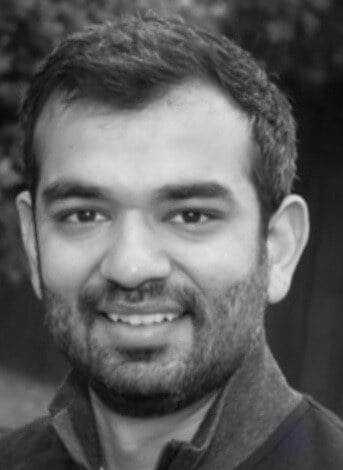
However, a borrower's decision to default is not purely a financial matter, and this is a challenge that purely statistical modeling struggles to overcome. The choice to default - especially in the case of illiquid or hard-to-value CRE assets - is always influenced by unique subjective assessments.
At the borrower level, these factors may make default a reasonable and rational choice, but observation at the aggregate level conceals these idiosyncrasies and makes these decisions appear “sub-optimal.” It is therefore crucial to observe and to record loan, property and subjective borrower-specific factors.
How should these factors be incorporated into models? One way is through a qualitative overlay in which lenders can rate these additional risk factors on a “scorecard.”
The final risk measure is a weighted combination of the quantitative model output and qualitative score, which enables financial institutions to improve how they measure and manage CRE credit risk through a more comprehensive perspective.
Know Your Measurements
Theoretical thinking is useful as a starting point in disentangling the causes and consequences from a rational economic-reasoning perspective. However, a model will not be accurate and useful for business applications if it is simply an intellectual exercise without the benefit of actual, observed historical data.
Our research shows following empirical measures are critical to default modelling:
- Debt Service Coverage Ratio (DSCR). This is the ratio between net operating income (NOI) of the CRE collateral and the scheduled debt service payment for the borrower. A higher DSCR indicates better ability of the borrower to meet the debt obligation, and therefore has a lower probability of default.
- Loan-to-Value (LTV). This is the ratio between outstanding loan balance and the value of the CRE collateral. An increasing LTV leads to a growing incentive for the borrower to walk away from the property and default on the loan.
- Market Vacancy. This factor captures the health of the local CRE space market environment. Given the same loan-level characteristics, default risk tends to be higher in markets with higher-than-average vacancy.
- Market Price Change. This factor captures the health of the local CRE asset-market environment. Given the same loan-level characteristics, default risk tends to be lower in markets that experience stronger-than-average price growth.
- Loan Seasoning. CRE loans typically exhibit a hump-shaped seasoning pattern, with default risk peaking in the fourth to eighth year.
- Property Type. Given the short-term nature of rentals, hotel and other non-core property types tend to have higher operating costs and greater default risk. By contrast, property cash flows tend to be more stable for core property types (including office, retail, industrial and residential), because space is usually leased out on a multi-year basis.
Map Empirical Measures to Systematic Factors
The integration of CRE market systematic factors is an important enhancement in providing the best estimates of the point-in-time probability of default (PD) levels. For example, one can use the market vacancy rate as a proxy for the current condition of the space market, and the market price change as a proxy for CRE asset-market conditions.
This process leads to a comprehensive credit risk assessment approach that combines market-based (“systematic”) risk factors and loan-specific (“idiosyncratic”) risk factors that drive the vast majority of credit problems in the commercial mortgage industry. Without these systematic factors, while the model can still discriminate very well between defaults and non-defaults, it would underestimate PD during market downturns and overestimate PD during market upturns.
Everything Old is New Again
During the development of our model, one surprising finding was that after controlling for common risk factors such as DSCR, LTV, market and property type, commercial mortgages all behave similarly, whether the loans were originated by banks, insurance companies or CMBS. As it turns out, because the borrowers ultimately operate the assets, they do so to optimize their own financial interests, regardless of lender type.
The upshot is that despite the changing CRE landscape in Europe, a commercial property's financials - visible through its DSCR and LTV - continue to play a dominant role in mortgage defaults and losses. This little-changed relationship between a loan's financial performance and its credit risk makes a quantitative model not only possible, but vital, as heightened regulatory scrutiny around commercial mortgage lending will persist in at least the near term.
Eric Bao and Sumit Grover both work at Moody's Analytics. Bao is the director of commercial real estate research, while Grover is the director of product management.
Article Updated: March 7, 2022