Risk Insights
Your dedicated resource for the latest risk articles, podcasts, webcasts, white papers, and more.
Financial Risk
Financial Risk
Financial Risk
Latest in Financial Risk
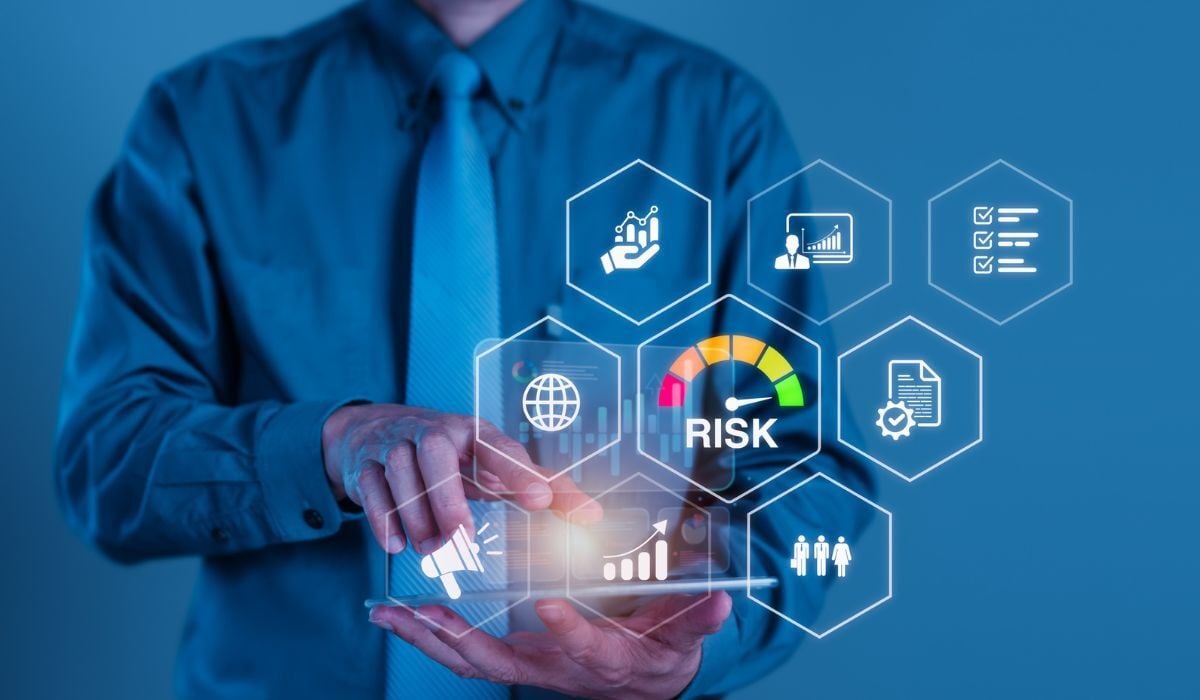
“Curiosity and Challenge” Fueled the Career Journey of Santander’s Ashwani Aggarwal
July 18
Educated in mechanical engineering, the bank’s U.S. CRO had his financ...
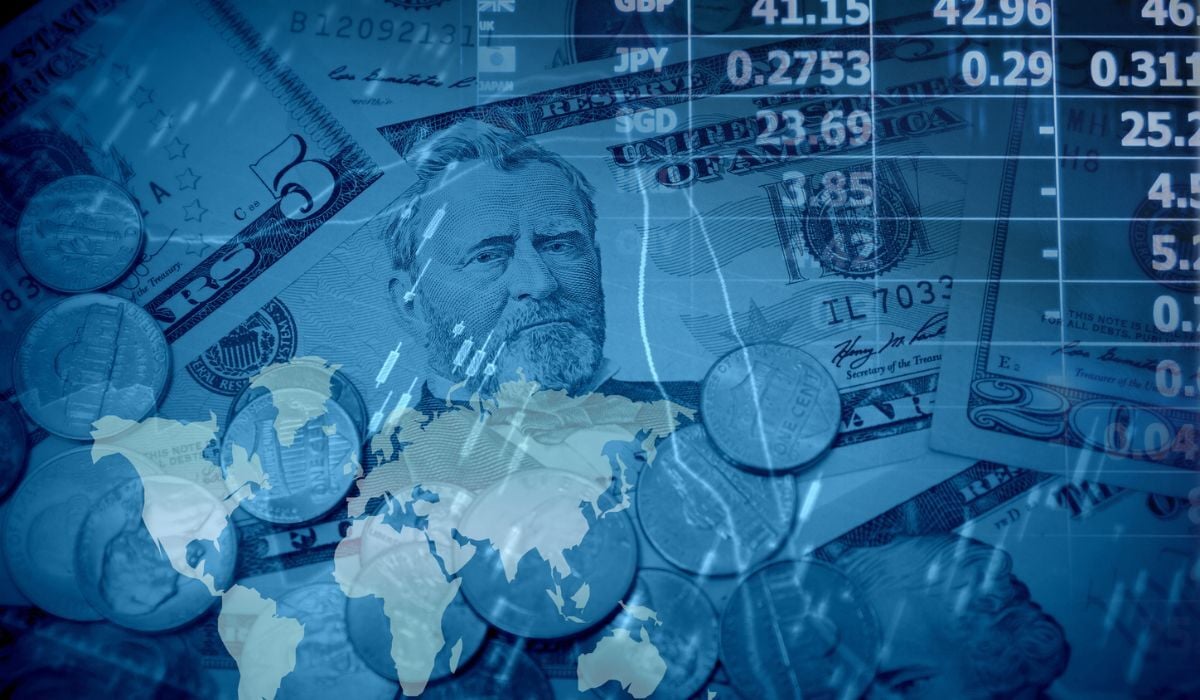
Should Banks Be Concerned About Geopolitical Risk, and Can They Actually Do Anything About It?
July 18
Geopolitical dangers like wars and fluctuating tariffs undoubtedly hav...
Latest in Sustainability and Climate
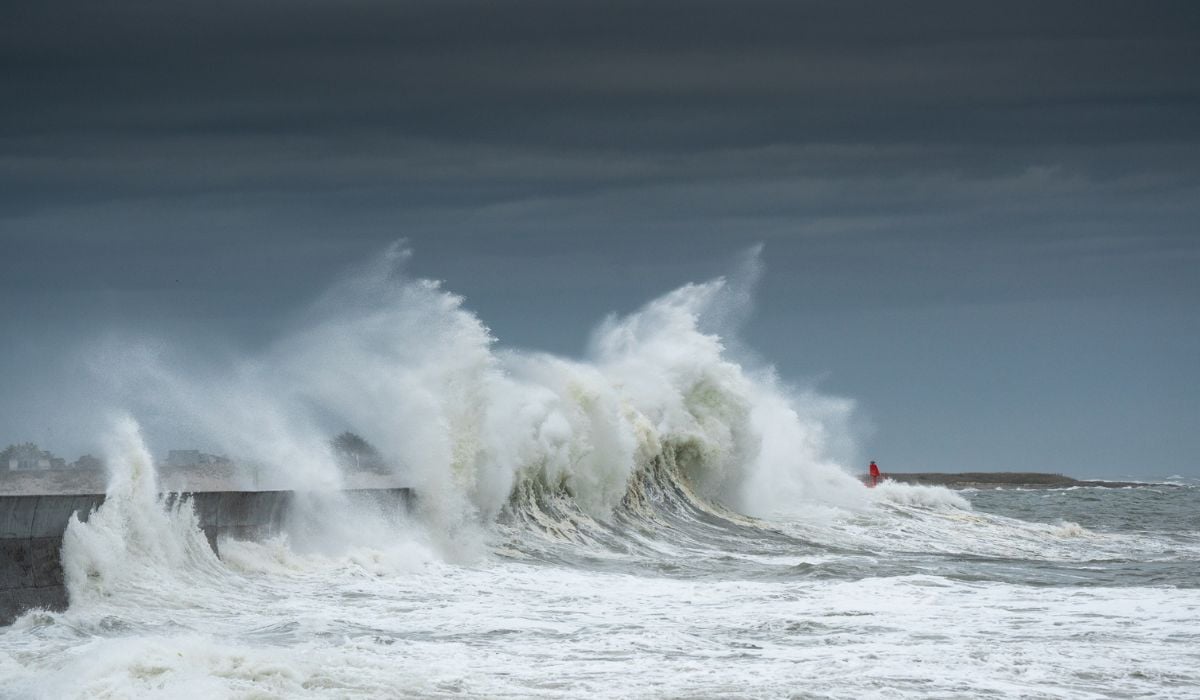
Valuing Adaptation: A Call to Understand What Matters
July 17
Increasing physical risks from climate change require greater efforts ...
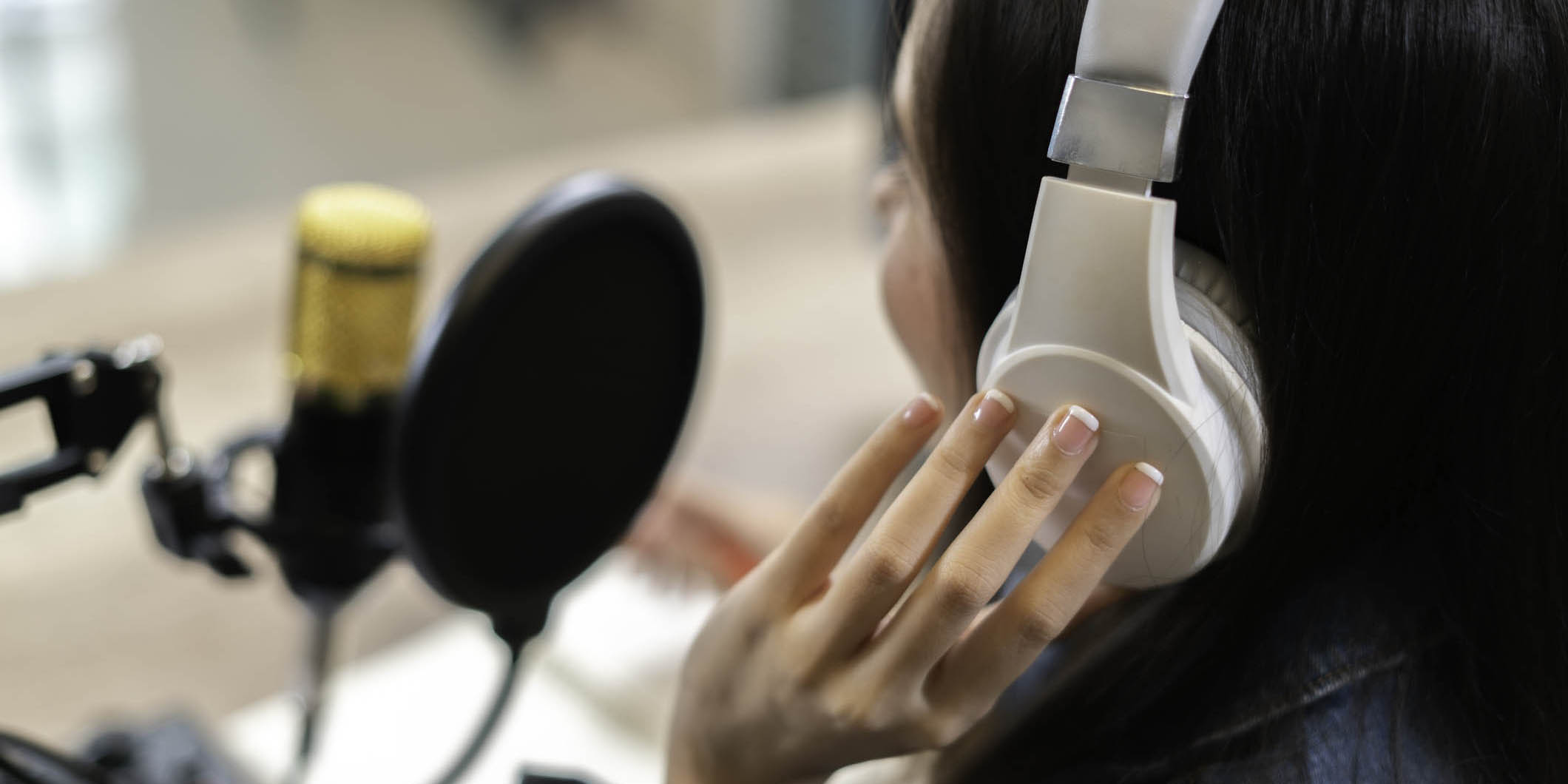
Greening the Games: How Paris 2024 Delivered on Climate Ambition
July 10
Hear from Georgina Grenon, Director of Environmental Excellence for th...
Latest in Risk and Artificial Intelligence
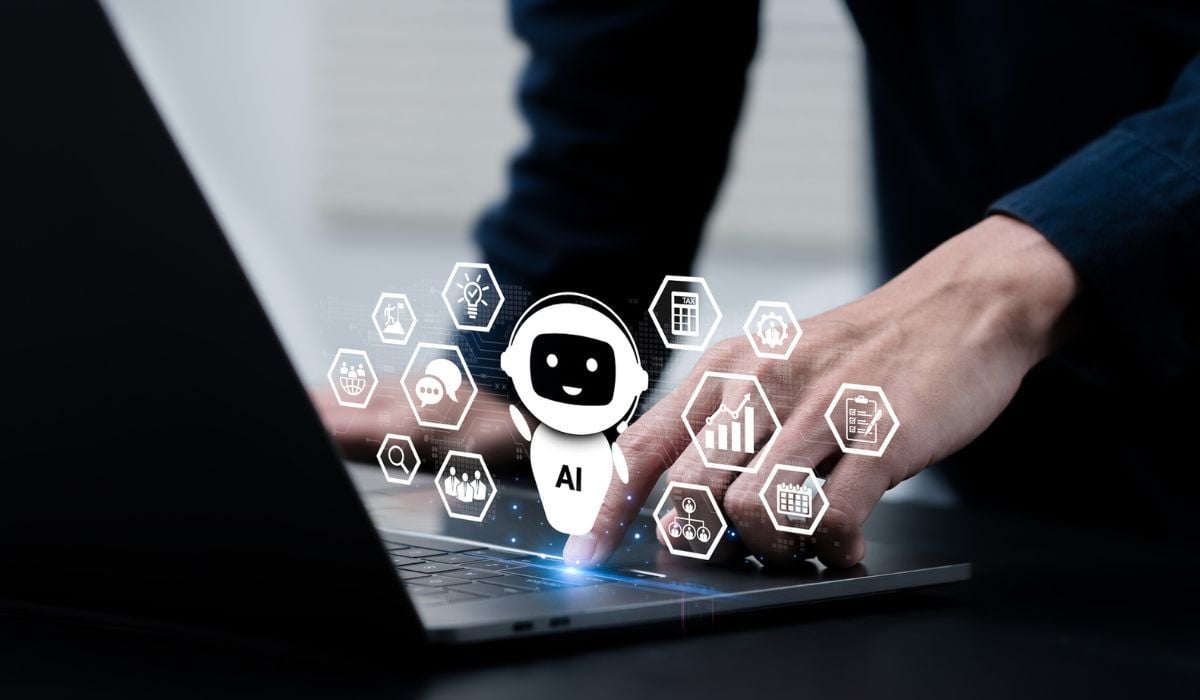
Agentic AI: On the Frontier of Autonomy
June 13
The capabilities build on, and go beyond, those of generative AI. The ...
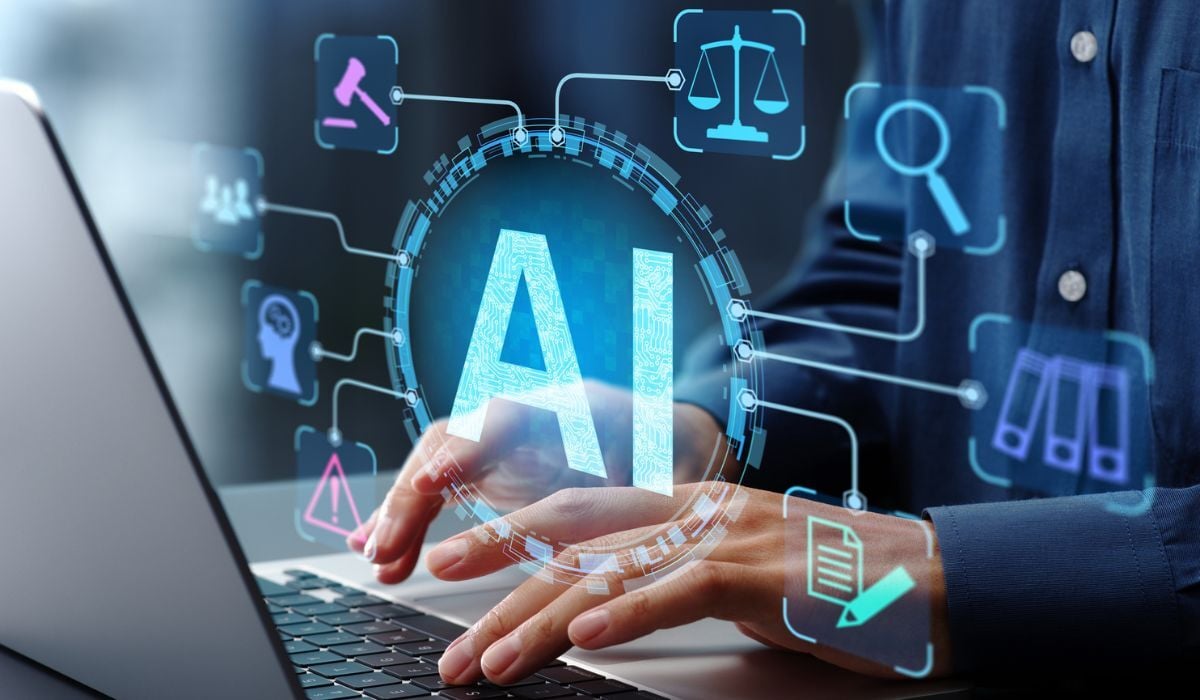
The EU AI Act: A Risk Manager's Roadmap
June 6
New regulation in Europe should motivate financial risk institutions a...
Events
Chapter Meeting
ESG Risk Assessment and the Importance of Data Quality
February 13, 2025 | 6:00 PM
In-Person | Eurizon Capital SGR, Via Melchiorre Gioia, 22, Milan,
Featured Columns
Should Banks Be Concerned About Geopolitical Risk, and Can They Actually Do Anything About It?
By Tony Hughes | July 18, 2025
Read Article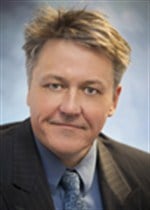
Rethinking Capital Management: Advice for Banks in a Time of Volatility and Uncertainty
By Marco Folpmers | July 11, 2025
Read Article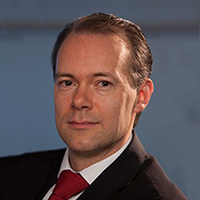
Know Your Customer: Who’s Short Technology Venture Options?
By Aaron Brown | June 27, 2025
Read Article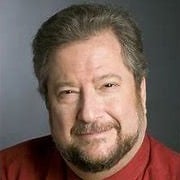
ERM: Risk Identification and Assessment
By Brenda Boultwood and Brendan Schroeder | June 20, 2025
Read ArticleGet Additional Risk Insights
Want more on financial risk, AI, and sustainability and climate? Searching for thought leadership on a topic you don't see here? Explore our Risk Insights Resource Center now.