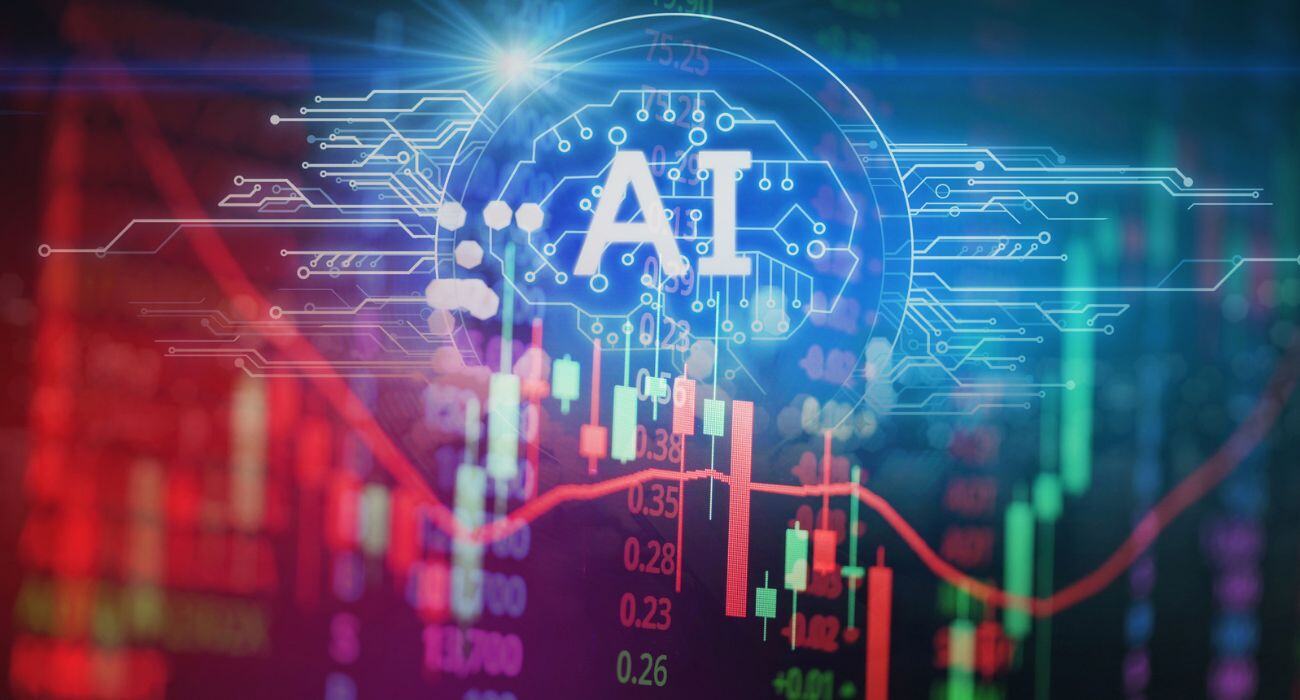
Last month, the meteoric success of the DeepSeek R1 artificial intelligence engine grabbed worldwide headlines. What were the key risk management takeaways of this markets-altering event, and what did it tell us about the importance and difficulty of technology stress testing?
The prospect of cheap, open-source AI not only triggered disruption in the entire technology sector but also had historic short-term financial ramifications – moving $1.3 trillion of market capitalization from India to China and causing by far the largest one-day decline in market capitalization ($593 billion) for a stock.
Aaron Brown
Some of the predictions about the impact of DeepSeek’s shockingly fast ascension – e.g., that it would cause China’s yuan to replace the U.S. dollar as the global reserve currency, or that it represented a triumph of authoritarianism over liberal democracy – were undoubtedly over the top. But there’s no question this was a major technology risk event. Moreover, to my knowledge, it was not one captured in anyone’s stress tests or scenario analyses.
Of course, I would not expect risk managers even in the AI sector to have run specific stress tests on DeepSeek achieving spectacular success. But the idea that a semi-outsider on a modest budget would achieve a significant breakthrough in AI commercialization was certainly plausible. After all, “two guys in a garage” disrupting everything has been reasonably common in recent decades.
The risk managers I have spoken to all tell me that DeepSeek wasn’t included in risk analyses because they underestimated the financial and political impact such a breakthrough would cause. All of them have devoted serious thought to AI risk, but tended to assume it would take big AI events to cause big changes outside of the sector.
Thinking Beyond the Extremes
This led me to start considering other recent examples of blind spots caused by focusing on extremes. People have devoted a lot of thought, for instance, to globally catastrophic asteroid impacts with estimated probabilities in the range of 0.001% to 0.0001% — but not so much to 2% probabilities of massive local asteroid disasters.
“City-killer” asteroid 2024 YR4, estimated in early February to have a 1-in-43 chance of hitting the Earth on December 22, 2032, is one potential “local” disaster that is outside of the extremes. The odds of this asteroid colliding with Earth fluctuate and recently dipped significantly — but, at an estimated 196-foot diameter, it could flatten an area of 1,000 square miles or more and have a major impact on global weather. Indeed, if it hit a populated area, it could rank among the greatest natural disasters in human history.
When we talk about risk managers perhaps focusing too much on the extremes, global pandemics and climate change are two other issues that come to mind.
Even before COVID-19 in 2020, pandemics had long been a major risk concern. But how many people expected supermarket egg shelves to empty due to Avian flu?
Similarly, a lot of ink has been spilled over the catastrophic risk of climate change, but much of it reads like Hollywood disaster movie plots. In fact, the two most expensive natural disasters in U.S. history – 2024’s Hurricane Helene and 2025’s Los Angeles wildfires – were at least partially related to climate. In one sense the events were ordinary: hurricanes and wildfires are common and will recur often in the future. Nevertheless, they had historically extreme impacts.
The point here is that risk managers should not spend all their time thinking about the worst-case scenarios, whether they are pondering economic meltdowns, natural disasters or technology shocks. There are smaller, more localized, more likely events that can also have a huge impact.
VaR: An Imperfect Solution
To understand our obsession with the extreme, let’s take a step back to consider the risk management paradigm developed in the late 1980s and early 1990s. Most risks can be quantified using theory and historical data, which allow precise optimization of risk decisions. But the stock market crash of October 19, 1987, convinced many people, including me, that long-term outcomes were dominated by low-probability, high-impact events that defied calculation.
The solution that emerged in the wake of Black Monday, after a few years of research and debate, was Value-at-Risk (VaR). Using a standard 95%, 10-day VaR meant you expected one VaR-break (one loss larger than the VaR) every 10 months or so. Within the VaR limit, you could predict consequences with reasonable confidence, and you could assume line risk takers had enough experience to make sound decisions.
However, outside of VaR (i.e., events that fell beyond the 95% confidence interval), all bets were off. Market prices might not exist. You might not know your positions and exposures, nor be able to manage them. People in authority might be unavailable, information systems might be down or wrong, and legalities could be unclear. Counterparties and institutions, moreover, might fail, and fundamental economic relationships might not hold. Events could cascade in positive-feedback spirals, and governments might change rules in response to them. You may not even be able to define losses or probabilities.
To cover the full range of risks — not just the ones with reliable historical statistics — we developed tools like stress tests and scenario analyses. These were “plausible extreme” situations used to make contingency plans, clarify responsibilities and authorities, and communicate risk strategy to stakeholders. They had to be extreme to capture effects not found in everyday events, but also plausible, so you could guess what choices might be available and what the consequences would be.
To calibrate “plausible extreme,” we often started with rules of thumb like three-to-10-times VaR. Additionally, we looked to longer-term and more general history than was used for VaR estimation. For example, we might use three years of S&P 500 daily returns and high/low prices for a one-day, 99% S&P 500 VaR — but also look back to historic S&P 500 crashes in 1929, 1987 and 2008, as well as financial crashes in markets other than stocks.
Preparing for Plausible Extremes
The important point is no one expects the future to correspond to any one stress test or scenario analysis, or even a combination. The hope, though, is that you can navigate whatever actually happens by preparing for some plausible extreme conditions you can foresee.
Preparing for evacuating a building in a fire, for example, is useful for bomb threats, earthquakes, toxic spills and many other possible disasters. Similarly, preparing for a 50%, 10-day stock market crash forces risk managers to think about systems and procedures important in other large changes in market prices.
This paradigm was initially developed for market risk, for which it is relatively straightforward. Publishing a VaR every day before start of business — one with the correct number of breaks, no more and no less, with the breaks uncorrelated in time or with the level of VaR — is neither difficult conceptually nor hard to evaluate.
Daily VaR calculations forced system improvements costing literally billions of dollars in large global financial institutions, so they were, and remain, expensive. However, once you have a history of VaR, choosing market moves three-to-10 times as large is easy.
It took some modifications to apply this "VaR plus stress tests" approach to credit risk (first) and then to operational risk, but it seems less suited for technology risk. In 1990 we thought technology was changing rapidly – but in the short and medium term, it seemed less important than market, credit and operational risk.
Today, however, technological change could be the largest medium-term risk for many entities, and a significant short-term risk for all entities. Technology stocks drive a substantial part of stock market volatility, and technology shocks disrupt business and finance at least as much as any other type of shocks.
Unfortunately, it’s hard to know how to calibrate technology stress tests and scenario analyses. We don’t have daily mark-to-market numbers to compute precise VaRs, which we could multiply by three or 10. Indeed, it’s not even clear what it would mean to multiply by three or 10. Historical technology shocks are so different from plausible future ones that they’re of little use.
The Power of Reverse Stress Testing
One technique I have found useful is the reverse stress test. This starts with a disaster scenario, which can be implausibly large — implausibly large ones, in fact, work best. You then get a group of decision-makers to discuss the most plausible path to get to it.
Reverse stress testing works best when you engage a diverse group of people knowledgeable about different aspects of the organization — IT, finance, legal, operations, etc. The process is most effective when you start with lower-level employees, because they often are aware of things that senior people are not. (Ultimately, this knowledge should improve your senior-level discussions.)
After you’ve done several of these group discussions about different disaster scenarios, you’ll begin to find bottlenecks that many different paths go through — turning points where ordinary events accumulate to create dangerous situations.
Of course, this is only a starting point to creating useful stress tests. You’ll also want to discuss the bottleneck scenarios with subject matter experts on the technology team to estimate how plausible and how extreme they are, as well as to find some historical parallels to flesh out the descriptions.
Risk management cannot be done staring at a computer screen or annoying everyone with petty technical details. And one important side benefit of the reverse stress testing process is that it will encourage your staff to talk to lots of different people about interesting things.
What’s more, the exercise can help participants in these sessions think more broadly about major risks — and, importantly, to consider the full path from the everyday world to the Hollywood disaster movie. (Since risk is two-sided, it’s key to collect information about opportunities, as well as dangers.).
Certainly, there are other ways beyond reverse stress testing to assess and manage technology risk. But recall DeepSeek and some of the other examples I gave earlier to make sure that you’re not thinking too small or too large in your stresses.
Aaron Brown worked on Wall Street since the early 1980s as a trader, portfolio manager, head of mortgage securities and risk manager for several global financial institutions. Most recently he served for 10 years as chief risk officer of the large hedge fund AQR Capital Management. He was named the 2011 GARP Risk Manager of the Year. His books on risk management include The Poker Face of Wall Street, Red-Blooded Risk, Financial Risk Management for Dummies and A World of Chance (with Reuven and Gabriel Brenner). He currently teaches finance and mathematics as an adjunct and writes columns for Bloomberg.
Topics: Risks & Risk Factors