Risk Insights
Your dedicated resource for the latest risk articles, podcasts, webcasts, white papers, and more.
Financial Risk
Financial Risk
Financial Risk
Latest in Financial Risk
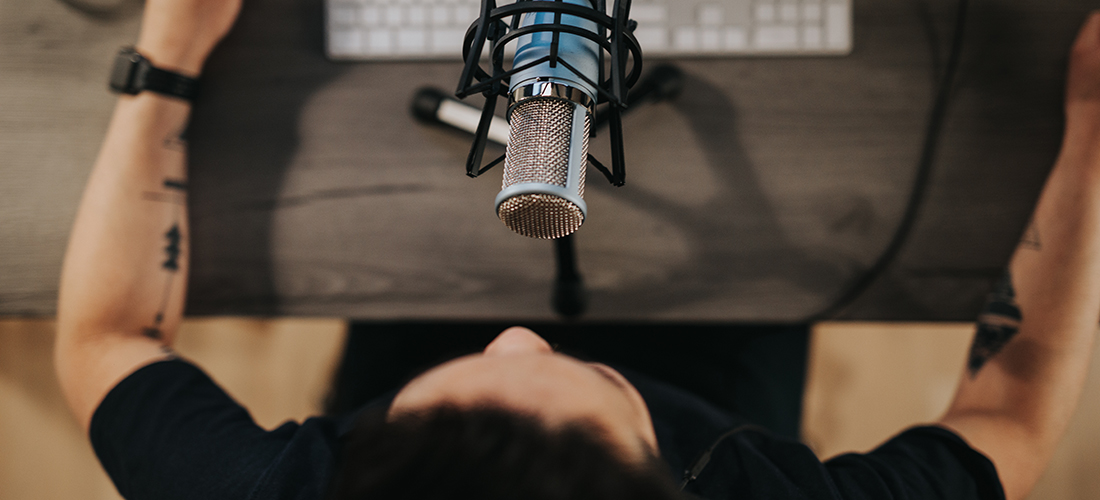
Stress Testing: Current Issues, Regulatory Analysis, and a Sneak Peek at the Fut
July 10
Hear from Cristian deRitis, deputy chief economist at Moody’s Analytic...
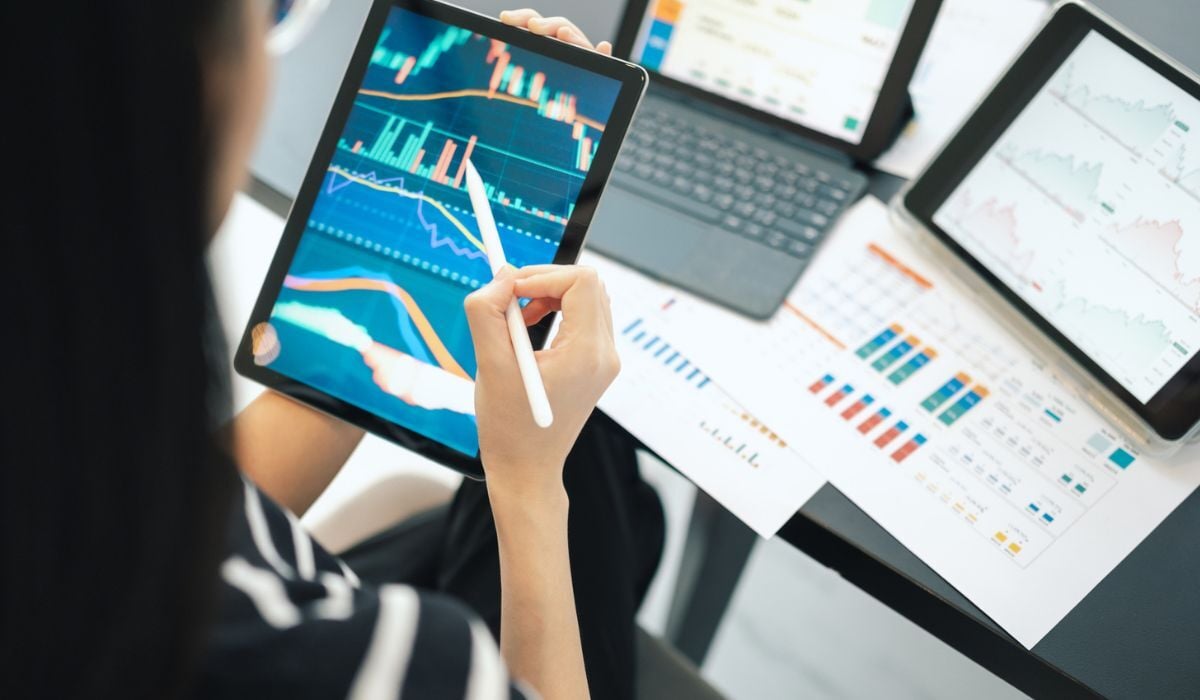
Rethinking Capital Management: Advice for Banks in a Time of Volatility and Uncertainty
July 11
Now that the Basel III finalization rules are being implemented and en...
Latest in Sustainability and Climate
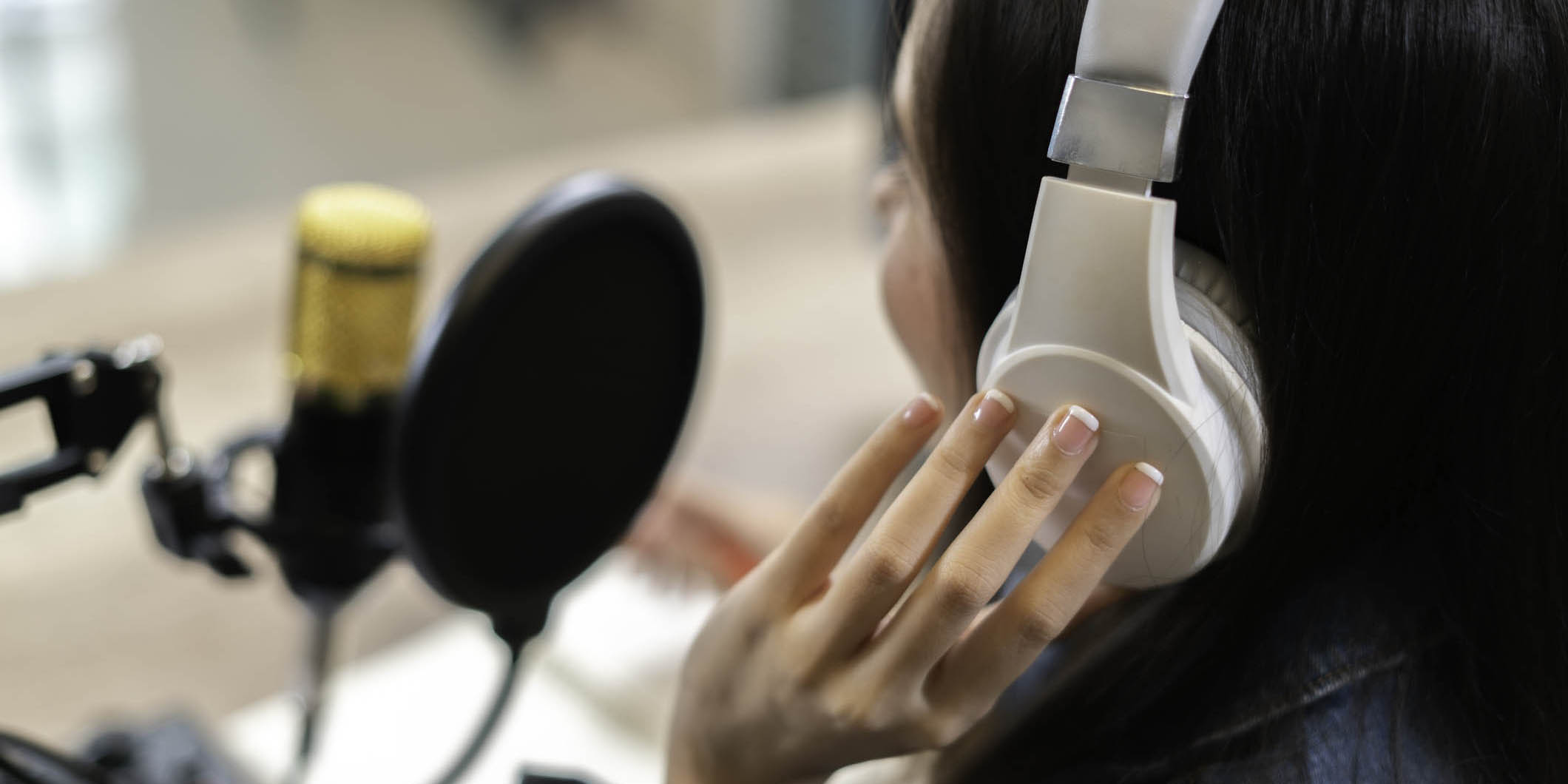
Greening the Games: How Paris 2024 Delivered on Climate Ambition
July 10
Hear from Georgina Grenon, Director of Environmental Excellence for th...
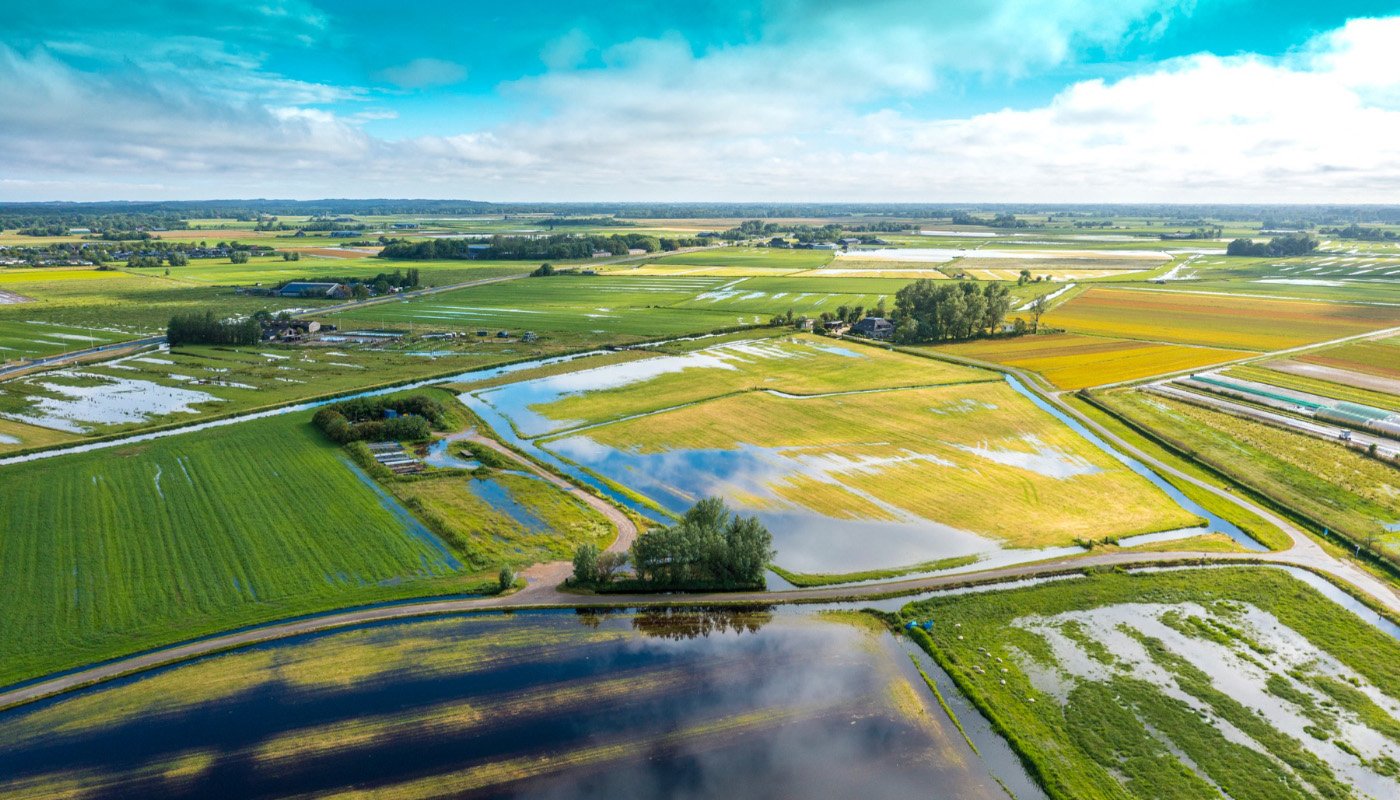
Understanding the Physical Risks Associated with Climate Change
July 3
How do financial risks arise from the physical impacts created by a ch...
Latest in Risk and Artificial Intelligence
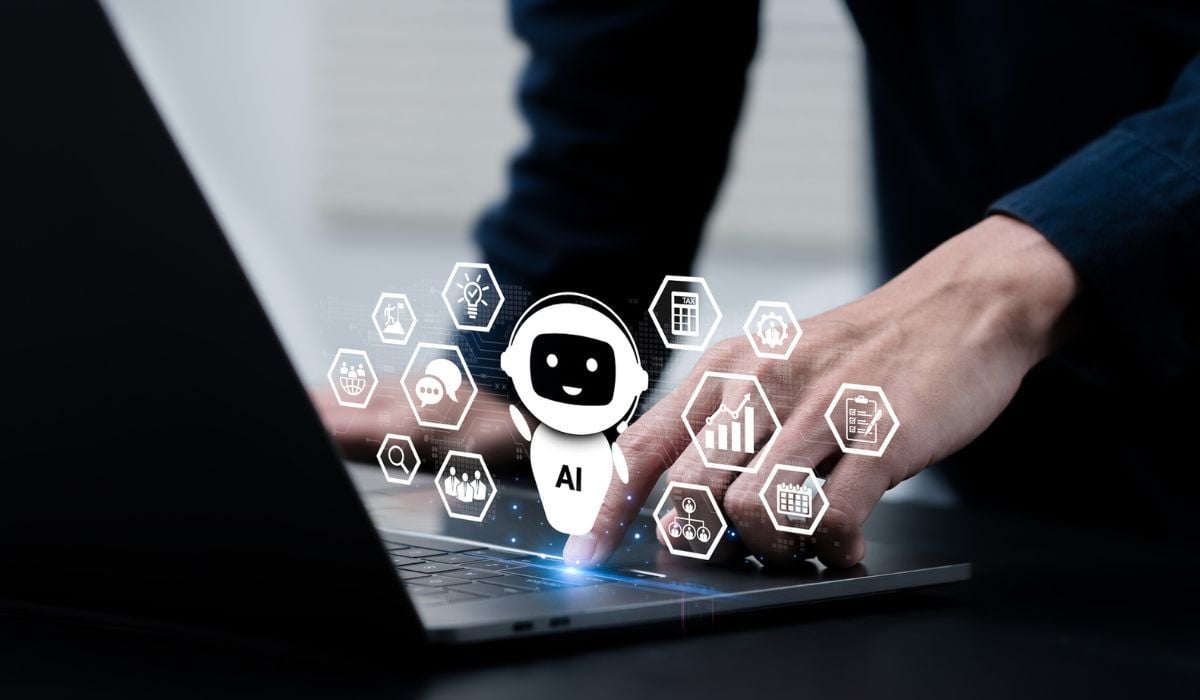
Agentic AI: On the Frontier of Autonomy
June 13
The capabilities build on, and go beyond, those of generative AI. The ...
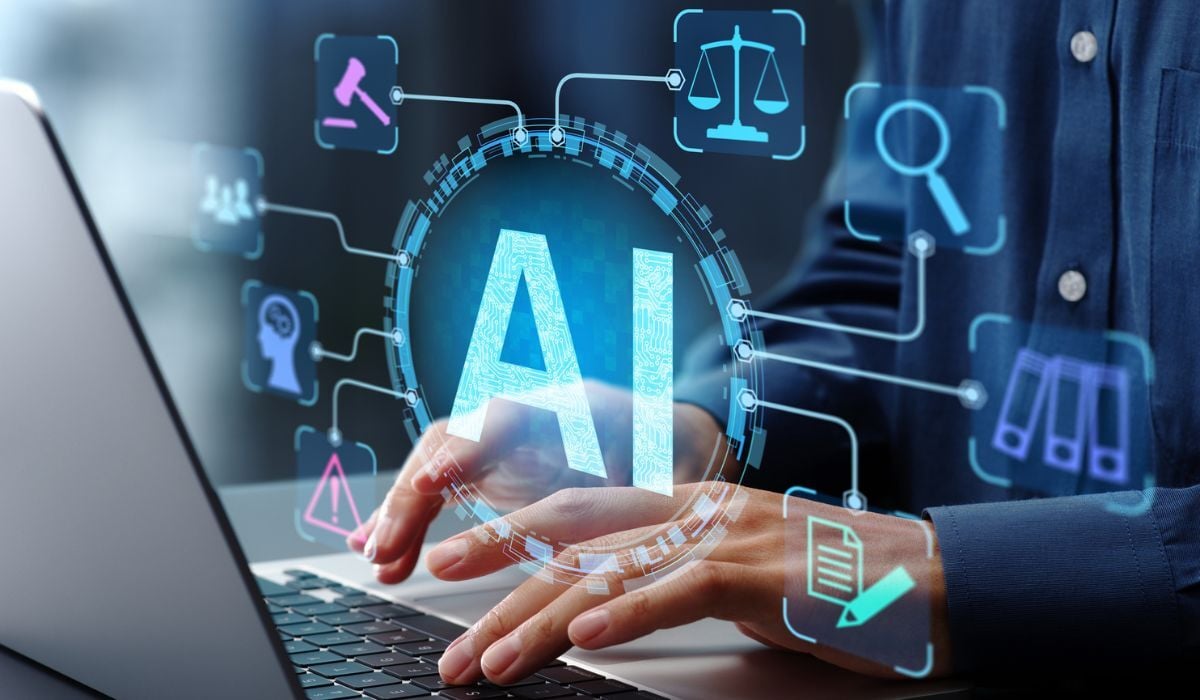
The EU AI Act: A Risk Manager's Roadmap
June 6
New regulation in Europe should motivate financial risk institutions a...
Events
Chapter Meeting
ESG Risk Assessment and the Importance of Data Quality
February 13, 2025 | 6:00 PM
In-Person | Eurizon Capital SGR, Via Melchiorre Gioia, 22, Milan,
Featured Columns
Rethinking Capital Management: Advice for Banks in a Time of Volatility and Uncertainty
By Marco Folpmers | July 11, 2025
Read Article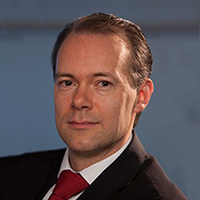
Know Your Customer: Who’s Short Technology Venture Options?
By Aaron Brown | June 27, 2025
Read Article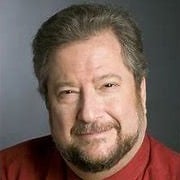
ERM: Risk Identification and Assessment
By Brenda Boultwood and Brendan Schroeder | June 20, 2025
Read ArticleGet Additional Risk Insights
Want more on financial risk, AI, and sustainability and climate? Searching for thought leadership on a topic you don't see here? Explore our Risk Insights Resource Center now.